Ad-load Balancing via Off-policy Learning in a Content Marketplace
PROCEEDINGS OF THE 17TH ACM INTERNATIONAL CONFERENCE ON WEB SEARCH AND DATA MINING, WSDM 2024(2024)
摘要
Ad-load balancing is a critical challenge in online advertising systems, particularly in the context of social media platforms, where the goal is to maximize user engagement and revenue while maintaining a satisfactory user experience. This requires the optimization of conflicting objectives, such as user satisfaction and ads revenue. Traditional approaches to ad-load balancing rely on static allocation policies, which fail to adapt to changing user preferences and contextual factors. In this paper, we present an approach that leverages off-policy learning and evaluation from logged bandit feedback. We start by presenting a motivating analysis of the ad-load balancing problem, highlighting the conflicting objectives between user satisfaction and ads revenue. We emphasize the nuances that arise due to user heterogeneity and the dependence on the user's position within a session. Based on this analysis, we define the problem as determining the optimal ad-load for a particular feed fetch. To tackle this problem, we propose an off-policy learning framework that leverages unbiased estimators such as Inverse Propensity Scoring (IPS) and Doubly Robust (DR) to learn and estimate the policy values using offline collected stochastic data. We present insights from online A/B experiments deployed at scale across over 80 million users generating over 200 million sessions, where we find statistically significant improvements in both user satisfaction metrics and ads revenue for the platform.
更多查看译文
关键词
Online advertising,Inverse Propensity Scoring,A/B-testing
AI 理解论文
溯源树
样例
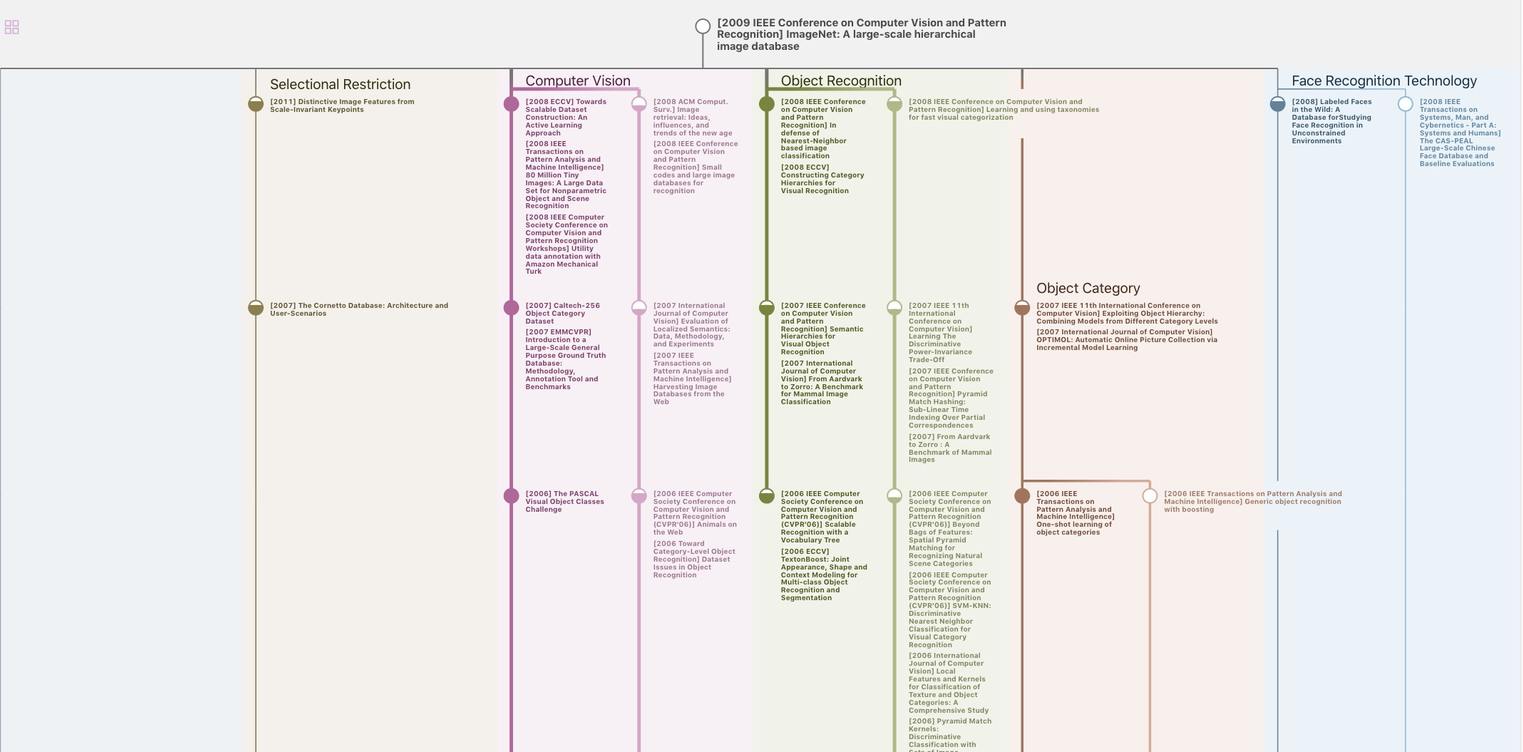
生成溯源树,研究论文发展脉络
Chat Paper
正在生成论文摘要