Syn-Att: Synthetic Speech Attribution via Semi-Supervised Unknown Multi-Class Ensemble of CNNs
CoRR(2023)
摘要
With the huge technological advances introduced by deep learning in audio & speech processing, many novel synthetic speech techniques achieved incredible realistic results. As these methods generate realistic fake human voices, they can be used in malicious acts such as people imitation, fake news, spreading, spoofing, media manipulations, etc. Hence, the ability to detect synthetic or natural speech has become an urgent necessity. Moreover, being able to tell which algorithm has been used to generate a synthetic speech track can be of preeminent importance to track down the culprit. In this paper, a novel strategy is proposed to attribute a synthetic speech track to the generator that is used to synthesize it. The proposed detector transforms the audio into log-mel spectrogram, extracts features using CNN, and classifies it between five known and unknown algorithms, utilizing semi-supervision and ensemble to improve its robustness and generalizability significantly. The proposed detector is validated on two evaluation datasets consisting of a total of 18,000 weakly perturbed (Eval 1) & 10,000 strongly perturbed (Eval 2) synthetic speeches. The proposed method outperforms other top teams in accuracy by 12-13% on Eval 2 and 1-2% on Eval 1, in the IEEE SP Cup challenge at ICASSP 2022.
更多查看译文
关键词
synthetic speech attribution,syn-att,semi-supervised,multi-class
AI 理解论文
溯源树
样例
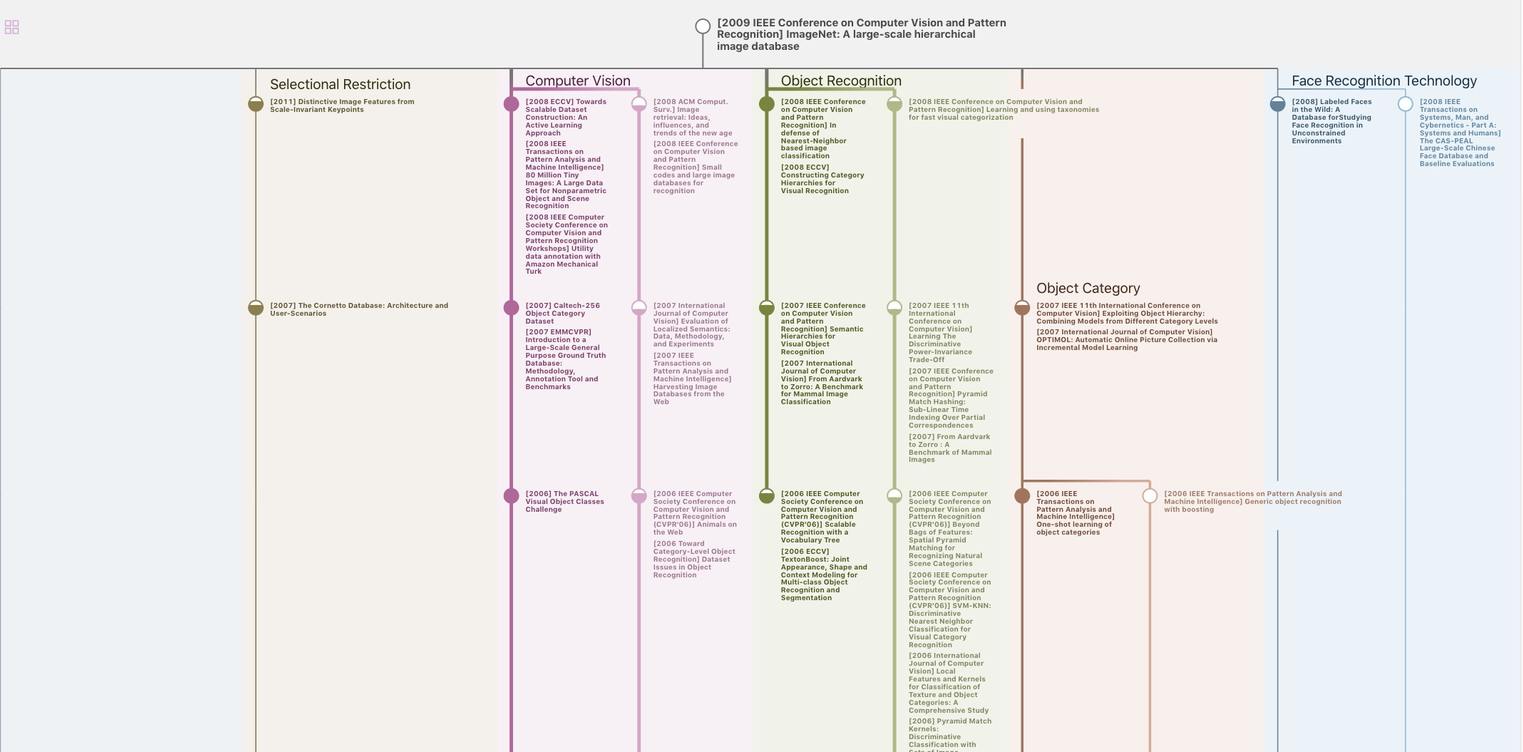
生成溯源树,研究论文发展脉络
Chat Paper
正在生成论文摘要