Reinforcement Learning with Goal Relabeling and Dynamic Model for Robotic Tasks
2023 IEEE International Conference on Real-time Computing and Robotics (RCAR)(2023)
摘要
Improving sample efficiency is crucial for reinforcement learning, especially for the robot manipulation tasks. The model-based method could improve the sample efficiency by introducing a dynamic model to generate a large number of samples, so that the requirement of interaction with the environment can be reduced. However, as the dynamic model is constructed by the deep network, the model error is inevitable. This error will increase the errors of the data generated by the model, which may damage the policy training process to a certain extent. To overcome the limitations of the above data augmentation methods, this paper proposes a new reinforcement learning method based on goal relabeling and dynamic (GMRL) model. In the GMRL, the quality of the explored data will be improved by the goal relabeling at first, followed by introducing a dynamic model to further increase the data quantity. The proposed method has been tested in a reinforcement learning benchmark environment, and the results show that the performance of the proposed method is significantly better than that both of the goal relabeling and standard model-based methods. At the same time, the proposed method has a higher sample efficiency than other existing combined schema of goal relabeling and dynamic model.
更多查看译文
AI 理解论文
溯源树
样例
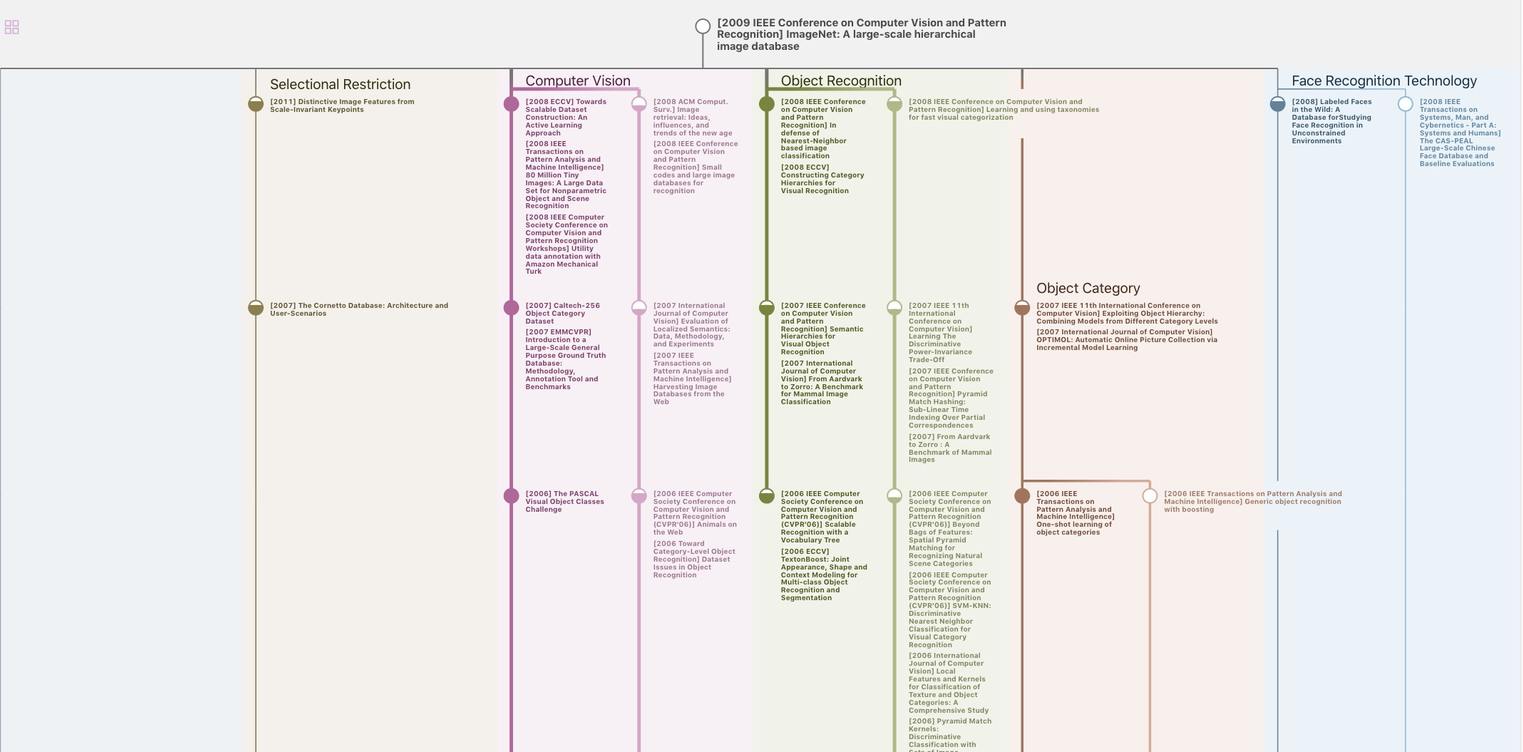
生成溯源树,研究论文发展脉络
Chat Paper
正在生成论文摘要