Towards Socially Responsive Autonomous Vehicles: A Reinforcement Learning Framework with Driving Priors and Coordination Awareness
IEEE Transactions on Intelligent Vehicles(2023)
摘要
The advent of autonomous vehicles (AVs) alongside human-driven vehicles (HVs) has ushered in an era of mixed traffic flow, presenting a significant challenge: the intricate interaction between these entities within complex driving environments. AVs are expected to have human-like driving behavior to seamlessly integrate into human-dominated traffic systems. To address this issue, we propose a reinforcement learning framework that considers driving priors and Social Coordination Awareness (SCA) to optimize the behavior of AVs. The framework integrates a driving prior learning (DPL) model based on a variational autoencoder to infer the driver's driving priors from human drivers' trajectories. A policy network based on a multi-head attention mechanism is designed to effectively capture the interactive dependencies between AVs and other traffic participants to improve decision-making quality. The introduction of SCA into the autonomous driving decision-making system, and the use of Coordination Tendency (CT) to quantify the willingness of AVs to coordinate the traffic system is explored. Simulation results show that the proposed framework can not only improve the decision-making quality of AVs but also motivate them to produce social behaviors, with potential benefits for the safety and traffic efficiency of the entire transportation system.
更多查看译文
关键词
Autonomous Vehicle,Reinforcement Learning,Driving Prior Learning,Autoencoder,Social Coordination
AI 理解论文
溯源树
样例
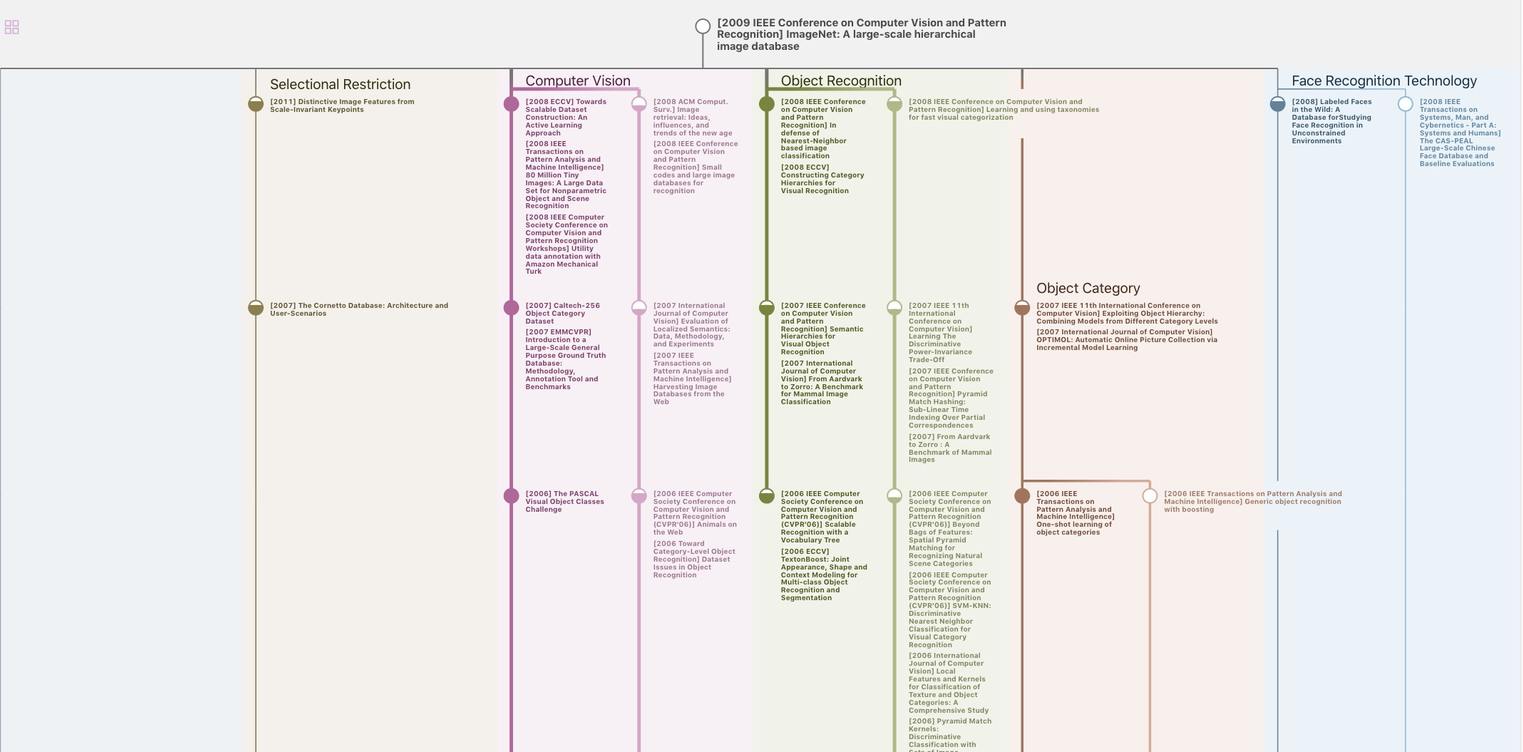
生成溯源树,研究论文发展脉络
Chat Paper
正在生成论文摘要