Folding Attention: Memory and Power Optimization for On-Device Transformer-based Streaming Speech Recognition
ICASSP 2024 - 2024 IEEE International Conference on Acoustics, Speech and Signal Processing (ICASSP)(2023)
摘要
Transformer-based models excel in speech recognition. Existing efforts to
optimize Transformer inference, typically for long-context applications, center
on simplifying attention score calculations. However, streaming speech
recognition models usually process a limited number of tokens each time, making
attention score calculation less of a bottleneck. Instead, the bottleneck lies
in the linear projection layers of multi-head attention and feedforward
networks, constituting a substantial portion of the model size and contributing
significantly to computation, memory, and power usage.
To address this bottleneck, we propose folding attention, a technique
targeting these linear layers, significantly reducing model size and improving
memory and power efficiency. Experiments on on-device Transformer-based
streaming speech recognition models show that folding attention reduces model
size (and corresponding memory consumption) by up to 24
by up to 23
更多查看译文
关键词
speech recognition,Transformer,attention,memory optimization,power optimization
AI 理解论文
溯源树
样例
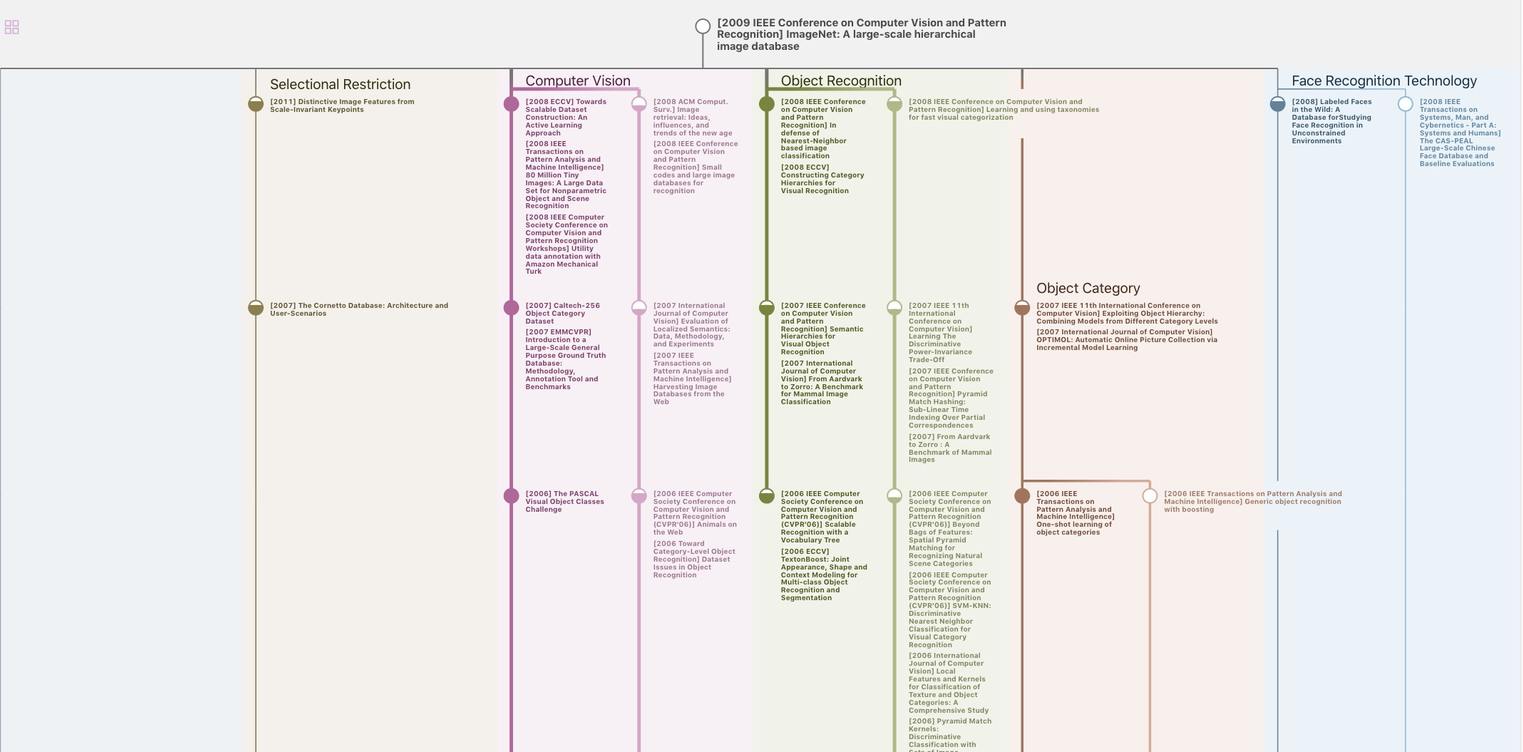
生成溯源树,研究论文发展脉络
Chat Paper
正在生成论文摘要