Augmented quantization: a general approach to mixture models
HAL (Le Centre pour la Communication Scientifique Directe)(2023)
摘要
The investigation of mixture models is a key to understand and visualize the distribution of multivariate data. Most mixture models approaches are based on likelihoods, and are not adapted to distribution with finite support or without a well-defined density function. This study proposes the Augmented Quantization method, which is a reformulation of the classical quantization problem but which uses the p-Wasserstein distance. This metric can be computed in very general distribution spaces, in particular with varying supports. The clustering interpretation of quantization is revisited in a more general framework. The performance of Augmented Quantization is first demonstrated through analytical toy problems. Subsequently, it is applied to a practical case study involving river flooding, wherein mixtures of Dirac and Uniform distributions are built in the input space, enabling the identification of the most influential variables.
更多查看译文
关键词
augmented quantization,models
AI 理解论文
溯源树
样例
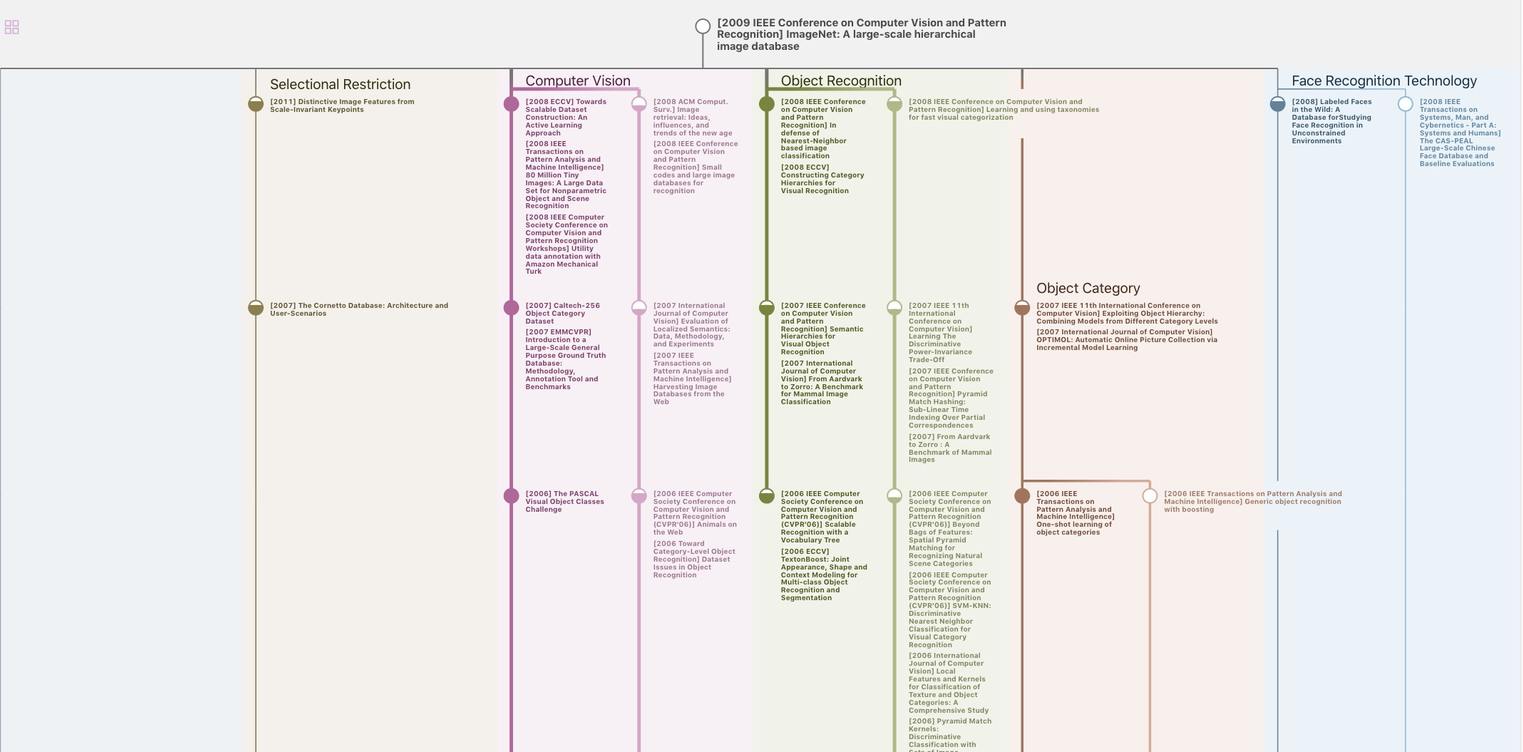
生成溯源树,研究论文发展脉络
Chat Paper
正在生成论文摘要