A Random Graph-based Autoregressive Model for Networked Time Series
arXiv (Cornell University)(2023)
摘要
Contemporary time series data often feature objects connected by a social network that naturally induces temporal dependence involving connected neighbours. The network vector autoregressive model is useful for describing the influence of linked neighbours, while recent generalizations aim to separate influence and homophily. Existing approaches, however, require either correct specification of a time series model or accurate estimation of a network model or both, and rely exclusively on least-squares for parameter estimation. This paper proposes a new autoregressive model incorporating a flexible form for latent variables used to depict homophily. We develop a first-order differencing method for the estimation of influence requiring only the influence part of the model to be correctly specified. When the part including homophily is correctly specified admitting a semiparametric form, we leverage and generalize the recent notion of neighbour smoothing for parameter estimation, bypassing the need to specify the generative mechanism of the network. We develop new theory to show that all the estimated parameters are consistent and asymptotically normal. The efficacy of our approach is confirmed via extensive simulations and an analysis of a social media dataset.
更多查看译文
关键词
autoregressive model,graph-based
AI 理解论文
溯源树
样例
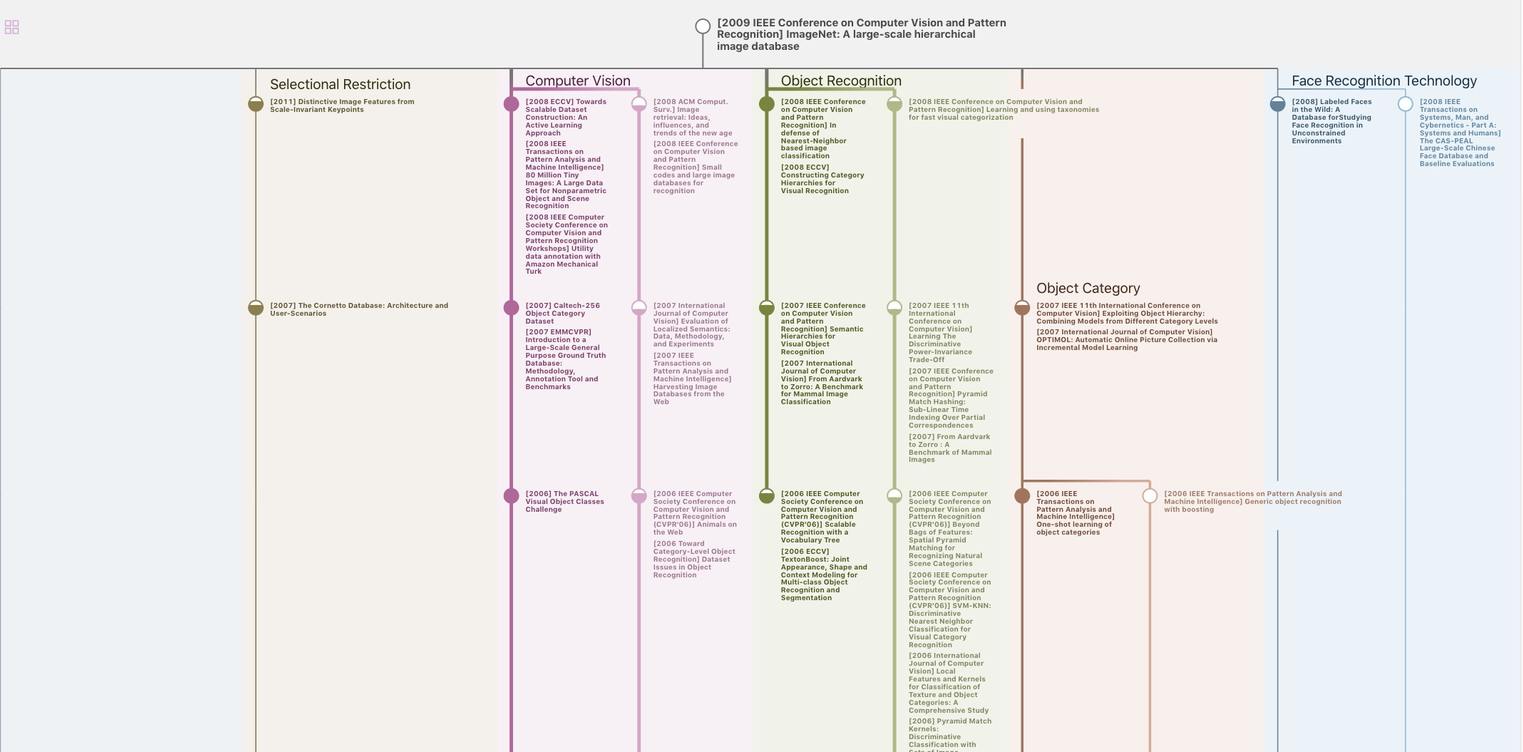
生成溯源树,研究论文发展脉络
Chat Paper
正在生成论文摘要