Identification technique of cryptomining behavior based on traffic features
Frontiers in Physics(2023)
摘要
Recently, the growth of blockchain technology and the economic benefits of cryptocurrencies have led to a proliferation of malicious cryptomining activities on the internet, resulting in significant losses for companies and institutions. Therefore, accurately detecting and identifying these behaviors has become essential. To address low accuracy in detecting and identifying cryptomining behaviors in encrypted traffic, a technique for identifying cryptomining behavior traffic is proposed. This technique is based on the time series characteristics of network traffic and introduces the feature of long-range dependence, and the recognition effect is not easily affected by the encryption algorithm. First, 48-dimensional features are extracted from the network traffic using statistical methods and the rescaled range method, of which 47 dimensions are statistical features and 1 dimension is a long-range dependence feature. Second, because there is much less cryptomining traffic information than normal network traffic information in the dataset, the dataset is processed using oversampling to make the two types of traffic data balanced. Finally, a random forest model is used to identify the type of traffic based on its features. Experiments demonstrate that this approach achieves good detection performance and provides an effective solution for identifying encrypted network traffic with malicious cryptomining behavior. The long-range dependence features introduced therein together with the statistical features describe a more comprehensive flow characteristics, and the preprocessing of the dataset improves the performance of the identification model.
更多查看译文
关键词
traffic features
AI 理解论文
溯源树
样例
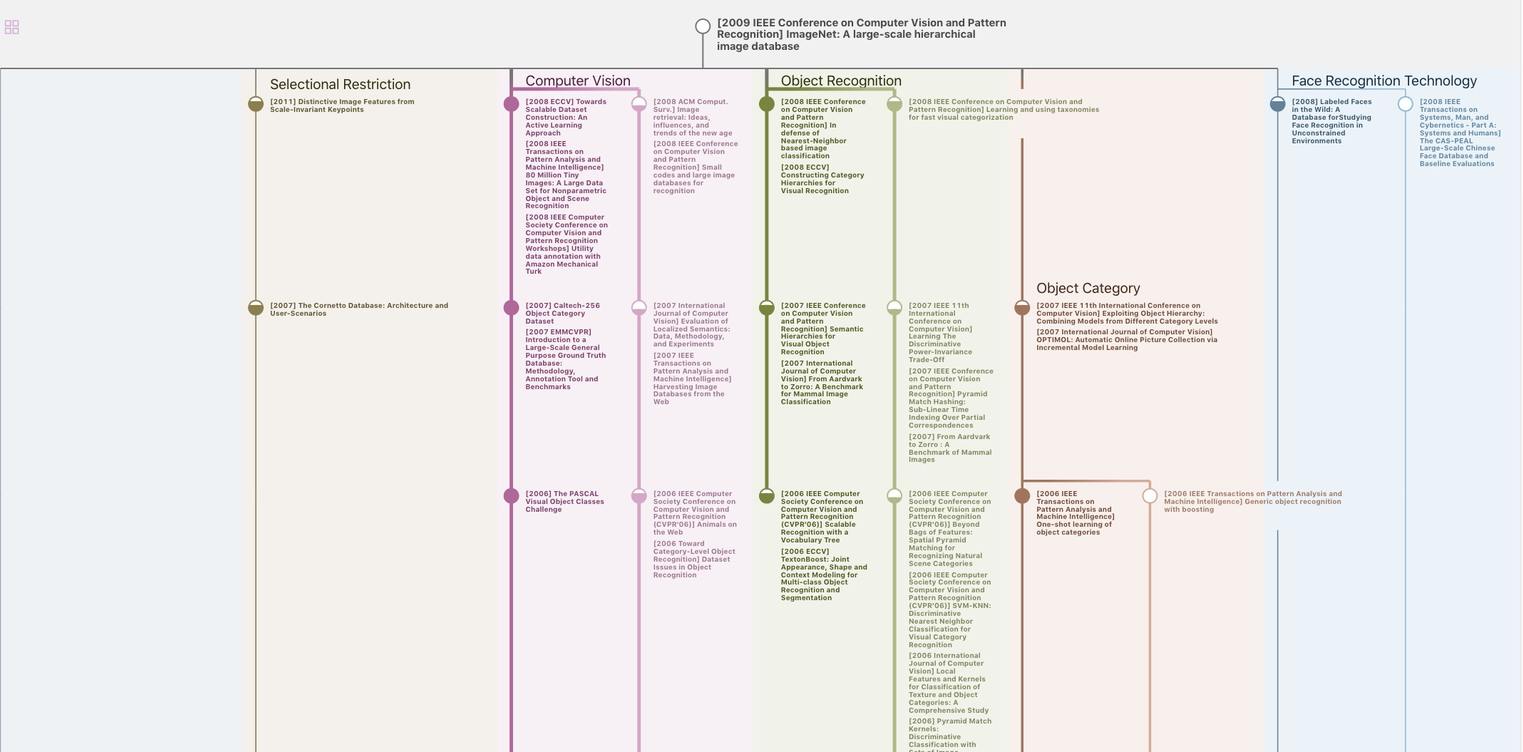
生成溯源树,研究论文发展脉络
Chat Paper
正在生成论文摘要