Leveraging In-the-Wild Data for Effective Self-Supervised Pretraining in Speaker Recognition
ICASSP 2024 - 2024 IEEE International Conference on Acoustics, Speech and Signal Processing (ICASSP)(2023)
摘要
Current speaker recognition systems primarily rely on supervised approaches, constrained by the scale of labeled datasets. To boost the system performance, researchers leverage large pretrained models such as WavLM to transfer learned high-level features to the downstream speaker recognition task. However, this approach introduces extra parameters as the pretrained model remains in the inference stage. Another group of researchers directly apply self-supervised methods such as DINO to speaker embedding learning, yet they have not explored its potential on large-scale in-the-wild datasets. In this paper, we present the effectiveness of DINO training on the large-scale WenetSpeech dataset and its transferability in enhancing the supervised system performance on the CNCeleb dataset. Additionally, we introduce a confidence-based data filtering algorithm to remove unreliable data from the pretraining dataset, leading to better performance with less training data. The associated pretrained models, confidence files, pretraining and finetuning scripts will be made available in the Wespeaker toolkit.
更多查看译文
关键词
self-supervised learning,DINO,in-the-wild,speaker recognition
AI 理解论文
溯源树
样例
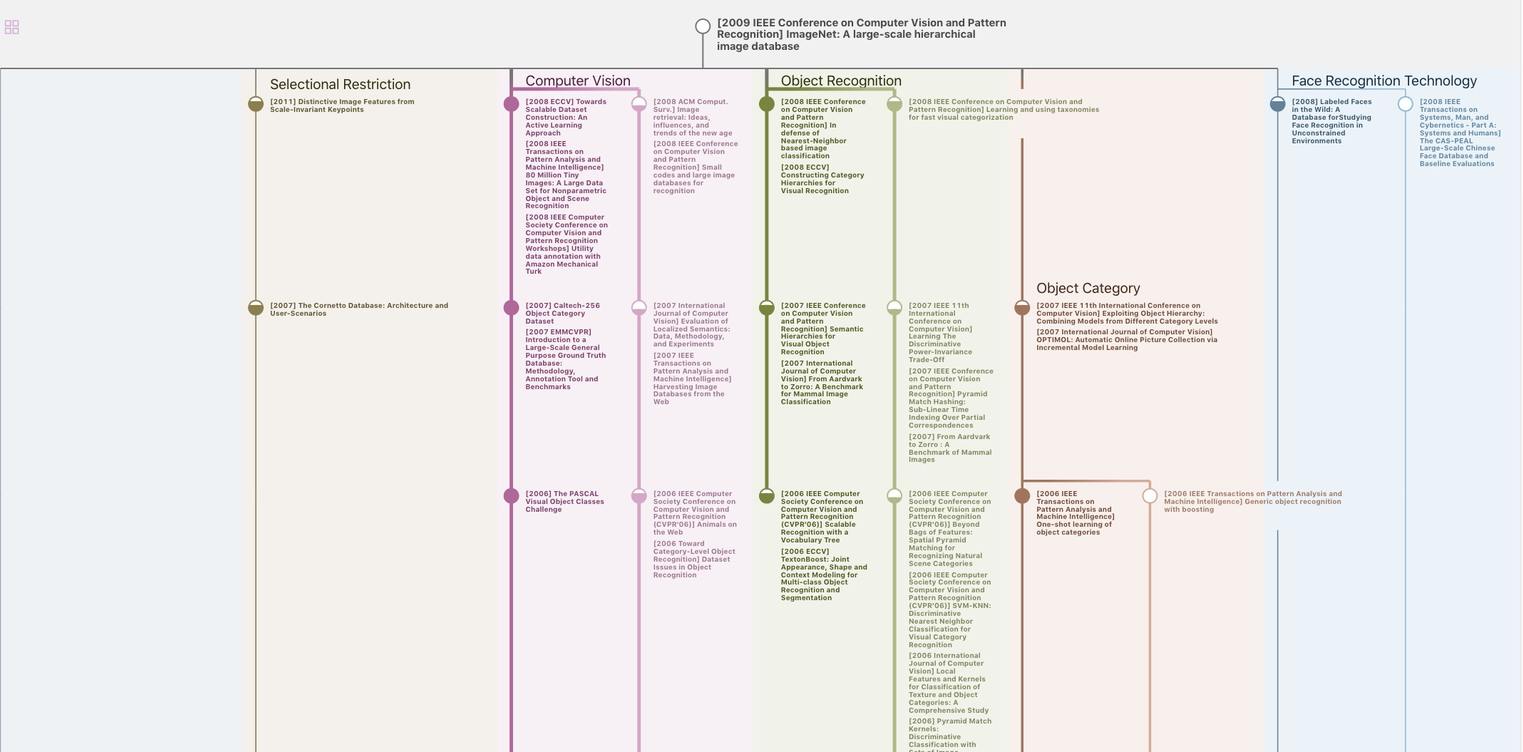
生成溯源树,研究论文发展脉络
Chat Paper
正在生成论文摘要