Online Fault Diagnosis of Harmonic Drives Using Semisupervised Contrastive Graph Generative Network via Multimodal Data
IEEE TRANSACTIONS ON INDUSTRIAL ELECTRONICS(2024)
摘要
Harmonic drive is a core component of the industrial robot, its failure will directly affect the robot's performance. Moreover, as the harmonic drive often works with excessive speed and load, it may fail unpredictably. Therefore, online fault diagnosis is quite significant. In this article, we propose an online intelligent fault diagnosis method for harmonic drives using a semisupervised contrastive graph generative network (SCGGN) via multimodal data. First, multimodal data (including motor current signals and encoder signals) of the harmonic drive are collected online. The Euclidean distance is used to analyze the similarity of the data in the frequency domain. Second, multiple graph convolution network and hierarchical graph convolution network are used to obtain complementary fault features from local and global views, respectively. Third, the contrastive learning network is constructed to obtain high-level information through unsupervised learning and perform data clustering to obtain the multiclassification output. Finally, a combination of learnable loss functions is used to optimize the SCGGN. The presented method is tested on an industrial robot. The experimental results show that the method can achieve 86.15% accuracy with 8% of the labeled training data and 79.9% accuracy with only 0.5% of the labeled training data, which are superior to the existing methods.
更多查看译文
关键词
Harmonic drives,multimodal data,online fault diagnosis,semisupervised contrastive graph generative network (SCGGN)
AI 理解论文
溯源树
样例
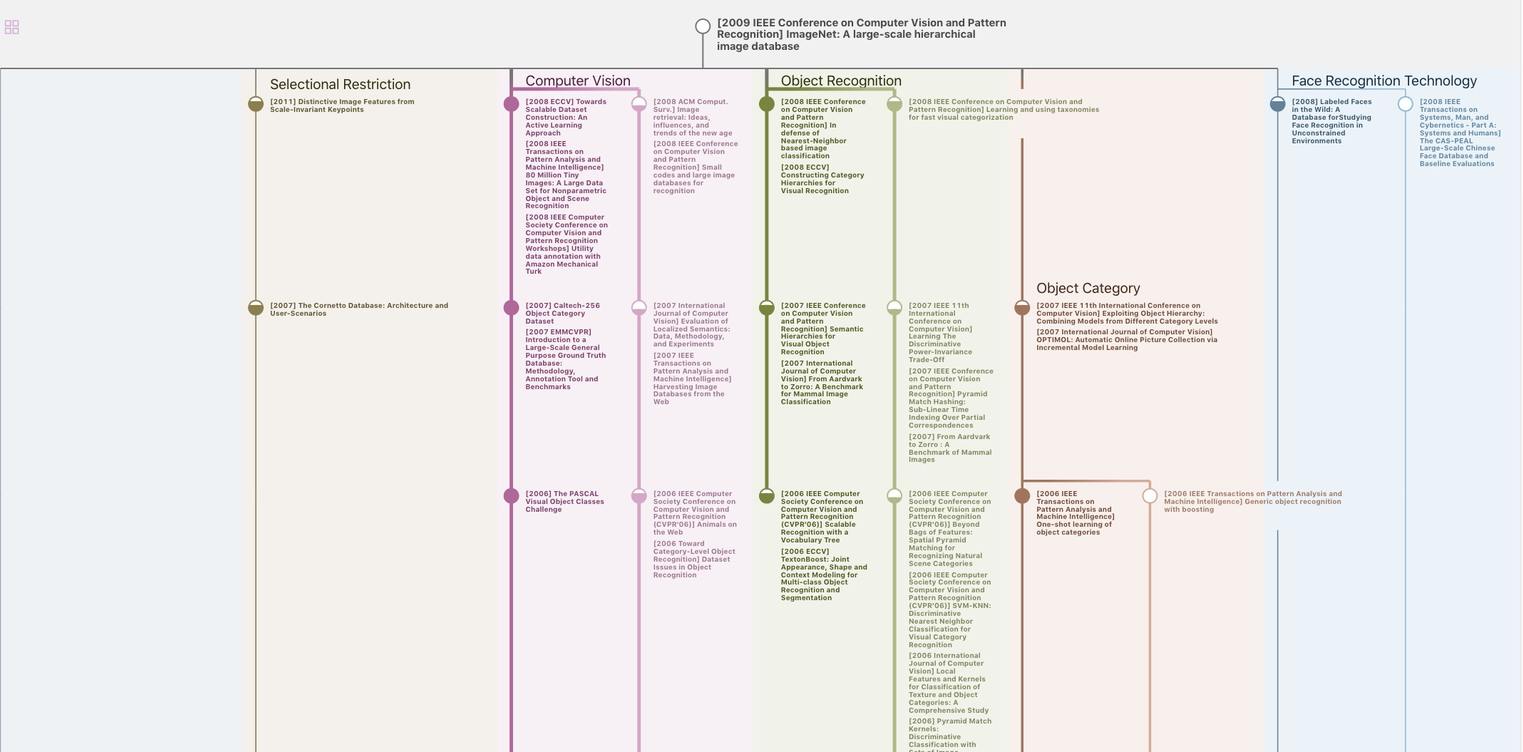
生成溯源树,研究论文发展脉络
Chat Paper
正在生成论文摘要