Optimize-via-Predict: Realizing out-of-sample optimality in data-driven optimization
arXiv (Cornell University)(2023)
摘要
We examine a stochastic formulation for data-driven optimization wherein the decision-maker is not privy to the true distribution, but has knowledge that it lies in some hypothesis set and possesses a historical data set, from which information about it can be gleaned. We define a prescriptive solution as a decision rule mapping such a data set to decisions. As there does not exist prescriptive solutions that are generalizable over the entire hypothesis set, we define out-of-sample optimality as a local average over a neighbourhood of hypotheses, and averaged over the sampling distribution. We prove sufficient conditions for local out-of-sample optimality, which reduces to functions of the sufficient statistic of the hypothesis family. We present an optimization problem that would solve for such an out-of-sample optimal solution, and does so efficiently by a combination of sampling and bisection search algorithms. Finally, we illustrate our model on the newsvendor model, and find strong performance when compared against alternatives in the literature. There are potential implications of our research on end-to-end learning and Bayesian optimization.
更多查看译文
关键词
optimization,optimize-via-predict,out-of-sample,data-driven
AI 理解论文
溯源树
样例
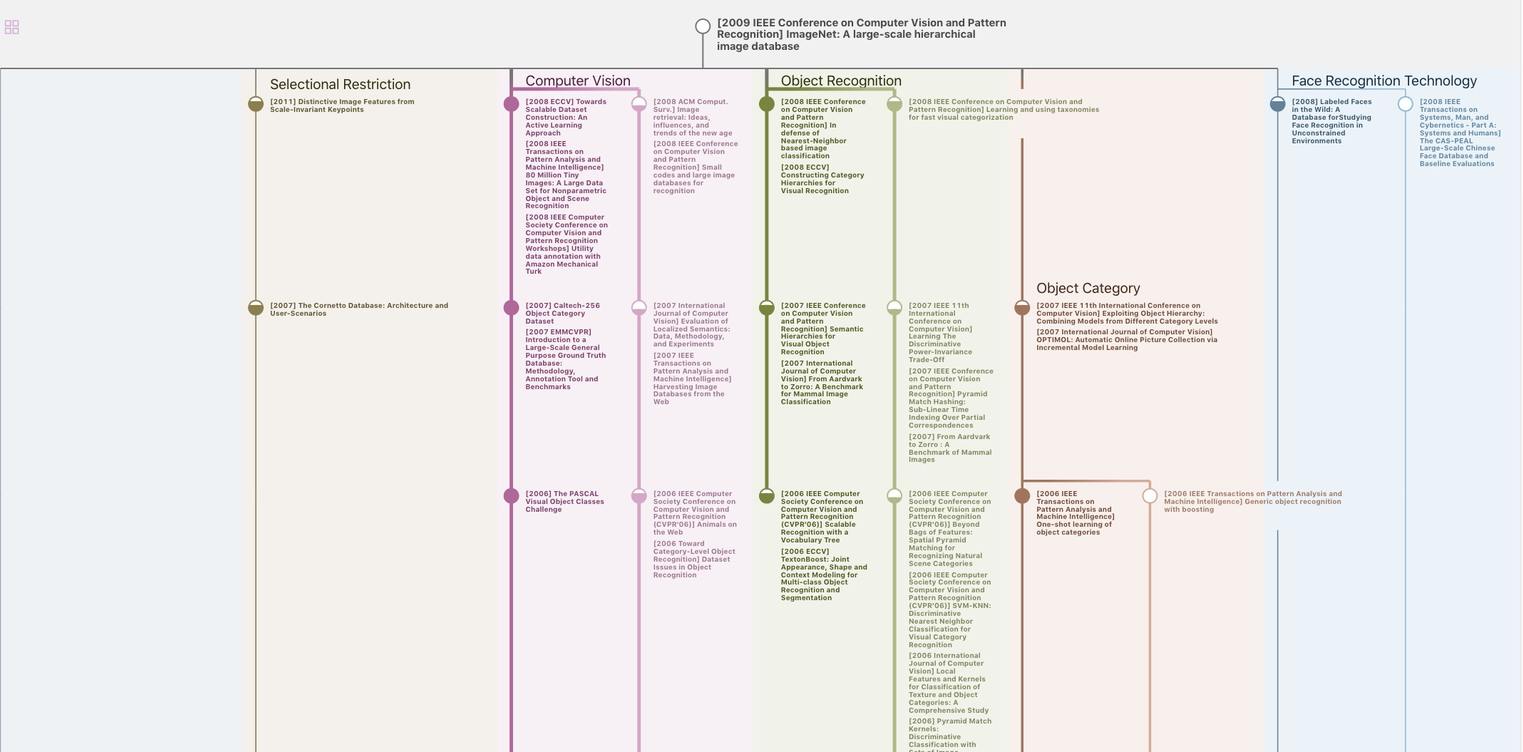
生成溯源树,研究论文发展脉络
Chat Paper
正在生成论文摘要