Language-driven Object Fusion into Neural Radiance Fields with Pose-Conditioned Dataset Updates
CVPR 2024(2024)
The Hong Kong University of Science and Technology | Hong Kong University of Science and Technology | Trinity College Dublin | Deakin University | The Hong Kong University of Science and Technology (HKUST
Abstract
Neural radiance field (NeRF) is an emerging technique for 3D scene reconstruction and modeling. However, current NeRF-based methods are limited in the capabilities of adding or removing objects. This paper fills the aforementioned gap by proposing a new language-driven method for object manipulation in NeRFs through dataset updates. Specifically, to insert an object represented by a set of multi-view images into a background NeRF, we use a text-to-image diffusion model to blend the object into the given background across views. The generated images are then used to update the NeRF so that we can render view-consistent images of the object within the background. To ensure view consistency, we propose a dataset update strategy that prioritizes the radiance field training based on camera poses in a pose-ordered manner. We validate our method in two case studies: object insertion and object removal. Experimental results show that our method can generate photo-realistic results and achieves state-of-the-art performance in NeRF editing.
MoreTranslated text
Key words
Radiance Field,Neural Radiance Fields,Image Object,Diffusion Model,3D Scene,Camera Pose,Multi-view Images,Convolutional Neural Network,Generative Adversarial Networks,Target Object,Background Image,Image Synthesis,Sneakers,Insertion Method,Image Inpainting,3D Bounding Box,Traditional Pipeline,Traditional 3D,Masked Area
PDF
View via Publisher
AI Read Science
AI Summary
AI Summary is the key point extracted automatically understanding the full text of the paper, including the background, methods, results, conclusions, icons and other key content, so that you can get the outline of the paper at a glance.
Example
Background
Key content
Introduction
Methods
Results
Related work
Fund
Key content
- Pretraining has recently greatly promoted the development of natural language processing (NLP)
- We show that M6 outperforms the baselines in multimodal downstream tasks, and the large M6 with 10 parameters can reach a better performance
- We propose a method called M6 that is able to process information of multiple modalities and perform both single-modal and cross-modal understanding and generation
- The model is scaled to large model with 10 billion parameters with sophisticated deployment, and the 10 -parameter M6-large is the largest pretrained model in Chinese
- Experimental results show that our proposed M6 outperforms the baseline in a number of downstream tasks concerning both single modality and multiple modalities We will continue the pretraining of extremely large models by increasing data to explore the limit of its performance
Try using models to generate summary,it takes about 60s
Must-Reading Tree
Example
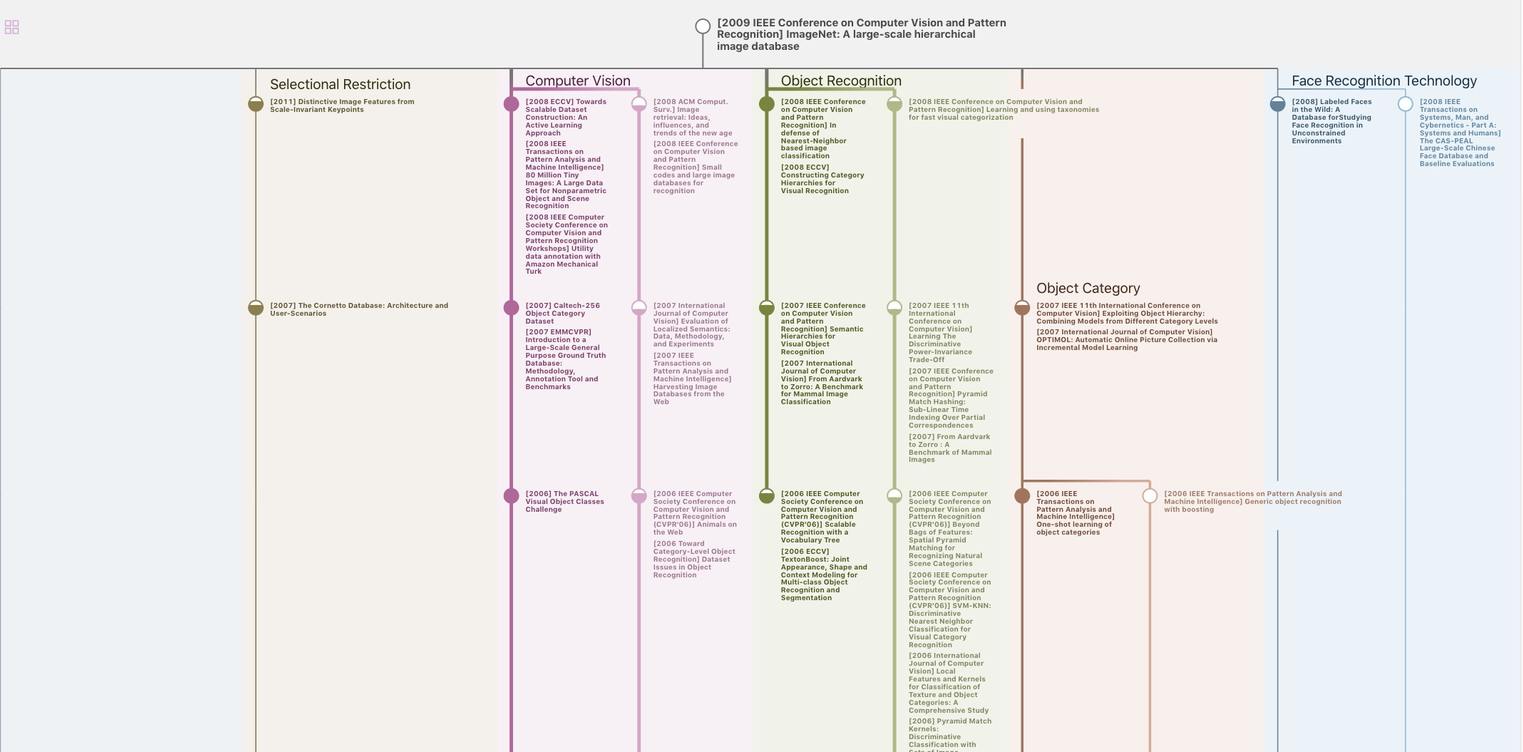
Generate MRT to find the research sequence of this paper
Related Papers
Consolidating Attention Features for Multi-view Image Editing
ACM SIGGRAPH Conference and Exhibition on Computer Graphics and Interactive Techniques in Asia 2024
被引用0
Data Disclaimer
The page data are from open Internet sources, cooperative publishers and automatic analysis results through AI technology. We do not make any commitments and guarantees for the validity, accuracy, correctness, reliability, completeness and timeliness of the page data. If you have any questions, please contact us by email: report@aminer.cn
Chat Paper
去 AI 文献库 对话