A computational framework for generating patient-specific vascular models and assessing uncertainty from medical images
arXiv (Cornell University)(2023)
摘要
Patient-specific computational modeling is a popular, non-invasive method to answer medical questions. Medical images are used to extract geometric domains necessary to create these models, providing a predictive tool for clinicians. However, in vivo imaging is subject to uncertainty, impacting vessel dimensions essential to the mathematical modeling process. While there are numerous programs available to provide information about vessel length, radii, and position, there is currently no exact way to determine and calibrate these features. This raises the question, if we are building patient-specific models based on uncertain measurements, how accurate are the geometries we extract and how can we best represent a patient's vasculature? In this study, we develop a novel framework to determine vessel dimensions using change points. We explore the impact of uncertainty in the network extraction process on hemodynamics by varying vessel dimensions and segmenting the same images multiple times. Our analyses reveal that image segmentation, network size, and minor changes in radius and length have significant impacts on pressure and flow dynamics in rapidly branching structures and tapering vessels. Accordingly, we conclude that it is critical to understand how uncertainty in network geometry propagates to fluid dynamics, especially in clinical applications.
更多查看译文
关键词
medical images,models,uncertainty,computational framework,patient-specific
AI 理解论文
溯源树
样例
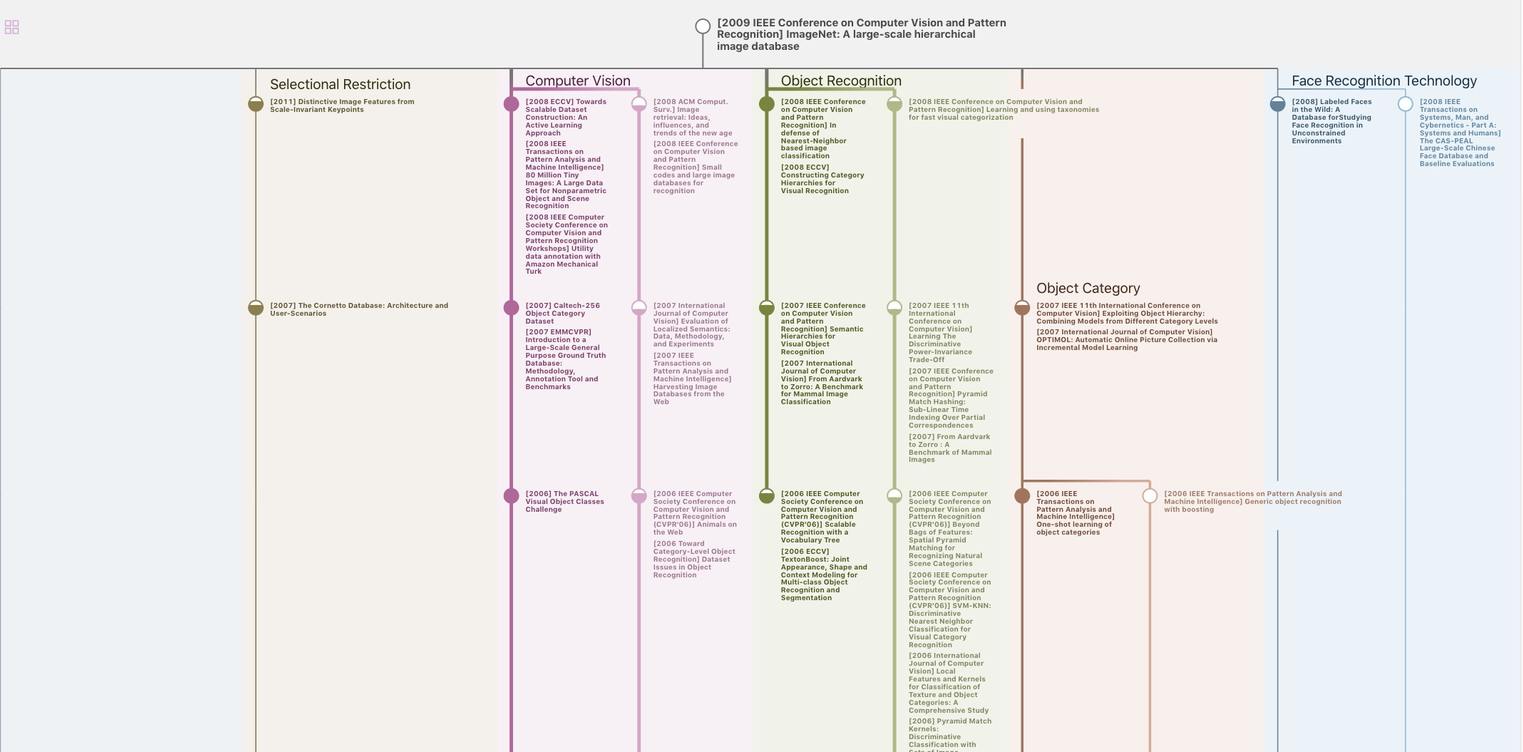
生成溯源树,研究论文发展脉络
Chat Paper
正在生成论文摘要