Sampling-Free Probabilistic Deep State-Space Models
CoRR(2023)
摘要
Many real-world dynamical systems can be described as State-Space Models (SSMs). In this formulation, each observation is emitted by a latent state, which follows first-order Markovian dynamics. A Probabilistic Deep SSM (ProDSSM) generalizes this framework to dynamical systems of unknown parametric form, where the transition and emission models are described by neural networks with uncertain weights. In this work, we propose the first deterministic inference algorithm for models of this type. Our framework allows efficient approximations for training and testing. We demonstrate in our experiments that our new method can be employed for a variety of tasks and enjoys a superior balance between predictive performance and computational budget.
更多查看译文
关键词
models,deep,sampling-free,state-space
AI 理解论文
溯源树
样例
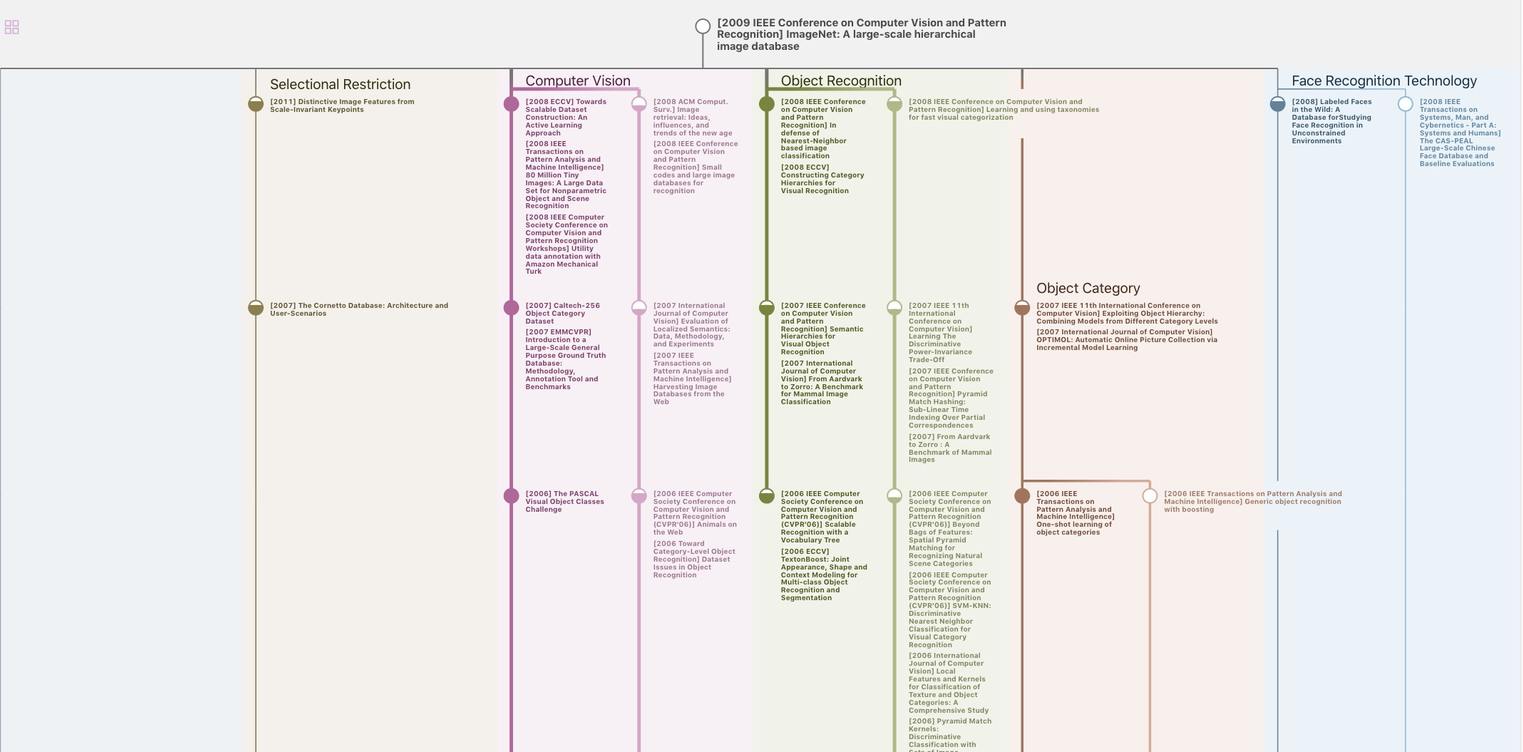
生成溯源树,研究论文发展脉络
Chat Paper
正在生成论文摘要