Dense 2D-3D Indoor Prediction with Sound via Aligned Cross-Modal Distillation
2023 IEEE/CVF International Conference on Computer Vision (ICCV)(2023)
摘要
Sound can convey significant information for spatial reasoning in our daily lives. To endow deep networks with such ability, we address the challenge of dense indoor prediction with sound in both 2D and 3D via cross-modal knowledge distillation. In this work, we propose a Spatial Alignment via Matching (SAM) distillation framework that elicits local correspondence between the two modalities in vision-to-audio knowledge transfer. SAM integrates audio features with visually coherent learnable spatial embeddings to resolve inconsistencies in multiple layers of a student model. Our approach does not rely on a specific input representation, allowing for flexibility in the input shapes or dimensions without performance degradation. With a newly curated benchmark named Dense Auditory Prediction of Surroundings (DAPS), we are the first to tackle dense indoor prediction of omnidirectional surroundings in both 2D and 3D with audio observations. Specifically, for audio-based depth estimation, semantic segmentation, and challenging 3D scene reconstruction, the proposed distillation framework consistently achieves state-of-the-art performance across various metrics and backbone architectures.
更多查看译文
关键词
indoor prediction,cross-modal
AI 理解论文
溯源树
样例
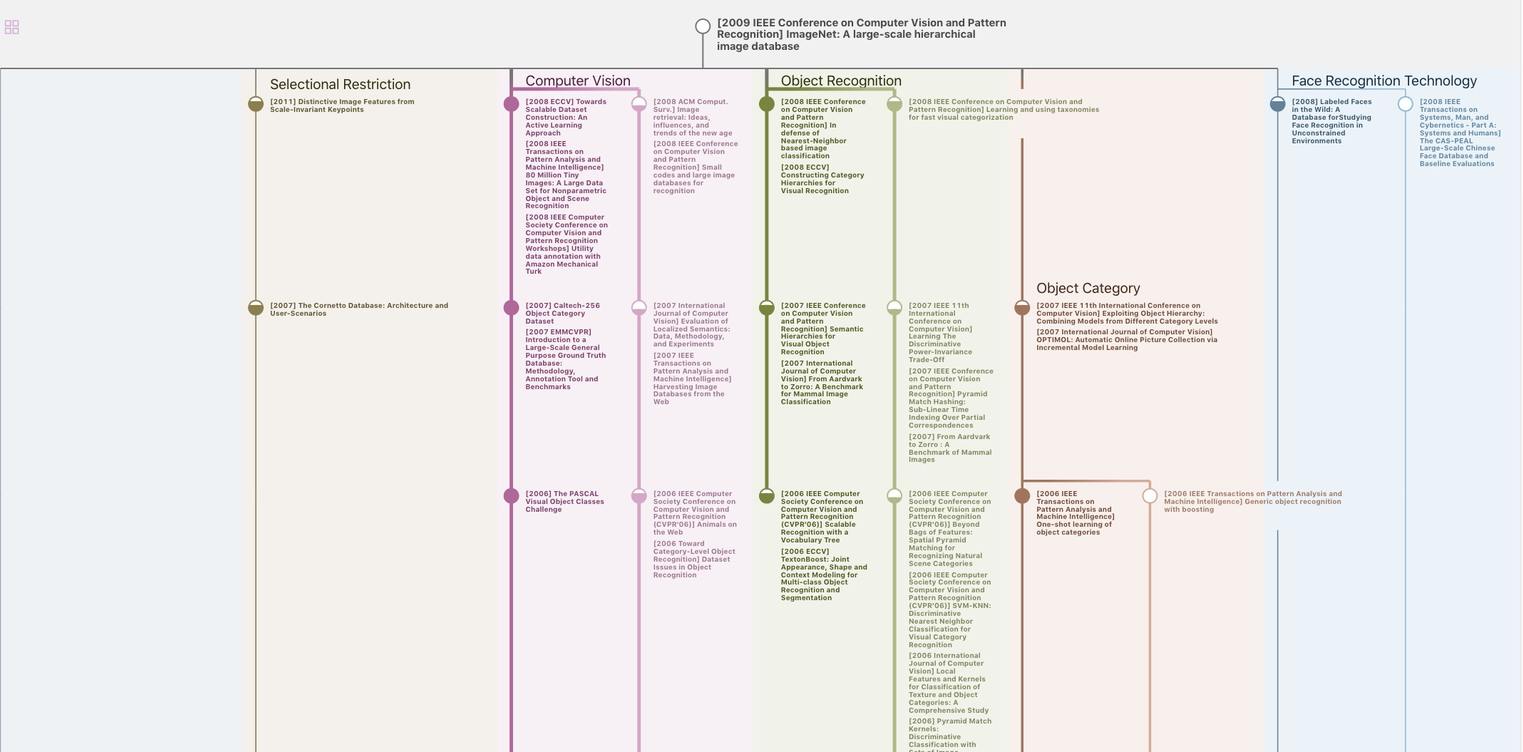
生成溯源树,研究论文发展脉络
Chat Paper
正在生成论文摘要