HAIMA: A Hybrid SRAM and DRAM Accelerator-in-Memory Architecture for Transformer
2023 60th ACM/IEEE Design Automation Conference (DAC)(2023)
摘要
Through the attention mechanism, Transformer-based large-scale deep neural networks (LSDNNs) have demonstrated remarkable achievements in artificial intelligence applications such as natural language processing and computer vision. The matrix-matrix multiplication operation (MMMO) in Transformer makes data movement dominate the inference overhead over computation. A solution for efficient data movement during Transformer inference is to embed arithmetic logic units (ALUs) into the memory array, hence an accelerator-in-memory architecture (AIMA). Existing work along this direction has not considered the heterogeneity of parallelism and resource requirements among Transformer layers. This increases the inference latency and lowers the resource utilization, which is critical for the embedded systems domain. To this end, we propose HAIMA, a hybrid AIMA and the parallel dataflow for Transformer, which exploit the cooperation between SRAM and DRAM to accelerate different MMMOs. Compared to the state-of-the-art Newton and TransPIM, our proposed hardware-software co-design achieves 1.4x-1.5x speedup, and solves the problem of resource under-utilization when DRAM-based AIMA performs the light-weight MMMOs.
更多查看译文
AI 理解论文
溯源树
样例
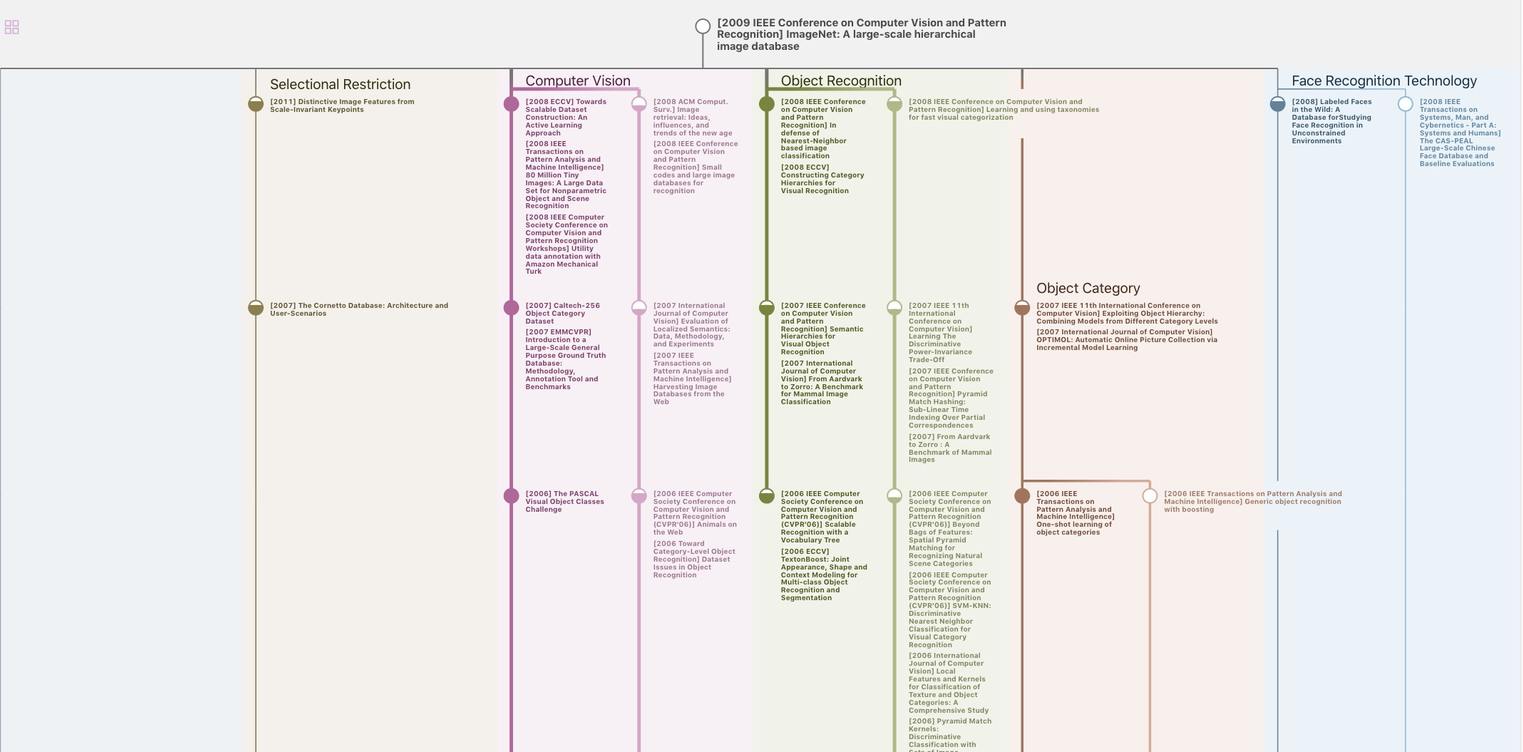
生成溯源树,研究论文发展脉络
Chat Paper
正在生成论文摘要