Reconstruction of subsurface ocean state variables using Convolutional Neural Networks with combined satellite and in situ data
Frontiers in Marine Science(2023)
摘要
Subsurface ocean measurements are extremely sparse and irregularly distributed, narrowing our ability to describe deep ocean processes and thus also limiting our understanding of the role of ocean and marine ecosystems in the Earth system. To overcome these observational limitations, neural networks combining remotely-sensed surface measurements and in situ vertical profiles are increasingly being used to retrieve high-quality three-dimensional estimates of the ocean state. This study proposes a convolutional neural network (CNN) architecture for the reconstruction of vertical profiles of temperature and salinity starting from surface observation-based data. The model is trained on satellite and in situ data collected between 2005 and 2020 in the Atlantic Ocean. Rather than using spatially gridded in situ observations, we use directly measured vertical profiles. Different combinations of surface variables are analyzed and compared in order to determine the most effective inputs for the CNN. Furthermore, the relative importance of each of these variables in the vertical reconstruction is assessed using Shapley values, originally developed in the framework of cooperative game theory. The model performance is shown to be superior to current state-of-the-art methods and the same approach can easily be extended to other basins or to the global ocean.
更多查看译文
关键词
3D reconstruction,remote sensing,Convolutional Neural Networks,sea surface temperature,sea surface salinity,earth observation
AI 理解论文
溯源树
样例
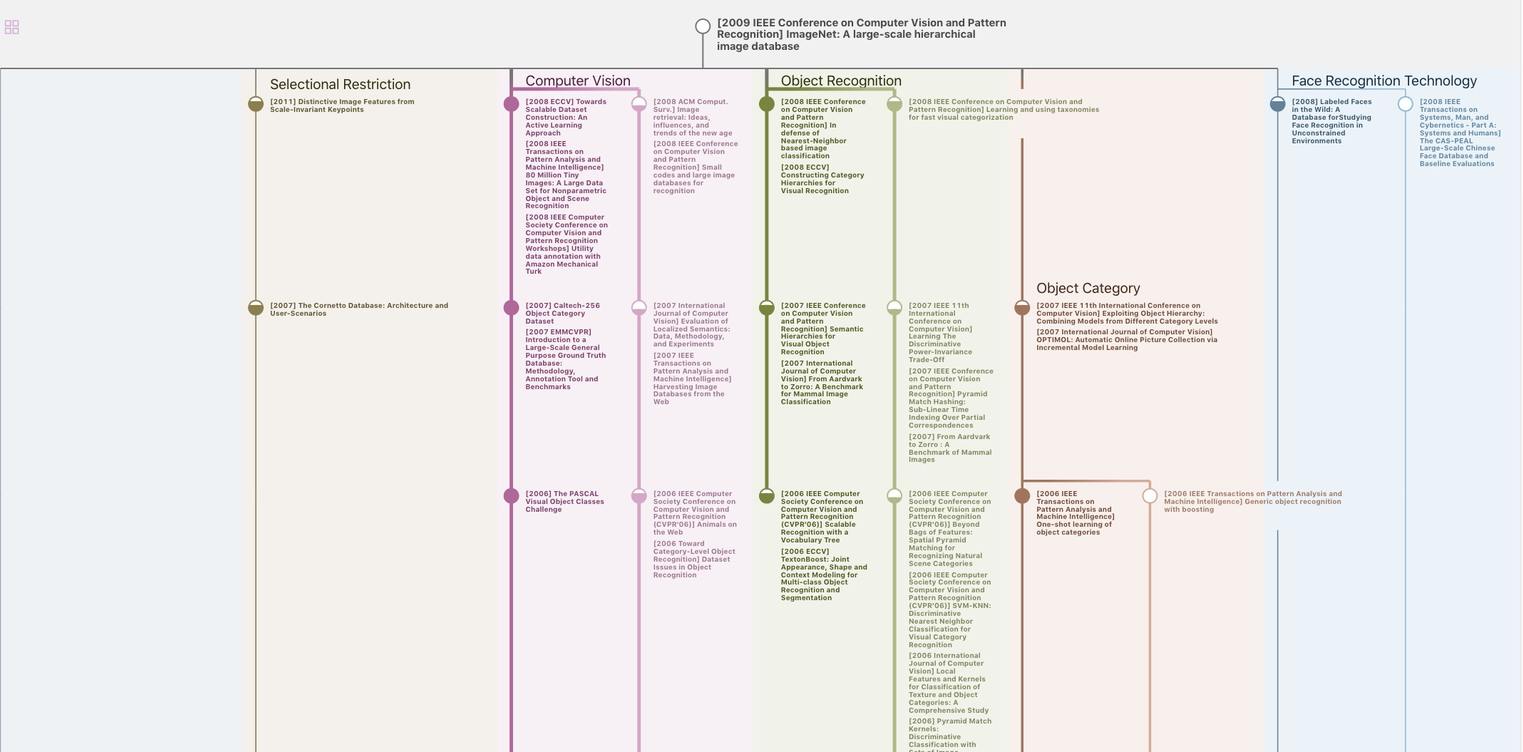
生成溯源树,研究论文发展脉络
Chat Paper
正在生成论文摘要