Astronomaly at scale: searching for anomalies amongst 4 million galaxies
MONTHLY NOTICES OF THE ROYAL ASTRONOMICAL SOCIETY(2024)
摘要
Modern astronomical surveys are producing data sets of unprecedented size and richness, increasing the potential for high-impact scientific discovery. This possibility, coupled with the challenge of exploring a large number of sources, has led to the development of novel machine-learning-based anomaly detection approaches, such as astronomaly. For the first time, we test the scalability of astronomaly by applying it to almost 4 million images of galaxies from the Dark Energy Camera Legacy Survey. We use a trained deep learning algorithm to learn useful representations of the images and pass these to the anomaly detection algorithm isolation forest, coupled with astronomaly's active learning method, to discover interesting sources. We find that data selection criteria have a significant impact on the trade-off between finding rare sources such as strong lenses and introducing artefacts into the data set. We demonstrate that active learning is required to identify the most interesting sources and reduce artefacts, while anomaly detection methods alone are insufficient. Using astronomaly, we find 1635 anomalies among the top 2000 sources in the data set after applying active learning, including eight strong gravitational lens candidates, 1609 galaxy merger candidates, and 18 previously unidentified sources exhibiting highly unusual morphology. Our results show that by leveraging the human-machine interface, astronomaly is able to rapidly identify sources of scientific interest even in large data sets.
更多查看译文
关键词
gravitational lensing: strong,methods: data analysis,surveys,galaxies: general,galaxies: interactions
AI 理解论文
溯源树
样例
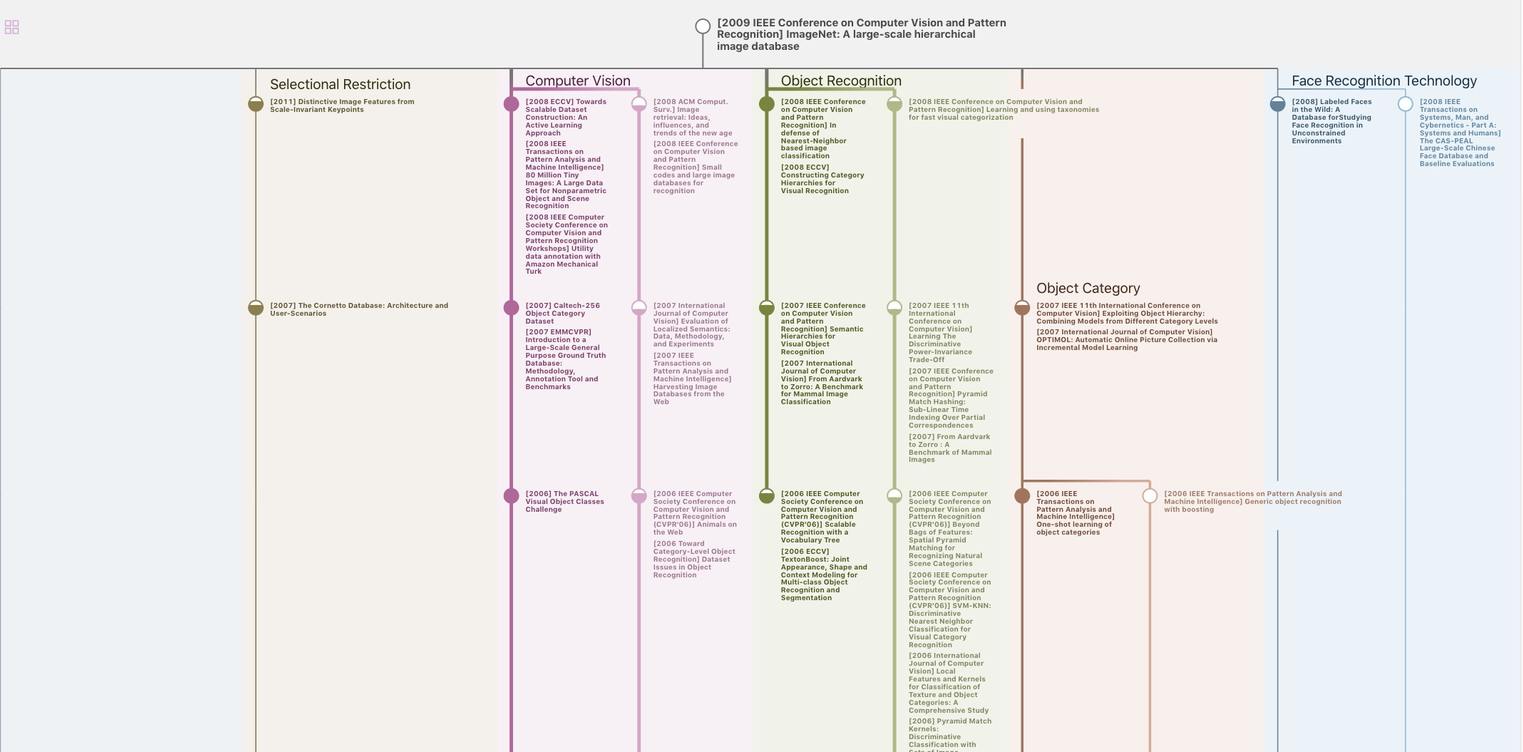
生成溯源树,研究论文发展脉络
Chat Paper
正在生成论文摘要