TOMAS: topology optimization of multiscale fluid flow devices using variational auto-encoders and super-shapes
CoRR(2024)
摘要
In this paper, we present a framework for multiscale topology optimization of fluid flow devices. The objective is to minimize dissipated power, subject to a desired contact area. The proposed strategy is to design optimal microstructures in individual finite element cells, while simultaneously optimizing the overall fluid flow. In particular, parameterized super-shapes are chosen here to represent microstructures since they exhibit a wide range of permeability and contact area. To avoid repeated homogenization, a finite set of these super-shapes are analyzed a priori and a variational auto-encoder (VAE) is trained on their fluid constitutive properties (permeability), contact area, and shape parameters. The resulting differentiable latent space is integrated with a coordinate neural network to carry out a global multiscale fluid flow optimization. The latent space enables the use of new microstructures that were not present in the original dataset. The proposed method is illustrated using numerous examples in 2D.
更多查看译文
关键词
Topology optimization,Multiscale,Stokes flow,Variational auto-encoders,Super-shapes
AI 理解论文
溯源树
样例
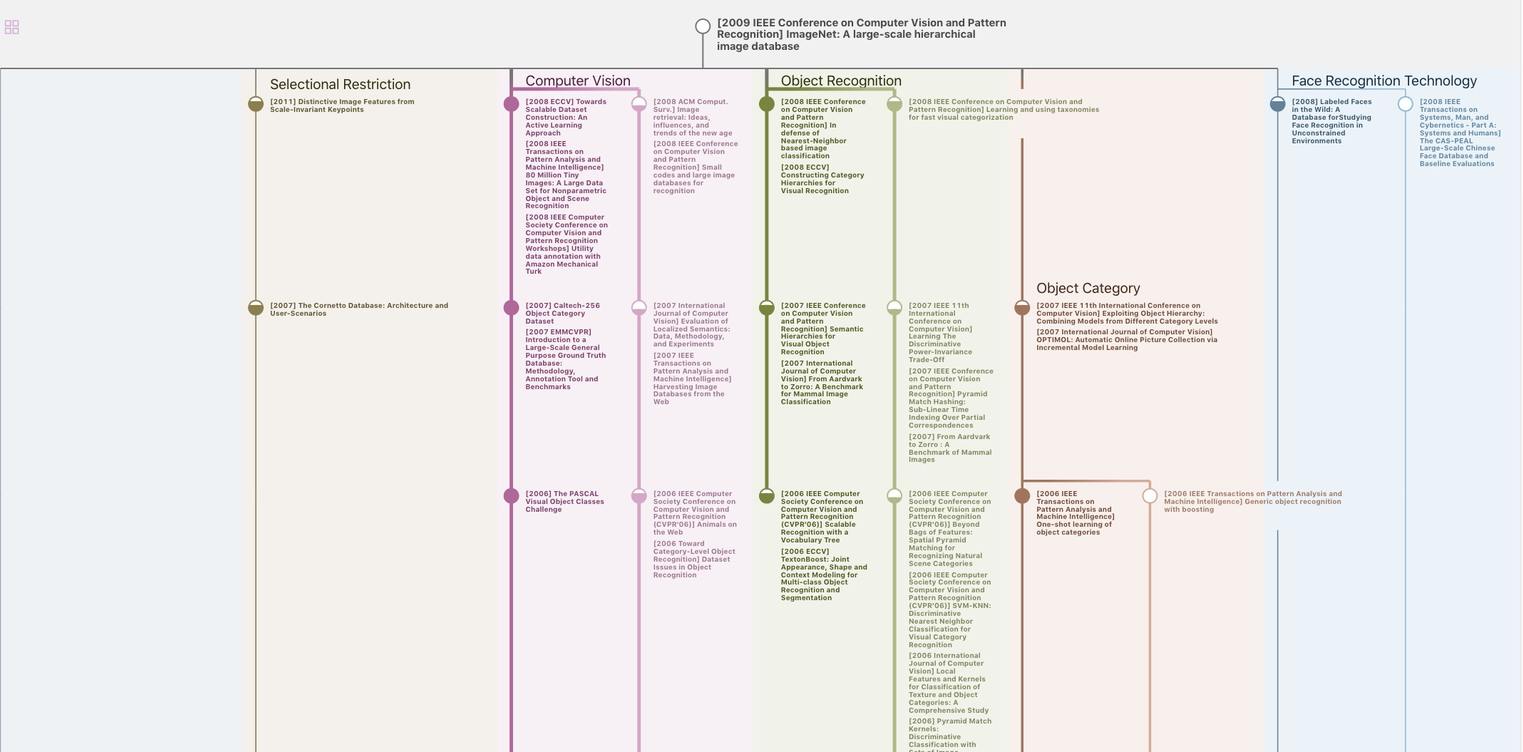
生成溯源树,研究论文发展脉络
Chat Paper
正在生成论文摘要