A semi-supervised medical image classification method based on combined pseudo-labeling and distance metric consistency
MULTIMEDIA TOOLS AND APPLICATIONS(2023)
摘要
In medical image analysis, obtaining high-quality labeled data is expensive, and there is a large amount of unlabeled image data that is not utilized. Semi-supervised learning can use unlabeled data to improve the model performance in the presence of data scarcity medical image analysis. In this paper, we propose a semi-supervised framework for medical image classification considering both feature extraction layer information and semantic classification layer information. It is a method that includes a combined pseudo-labeling strategy and a feature distance metric consistency method. Compared with other semi-supervised classification methods, our method significantly improves the accuracy of pseudo-labels by combining the feature metric pseudo-label with the semantic classification pseudo-label and enables the model to explore deeper information by constraining the distance relations of sample features in the feature space. We conducted extensive experiments on two public medical image datasets to demonstrate that our method outperforms various state-of-the-art semi-supervised learning methods.
更多查看译文
关键词
Semi-Supervised learning,Medical image classification,Pseudo-label,Consistency regularization
AI 理解论文
溯源树
样例
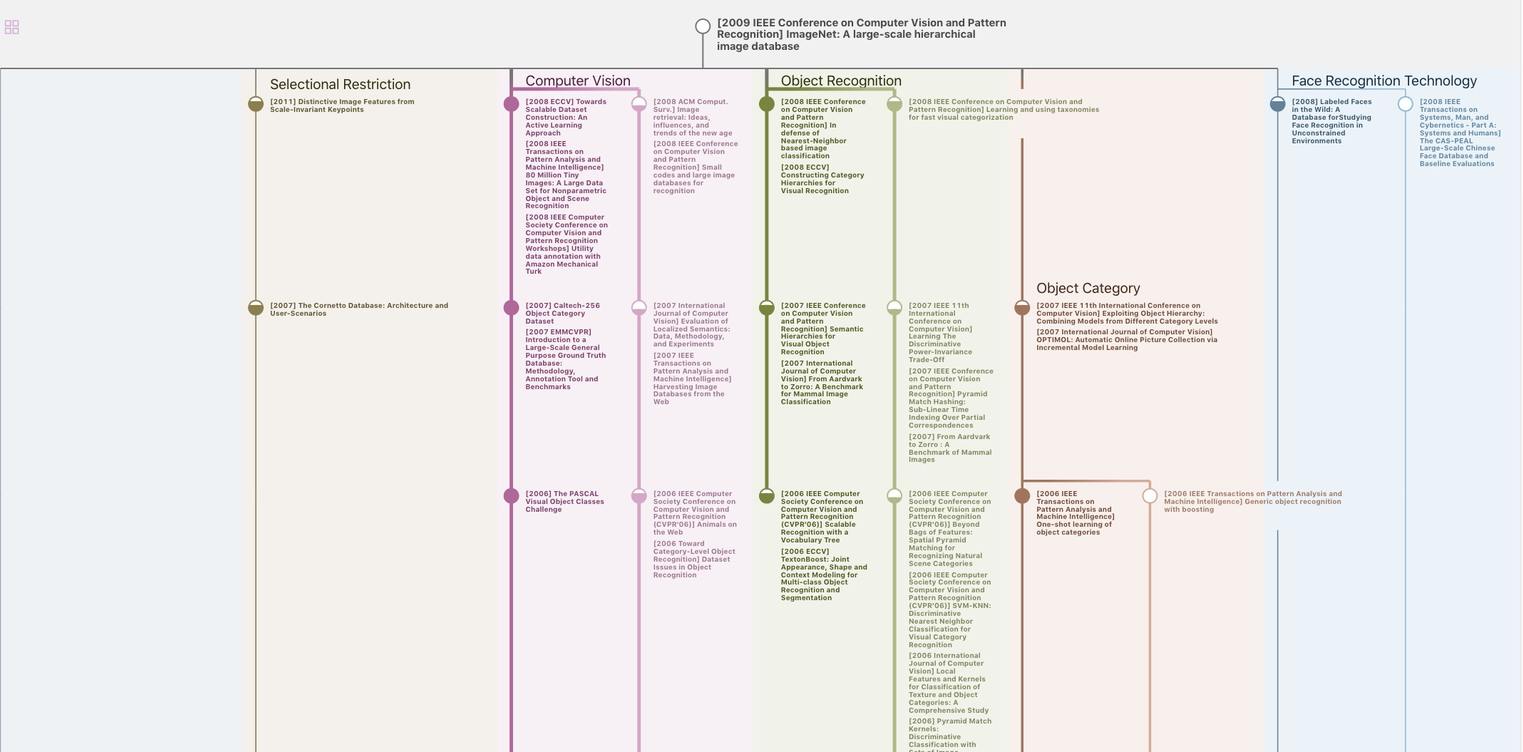
生成溯源树,研究论文发展脉络
Chat Paper
正在生成论文摘要