IHT-Inspired Neural Network for Single-Snapshot DOA Estimation with Sparse Linear Arrays
ICASSP 2024 - 2024 IEEE International Conference on Acoustics, Speech and Signal Processing (ICASSP)(2023)
摘要
Single-snapshot direction-of-arrival (DOA) estimation using sparse linear arrays (SLAs) has gained significant attention in the field of automotive MIMO radars. This is due to the dynamic nature of automotive settings, where multiple snapshots aren't accessible, and the importance of minimizing hardware costs. Low-rank Hankel matrix completion has been proposed to interpolate the missing elements in SLAs. However, the solvers of matrix completion, such as iterative hard thresholding (IHT), heavily rely on expert knowledge of hyperparameter tuning and lack task-specificity. Besides, IHT involves truncated-singular value decomposition (t-SVD), which has high computational cost in each iteration. In this paper, we propose an IHT-inspired neural network for single-snapshot DOA estimation with SLAs, termed IHT-Net. We utilize a recurrent neural network structure to parameterize the IHT algorithm. Additionally, we integrate shallow-layer autoencoders to replace t-SVD, reducing computational overhead while generating a novel optimizer through supervised learning. IHT-Net maintains strong interpretability as its network layer operations align with the iterations of the IHT algorithm. The learned optimizer exhibits fast convergence and higher accuracy in the full array signal reconstruction followed by single-snapshot DOA estimation. Numerical results validate the effectiveness of the proposed method.
更多查看译文
关键词
Sparse linear array,matrix completion,iterative hard thresholding,deep neural networks,single snapshot,direction-of-arrival estimation
AI 理解论文
溯源树
样例
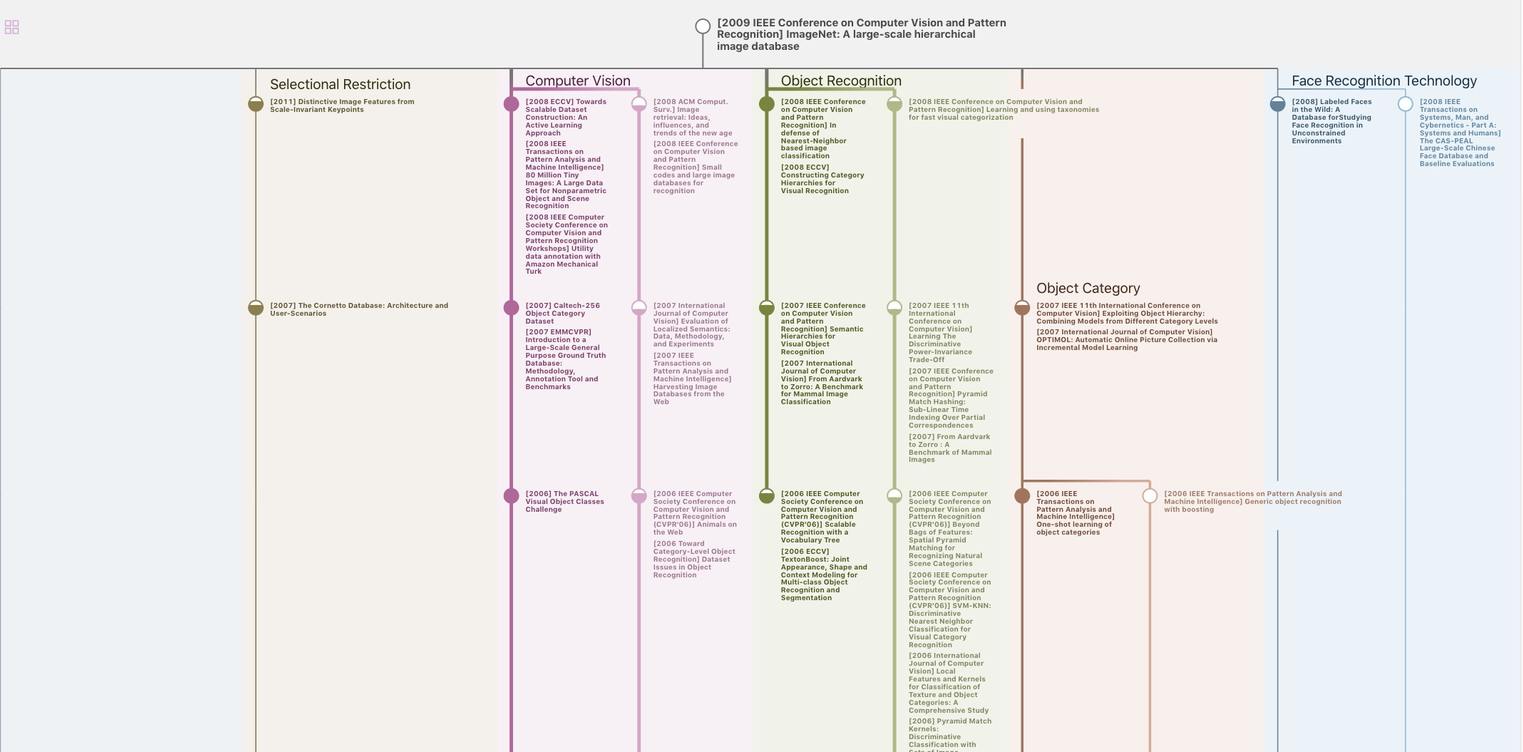
生成溯源树,研究论文发展脉络
Chat Paper
正在生成论文摘要