MSA-YOLOv5: Multi-scale Attention-Based YOLOv5 for Automatic Detection of Acute Ischemic Stroke from Multi-Modality MRI Images.
Comput Biol Medicine(2023)
摘要
BACKGROUND AND OBJECTIVE:Acute ischemic stroke (AIS) is a common neurological disorder characterized by the sudden onset of cerebral ischemia, leading to functional impairments. Swift and precise detection of AIS lesions is crucial for stroke diagnosis and treatment but poses a significant challenge. This study aims to leverage multimodal fusion technology to combine complementary information from various modalities, thereby enhancing the detection performance of AIS target detection models.METHODS:In this retrospective study of AIS, we collected data from 316 AIS patients and created a multi-modality magnetic resonance imaging (MRI) dataset. We propose a Multi-Scale Attention-based YOLOv5 (MSA-YOLOv5), targeting challenges such as small lesion size and blurred borders at low resolutions. Specifically, we augment YOLOv5 with a prediction head to detect objects at various scales. Next, we replace the original prediction head with a Multi-Scale Swin Transformer Prediction Head (MS-STPH), which reduces computational complexity to linear levels and enhances the ability to detect small lesions. We incorporate a Second-Order channel attention (SOCA) module to adaptively rescale channel features by employing second-order feature statistics for more discriminative representations. Finally, we further validate the effectiveness of our method using the ISLES 2022 dataset.RESULTS:On our in-house AIS dataset, MSA-YOLOv5 achieves a 79.0% mAP0.5, substantially surpassing other single-stage models. Compared to two-stage models, it maintains a comparable performance level while significantly reducing the number of parameters and resolution. On the ISLES 2022 dataset, MSA-YOLOv5 attains an 80.0% mAP0.5, outperforming other network models by a considerable margin. MS-STPH and SOCA modules can significantly increase mAP0.5 by 2.7% and 1.9%, respectively. Visualization interpretability results show that the proposed MSA-YOLOv5 restricts high attention in the small regions of AIS lesions.CONCLUSIONS:The proposed MSA-YOLOv5 is capable of automatically and effectively detecting acute ischemic stroke lesions in multimodal images, particularly for small lesions and artifacts. Our enhanced model reduces the number of parameters while improving detection accuracy. This model can potentially assist radiologists in providing more accurate diagnosis, and enable clinicians to develop better treatment plans.
更多查看译文
关键词
Acute ischemic stroke,Object detection,Multi-modality MRI,Deep learning,YOLOv5,Attention mechanism
AI 理解论文
溯源树
样例
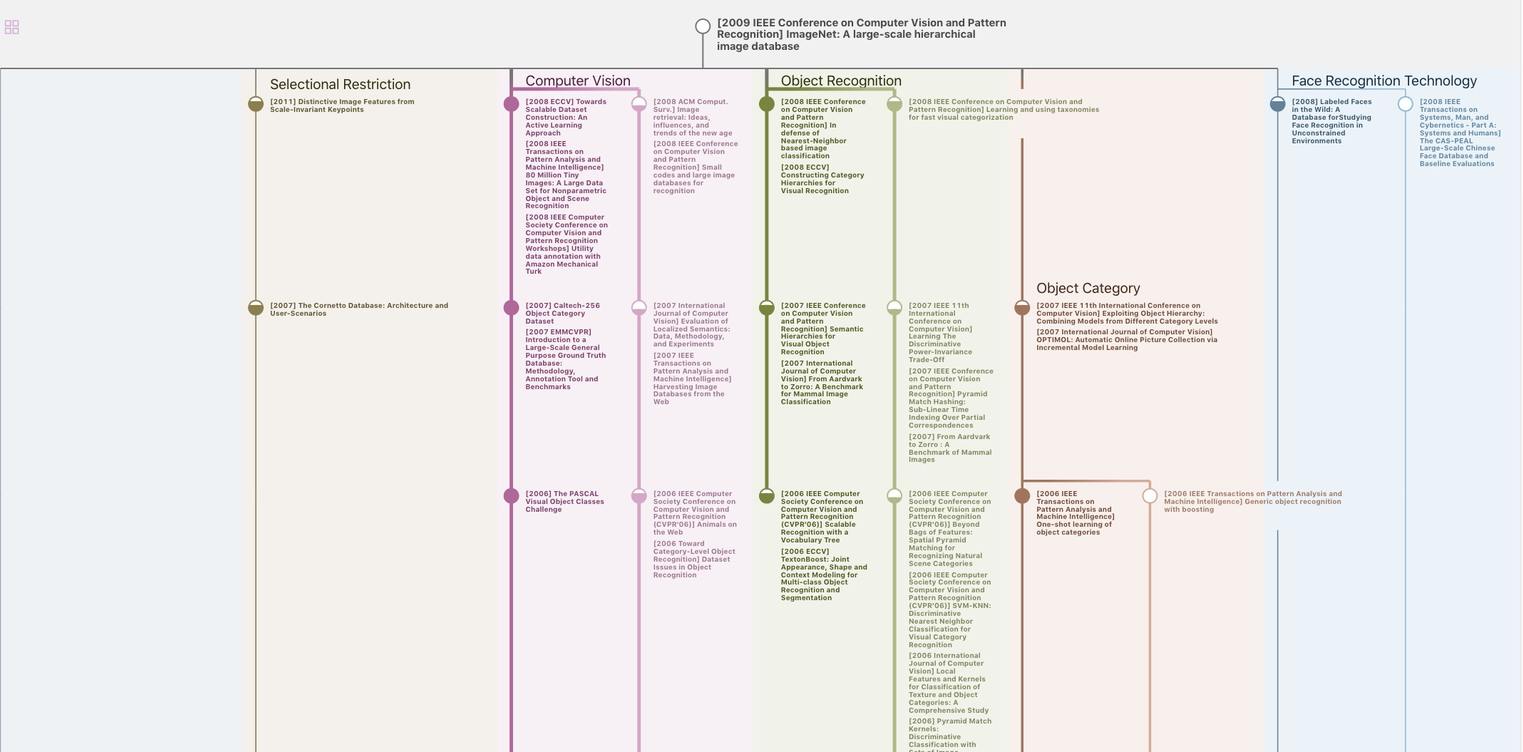
生成溯源树,研究论文发展脉络
Chat Paper
正在生成论文摘要