Incorporating Singletons and Mention-based Features in Coreference Resolution via Multi-task Learning for Better Generalization
Proceedings of the 13th International Joint Conference on Natural Language Processing and the 3rd Conference of the Asia-Pacific Chapter of the Association for Computational Linguistics (Volume 2: Short Papers)(2023)
摘要
Previous attempts to incorporate a mention detection step into end-to-end neural coreference resolution for English have been hampered by the lack of singleton mention span data as well as other entity information. This paper presents a coreference model that learns singletons as well as features such as entity type and information status via a multi-task learning-based approach. This approach achieves new state-of-the-art scores on the OntoGUM benchmark (+2.7 points) and increases robustness on multiple out-of-domain datasets (+2.3 points on average), likely due to greater generalizability for mention detection and utilization of more data from singletons when compared to only coreferent mention pair matching.
更多查看译文
关键词
coreference,generalization,mention-based,multi-task
AI 理解论文
溯源树
样例
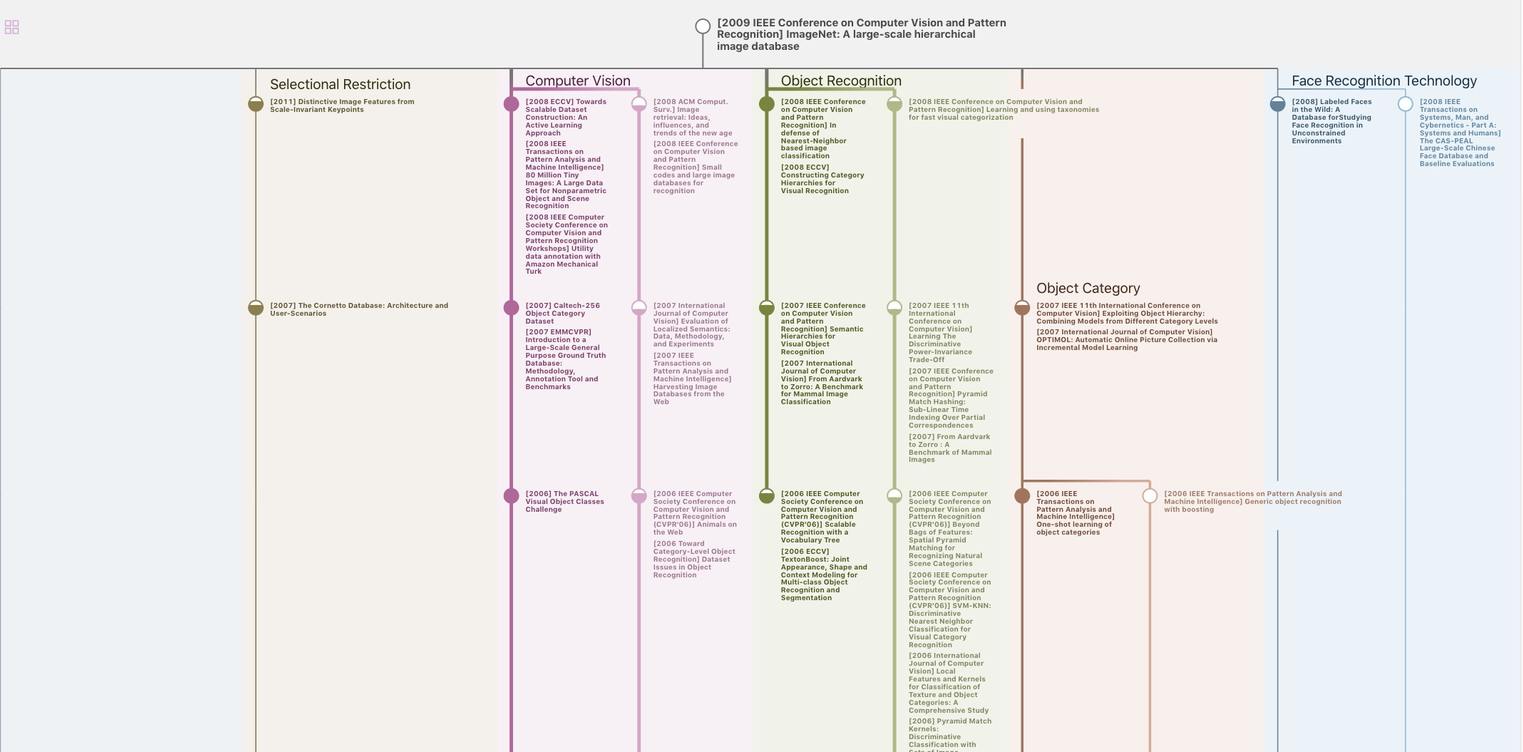
生成溯源树,研究论文发展脉络
Chat Paper
正在生成论文摘要