NOMAD: A Natural, Occluded, Multi-scale Aerial Dataset, for Emergency Response Scenarios
2024 IEEE/CVF Winter Conference on Applications of Computer Vision (WACV)(2023)
摘要
With the increasing reliance on small Unmanned Aerial Systems (sUAS) for Emergency Response Scenarios, such as Search and Rescue, the integration of computer vision capabilities has become a key factor in mission success. Nevertheless, computer vision performance for detecting humans severely degrades when shifting from ground to aerial views. Several aerial datasets have been created to mitigate this problem, however, none of them has specifically addressed the issue of occlusion, a critical component in Emergency Response Scenarios. Natural Occluded Multi-scale Aerial Dataset (NOMAD) presents a benchmark for human detection under occluded aerial views, with five different aerial distances and rich imagery variance. NOMAD is composed of 100 different Actors, all performing sequences of walking, laying and hiding. It includes 42,825 frames, extracted from 5.4k resolution videos, and manually annotated with a bounding box and a label describing 10 different visibility levels, categorized according to the percentage of the human body visible inside the bounding box. This allows computer vision models to be evaluated on their detection performance across different ranges of occlusion. NOMAD is designed to improve the effectiveness of aerial search and rescue and to enhance collaboration between sUAS and humans, by providing a new benchmark dataset for human detection under occluded aerial views.
更多查看译文
关键词
emergency,multi-scale
AI 理解论文
溯源树
样例
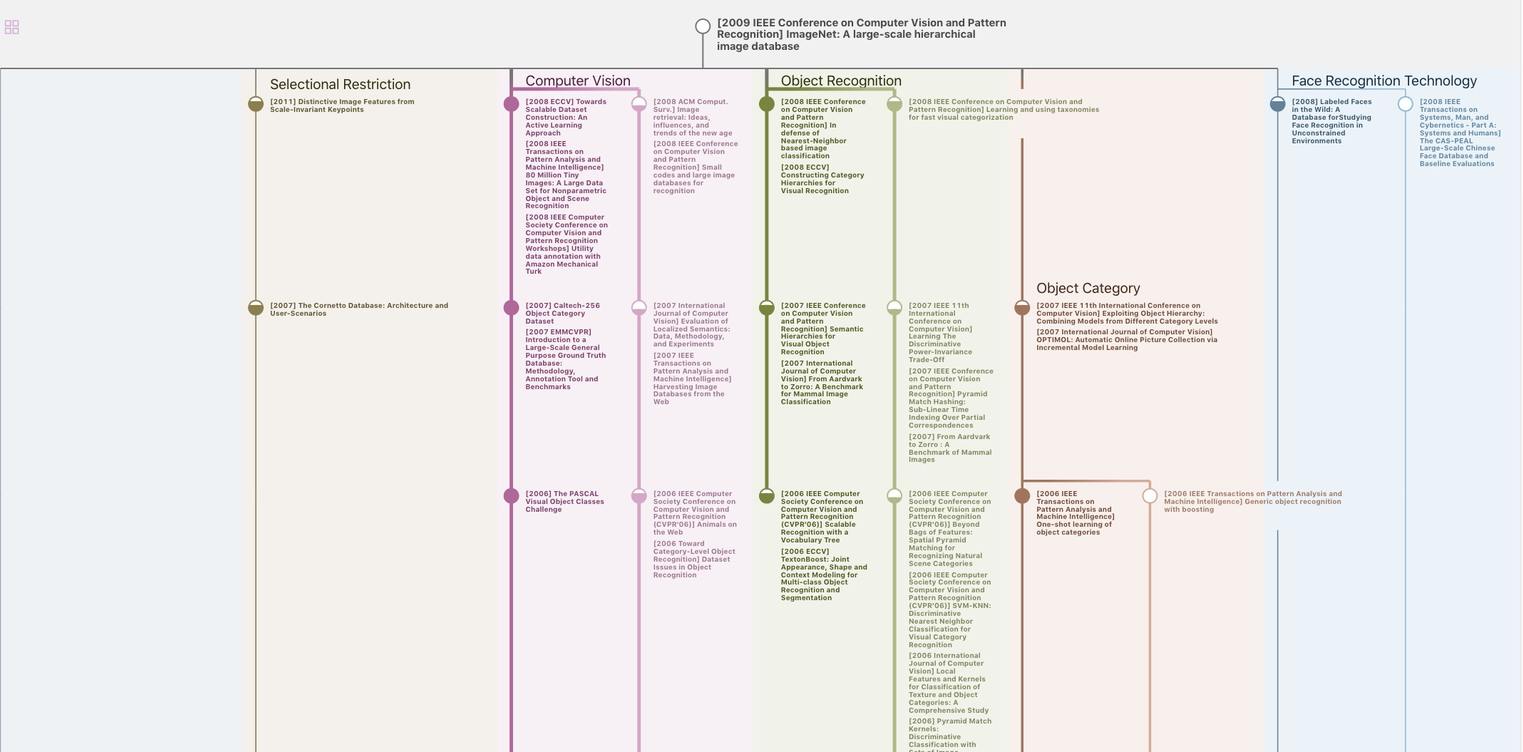
生成溯源树,研究论文发展脉络
Chat Paper
正在生成论文摘要