Iterative learning for maxillary sinus segmentation based on bounding box annotations
MULTIMEDIA TOOLS AND APPLICATIONS(2023)
摘要
An accurate segmentation of the maxillary sinus (MS) is helpful for preoperative planning of dental implantation, diagnosis and evaluation of sinusitis, and validation of radiotherapy for sinus cancer. Many medical image segmentation models based on convolutional neural networks have achieved excellent performance, however, relied heavily on manual accurate labeling of training data. We propose an iterative learning method for MS segmentation with only bounding box supervision. First, a cone-beam computed tomography (CBCT) image is over-segmented into a set of superpixels and a feature extraction network is optimized to better extract multi-scale features of each small-size superpixel. Second, an improved graph convolutional network (IGCN) is developed to merge superpixel regions and improve the feature transformation ability of each node on a superpixel-wise graph. Finally, the iterative learning combined with the superpixel-conditional random field and IGCN makes pseudo labels gradually refine and close to fully supervised information. On a practical MS dataset, the proposed method achieves 90.5% in Dice similarity coefficient. Extending to the public dataset Promise12 for prostate MR image segmentation, it also performs well. The results show that our proposed method has good comprehensive weakly supervised segmentation performance and can narrow a gap between the bounding box and full supervision.
更多查看译文
关键词
Maxillary sinus segmentation,Bounding boxes,Weakly supervised,Superpixel classification,Graph convolution network,Iterative learning
AI 理解论文
溯源树
样例
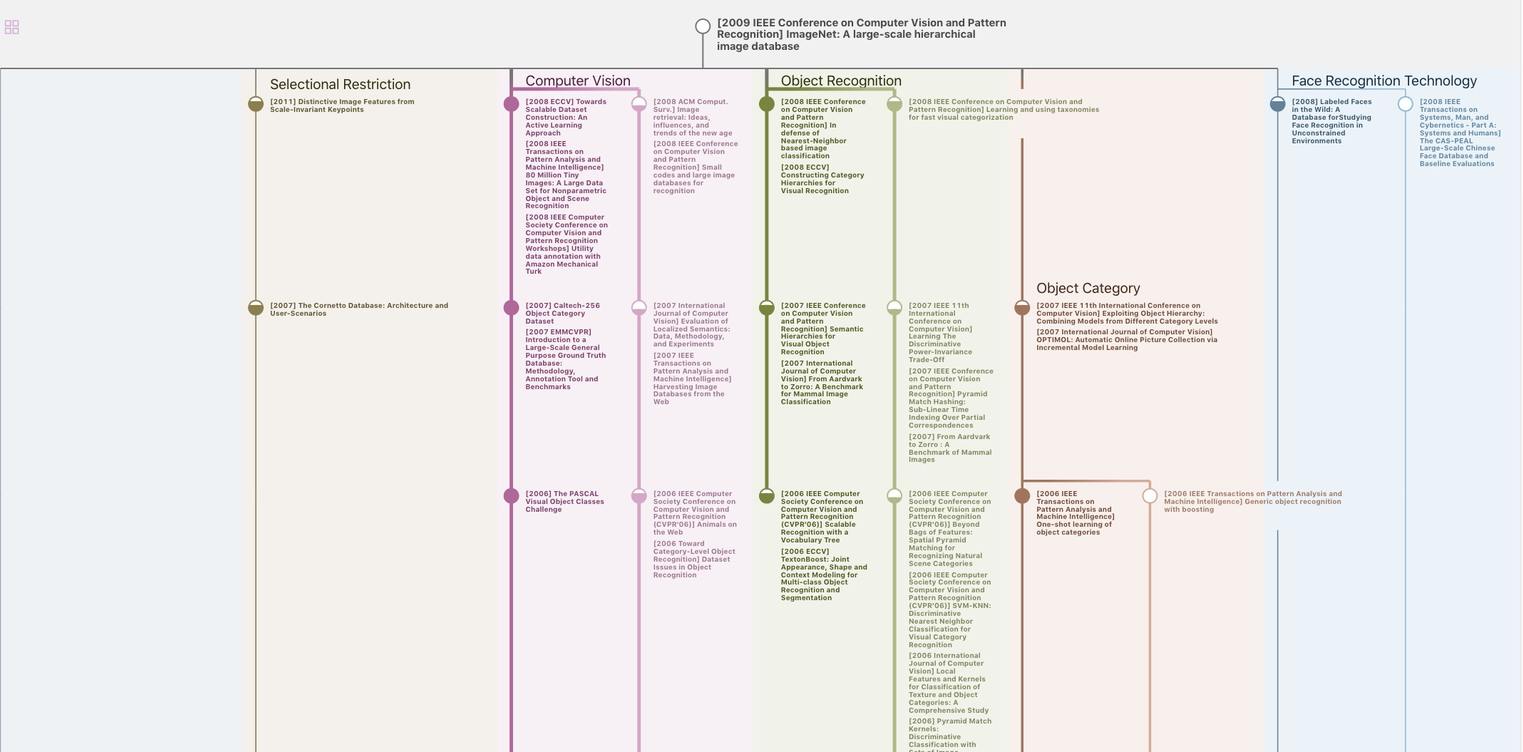
生成溯源树,研究论文发展脉络
Chat Paper
正在生成论文摘要