Multi-Branch ResNet-Transformer for Short-Term Spatio-Temporal Solar Irradiance Forecasting
IEEE Transactions on Industry Applications(2023)
摘要
The increasing penetration of solar generation into power grids has promoted the need for accurate and reliable short-term solar irradiance forecasting. Existing methods utilizing advanced deep learning architectures have shown advanced performance compared to conventional time-series analytical techniques but in general encountered shortcomings in modeling spatial correlations among neighboring solar generation sites, exploring the similarity of long-term, time-varying patterns, and alleviating overfitting issues in convolutional and recurrent neural networks, such as the popular Long Short-term Memory (LSTM). To effectively but yet reliably tackle these challenges in the existing literature, this article proposes a spatio-temporal framework consisting of a multi-branch hybrid Residual network and the
Transformer
architecture (ResTrans). The proposed framework has been tested on two groups' real-world data containing 17 years-long data from different solar sites in Philadelphia, USA, including 12 and 18 locations, respectively. Compared to other hybrid benchmark architectures, including single-branch ResTrans and multi-branch ResNet-LSTM (ResLSTM), single-branch ResLSTM, and CNN-LSTM, the proposed multi-branch ResTrans achieves the highest forecasting accuracy with an average RMSE of 0.049 (W/m
2
), an average MAE of 0.031 (W/m
2
), and an
$R^{2}$
coefficient of 97%.
更多查看译文
关键词
Solar irradiance forecasting,spatio-temporal modeling,deep residual network (ResNet),attention mechanism,transformer neural network
AI 理解论文
溯源树
样例
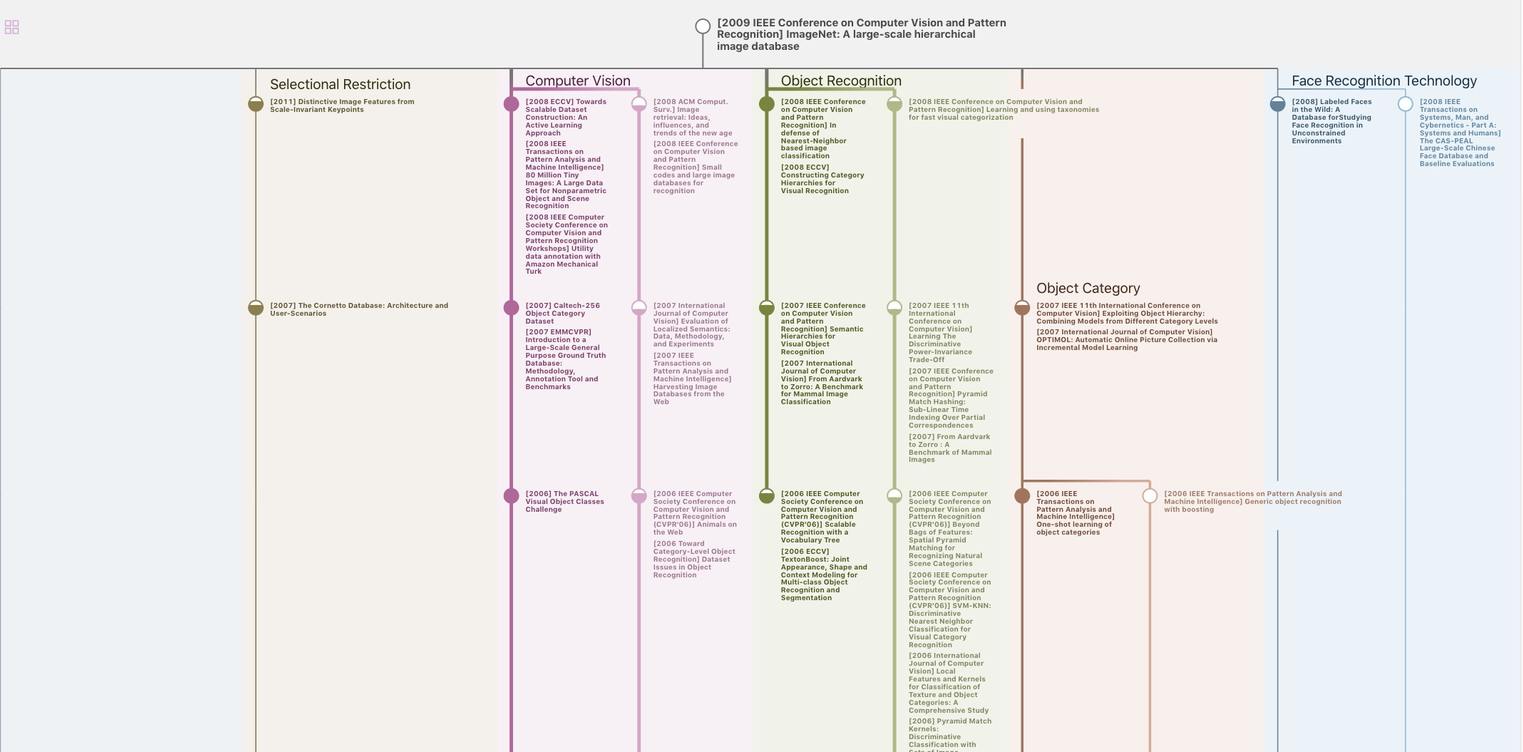
生成溯源树,研究论文发展脉络
Chat Paper
正在生成论文摘要