Contrastive self-representation learning for data clustering.
Neural networks : the official journal of the International Neural Network Society(2023)
摘要
This paper is concerned with self-representation subspace learning. It is one of the most representative subspace techniques, which has attracted considerable attention for clustering due to its good performance. Among these methods, low-rank representation (LRR) has achieved impressive results for subspace clustering. However, it only considers the similarity between the data itself, while neglecting the differences with other samples. Besides, it cannot well deal with noise and portray cluster-to-cluster relationships well. To solve these problems, we propose a Contrastive Self-representation model for Clustering (CSC). CSC simultaneously takes into account the similarity/dissimilarity between positive/negative pairs when learning the self-representation coefficient matrix of data while the form of the loss function can reduce the effect of noise on the results. Moreover, We use the ℓ-norm regularizer on the coefficient matrix to achieve its sparsity to better characterize the cluster structure. Thus, the learned self-representation coefficient matrix well encodes both the discriminative information and cluster structure. Extensive experiments on seven benchmark databases indicate the superiority of our proposed method.
更多查看译文
关键词
Self-representation,Contrastive learning,Subspace clustering
AI 理解论文
溯源树
样例
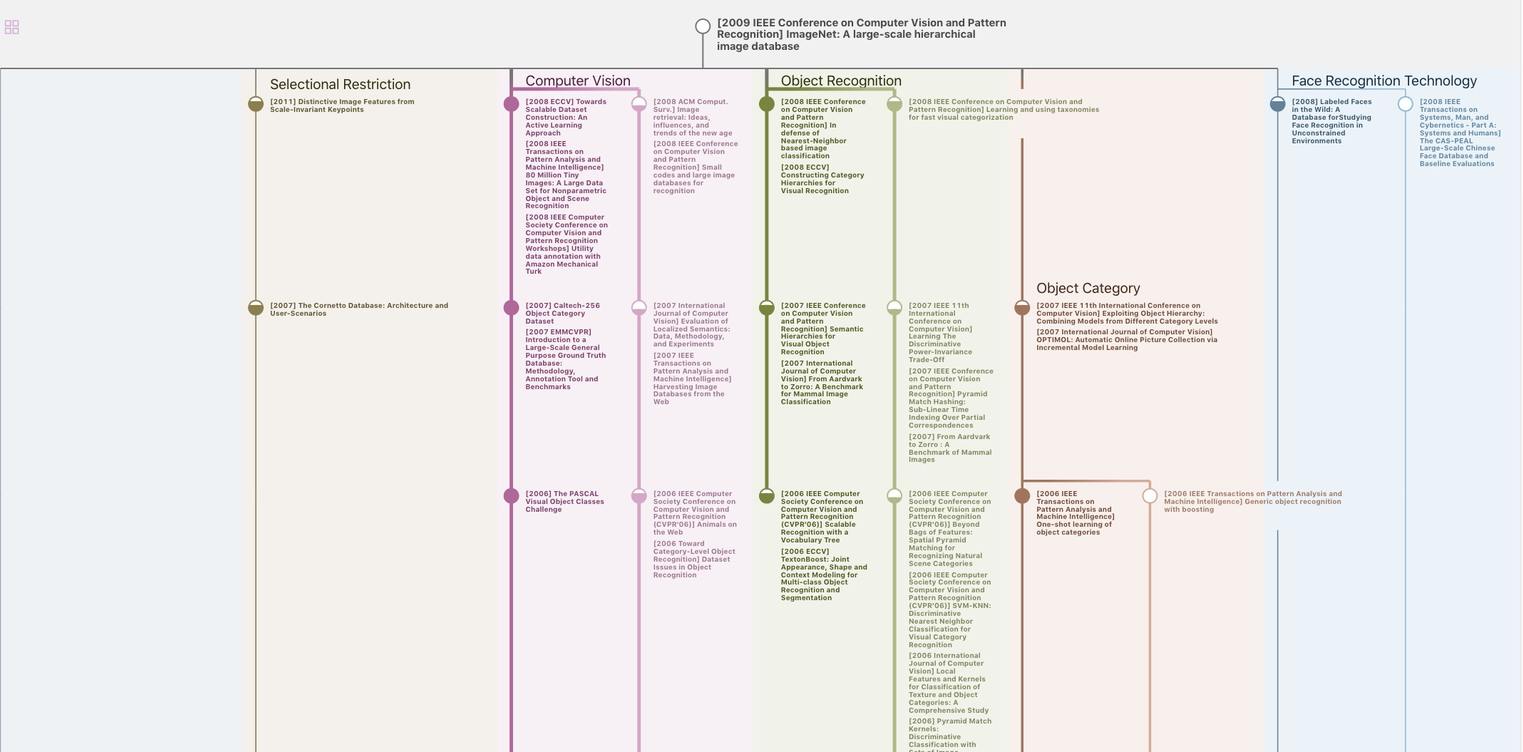
生成溯源树,研究论文发展脉络
Chat Paper
正在生成论文摘要