Leveraging Synthetic Data for Improving Chamber Ensemble Separation
2023 IEEE Workshop on Applications of Signal Processing to Audio and Acoustics (WASPAA)(2023)
摘要
In this work, we tackle the challenging problem of separating mono-phonic instrument mixtures found in chamber music from monaural recordings. This task differs from the Music Demixing Challenge where the task is to separate vocals, drums, and bass stems from mastered stereo tracks. In our task, we separate the instruments in a permutation invariant fashion such that our model is capable of separating any two monophonic instruments, including mixtures of the same instrument. This task is particularly difficult due to label ambiguity and high spectral overlap. In this paper, we present a pre-training strategy and data augmentation pipeline using the multi-mic renders from the synthetic chamber ensemble dataset EnsembleSet and evaluate its impact using real-world chamber ensemble recordings from the URMP dataset. Our data augmentation pipeline, using synthetic data, has resulted in up to a remarkable +5.14 dB cross-dataset performance improvement for time-domain separation models when tested on real data. Our fine-tuning strategy in conjunction with our data augmentation pipeline results in up to +10.62 dB performance improvement w.r.t. our baseline for chamber ensemble separation. We report a strong negative correlation between pitch overlap and separation performance with an average of 5 dB performance drop for examples with pitch overlaps. We also show that pre-training our model with string, wind, and brass ensembles helps with separation of vocal harmony mixtures from Bach Chorales and Barbershop Quartet datasets with up to +17.92 dB SI-SDR improvement for 2 source vocal harmony mixtures.
更多查看译文
关键词
chamber ensembles, domain adaptation, cross-dataset evaluation, monaural source separation
AI 理解论文
溯源树
样例
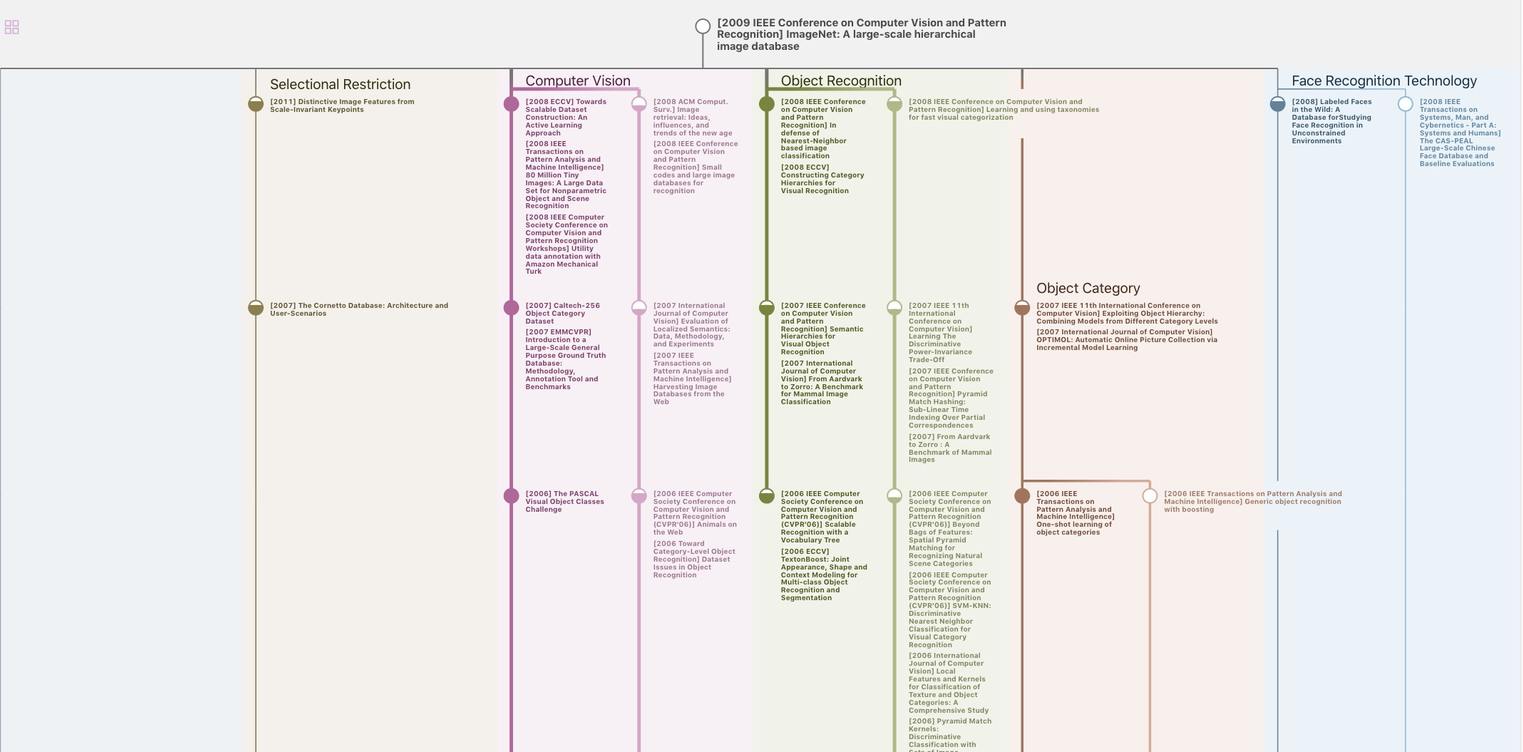
生成溯源树,研究论文发展脉络
Chat Paper
正在生成论文摘要