RGB-based Category-level Object Pose Estimation via Decoupled Metric Scale Recovery
CoRR(2023)
摘要
While showing promising results, recent RGB-D camera-based category-level object pose estimation methods have restricted applications due to the heavy reliance on depth sensors. RGB-only methods provide an alternative to this problem yet suffer from inherent scale ambiguity stemming from monocular observations. In this paper, we propose a novel pipeline that decouples the 6D pose and size estimation to mitigate the influence of imperfect scales on rigid transformations. Specifically, we leverage a pre-trained monocular estimator to extract local geometric information, mainly facilitating the search for inlier 2D-3D correspondence. Meanwhile, a separate branch is designed to directly recover the metric scale of the object based on category-level statistics. Finally, we advocate using the RANSAC-P$n$P algorithm to robustly solve for 6D object pose. Extensive experiments have been conducted on both synthetic and real datasets, demonstrating the superior performance of our method over previous state-of-the-art RGB-based approaches, especially in terms of rotation accuracy.
更多查看译文
关键词
object,rgb-based,category-level
AI 理解论文
溯源树
样例
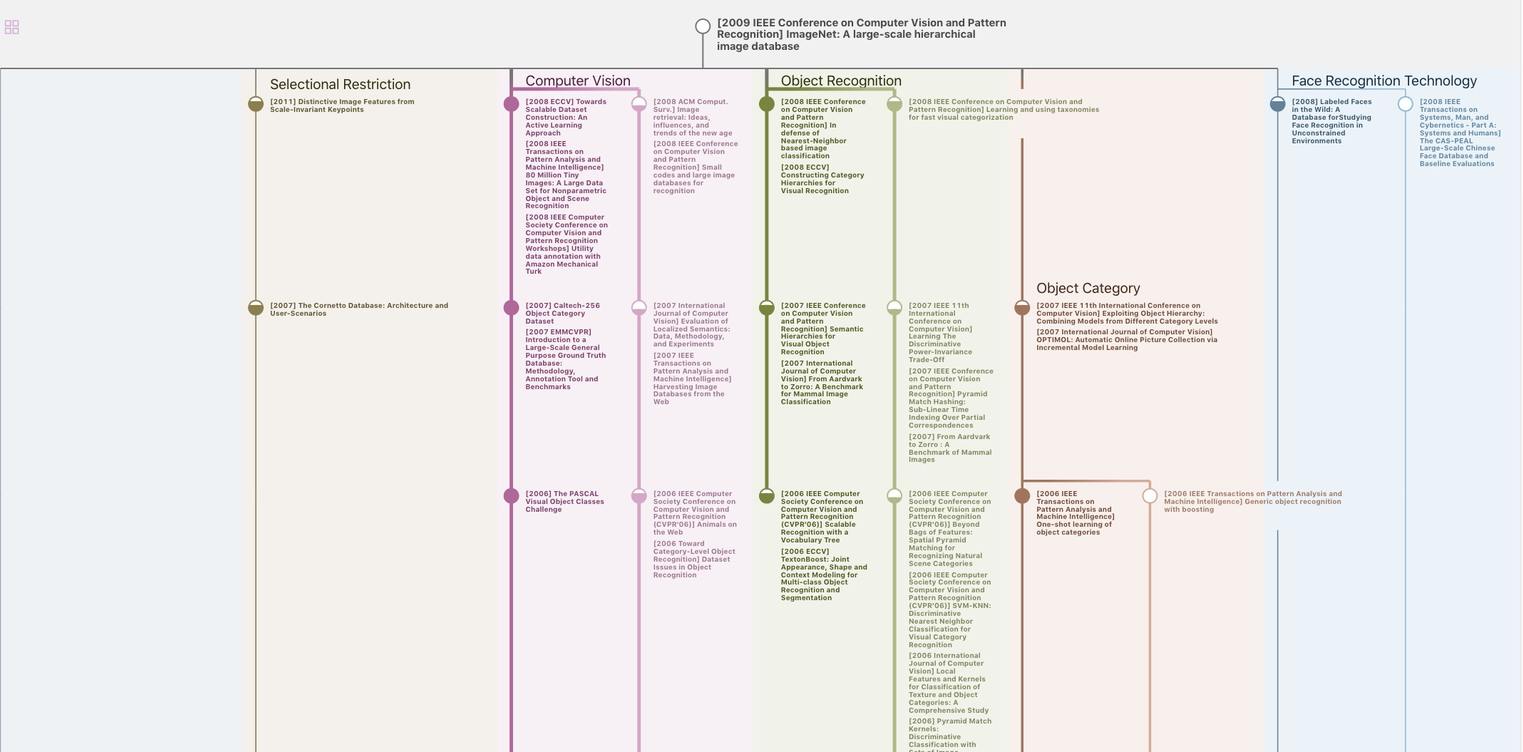
生成溯源树,研究论文发展脉络
Chat Paper
正在生成论文摘要