Corpus Synthesis for Zero-shot ASR domain Adaptation using Large Language Models
ICASSP 2024 - 2024 IEEE International Conference on Acoustics, Speech and Signal Processing (ICASSP)(2023)
摘要
While Automatic Speech Recognition (ASR) systems are widely used in many real-world applications, they often do not generalize well to new domains and need to be finetuned on data from these domains. However, target-domain data usually are not readily available in many scenarios. In this paper, we propose a new strategy for adapting ASR models to new target domains without any text or speech from those domains. To accomplish this, we propose a novel data synthesis pipeline that uses a Large Language Model (LLM) to generate a target domain text corpus, and a state-of-the-art controllable speech synthesis model to generate the corresponding speech. We propose a simple yet effective in-context instruction finetuning strategy to increase the effectiveness of LLM in generating text corpora for new domains. Experiments on the SLURP dataset show that the proposed method achieves an average relative word error rate improvement of $28\%$ on unseen target domains without any performance drop in source domains.
更多查看译文
关键词
corpus,adaptation,zero-shot
AI 理解论文
溯源树
样例
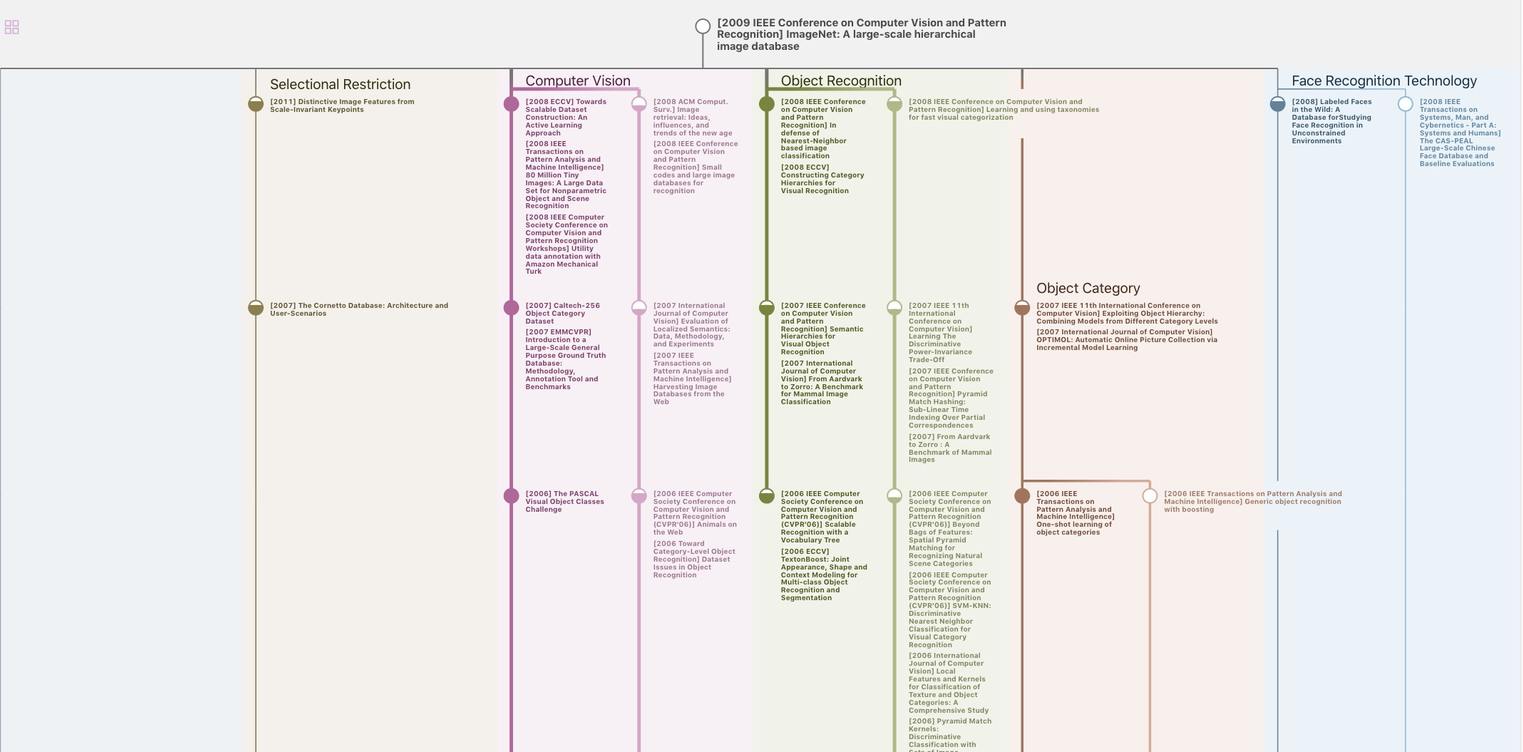
生成溯源树,研究论文发展脉络
Chat Paper
正在生成论文摘要