CV-SincNet: Learning Complex Sinc Filters From Raw Radar Data for Computationally Efficient Human Motion Recognition
IEEE Transactions on Radar Systems(2023)
摘要
The utilization of radio-frequency (RF) sensing in cyber-physical human systems, such as human-computer interfaces or smart environments, is an emerging application that requires real-time human motion recognition. However, current state-of-the-art radar-based recognition techniques rely on computing various RF data representations, such as range-Doppler or range-Angle maps, micro-Doppler signatures, or higher dimensional representations, which have great computational complexity. Consequently, classification of raw radar data has garnered increasing interest, while remaining limited in the accuracy that can be attained for recognition of even simple gross motor activities. To help address this challenge, this paper proposes a more interpretable complex-valued neural network design. Complex sinc filters are designed to learn frequency-based relationships directly from the complex raw radar data in the initial layer of the proposed model. The complex-valued sinc layer consists of windowed band-pass filters that learn the center frequency and bandwidth of each filter. A challenging RF dataset consisting of 100 words from American Sign Language (ASL) is selected to verify the model. About 40% improvement in classification accuracy was achieved over the application of a 1D CNN on raw RF data, while 8% improvement was achieved compared to real-valued SincNet. Our proposed approach achieved a 4% improvement in accuracy over that attained with a 2D CNN applied to micro-Doppler spectrograms, while also reducing the overall computational latency by 71%.
更多查看译文
关键词
Radar,RF sensing,FMCW,micro-Doppler signature,CV-SincNet,human activity recognition,ASL
AI 理解论文
溯源树
样例
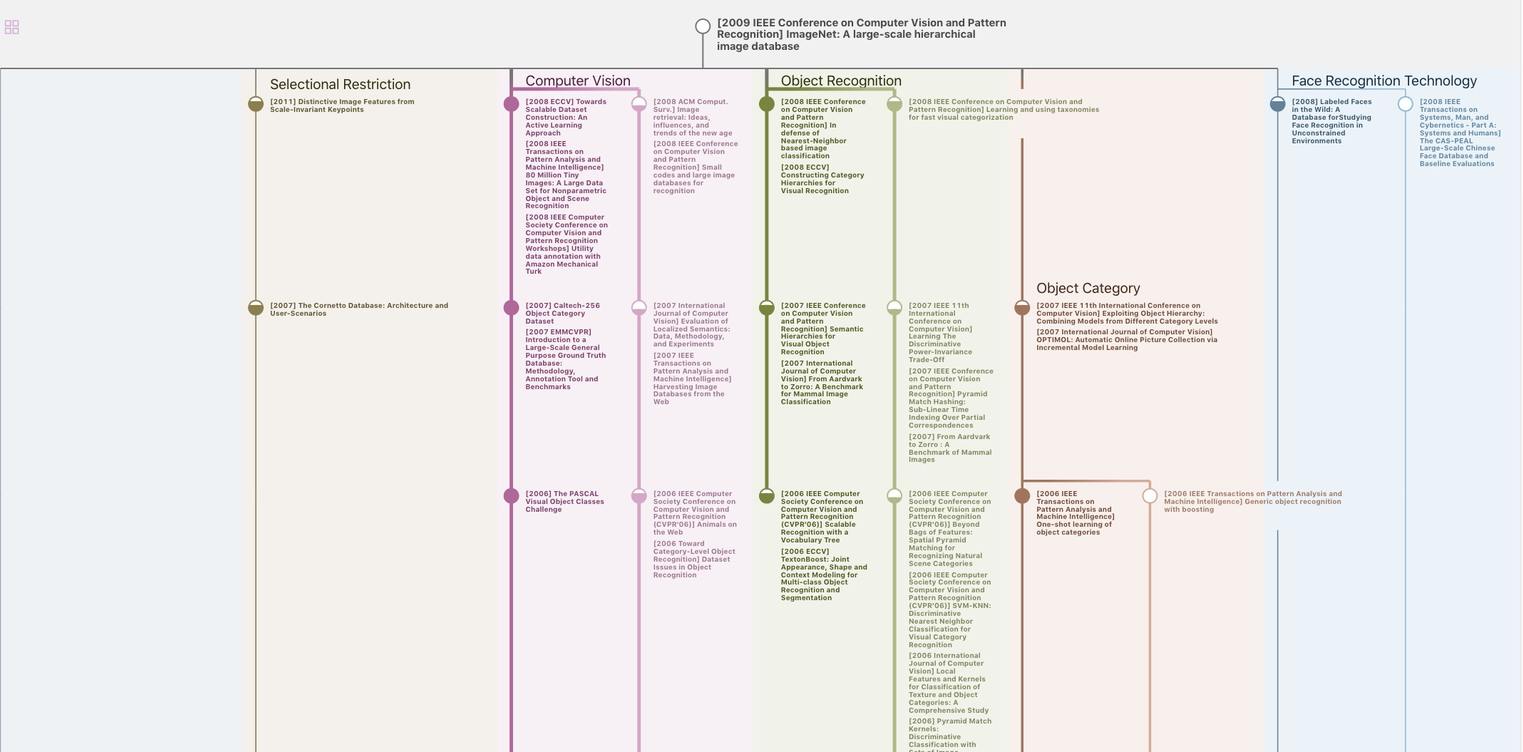
生成溯源树,研究论文发展脉络
Chat Paper
正在生成论文摘要