DGM-DR: Domain Generalization with Mutual Information Regularized Diabetic Retinopathy Classification
DOMAIN ADAPTATION AND REPRESENTATION TRANSFER, DART 2023(2024)
摘要
The domain shift between training and testing data presents a significant challenge for training generalizable deep learning models. As a consequence, the performance of models trained with the independent and identically distributed (i.i.d) assumption deteriorates when deployed in the real world. This problem is exacerbated in the medical imaging context due to variations in data acquisition across clinical centers, medical apparatus, and patients. Domain generalization (DG) aims to address this problem by learning a model that generalizes well to any unseen target domain. Many domain generalization techniques were unsuccessful in learning domain-invariant representations due to the large domain shift. Furthermore, multiple tasks in medical imaging are not yet extensively studied in existing literature when it comes to DG point of view. In this paper, we introduce a DG method that re-establishes the model objective function as a maximization of mutual information with a large pretrained model to the medical imaging field. We re-visit the problem of DG in Diabetic Retinopathy (DR) classification to establish a clear benchmark with a correct model selection strategy and to achieve robust domain-invariant representation for an improved generalization. Moreover, we conduct extensive experiments on public datasets to show that our proposed method consistently outperforms the previous state-of-the-art by a margin of 5.25% in average accuracy and a lower standard deviation. Source code available at https://github.com/BioMedIA-MBZUAI/DGM-DR.
更多查看译文
关键词
Domain Generalization,Diabetic Retinopathy,Mutual Information Regularization
AI 理解论文
溯源树
样例
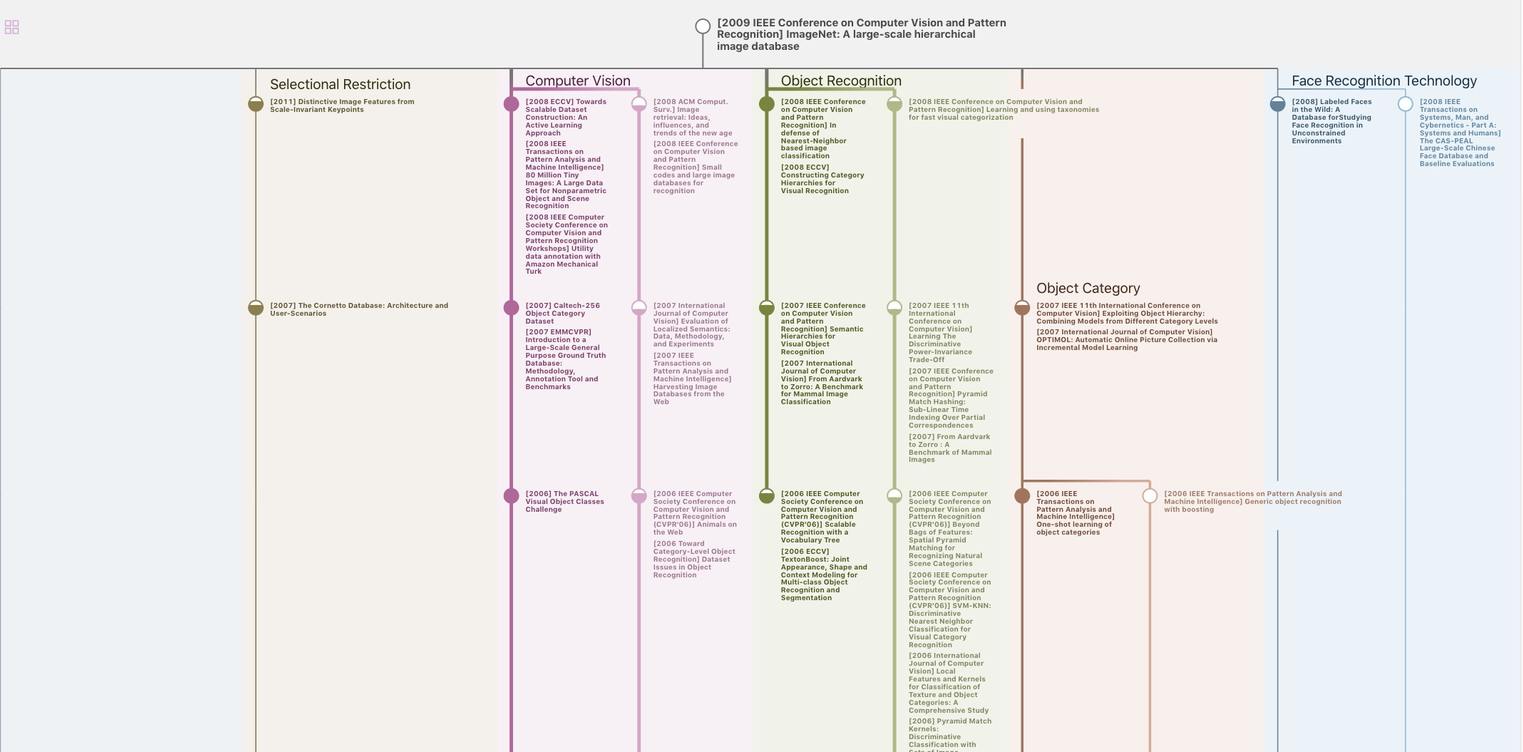
生成溯源树,研究论文发展脉络
Chat Paper
正在生成论文摘要