Evaluating Large Language Models for Document-grounded Response Generation in Information-Seeking Dialogues
CoRR(2023)
摘要
In this paper, we investigate the use of large language models (LLMs) like ChatGPT for document-grounded response generation in the context of information-seeking dialogues. For evaluation, we use the MultiDoc2Dial corpus of task-oriented dialogues in four social service domains previously used in the DialDoc 2022 Shared Task. Information-seeking dialogue turns are grounded in multiple documents providing relevant information. We generate dialogue completion responses by prompting a ChatGPT model, using two methods: Chat-Completion and LlamaIndex. ChatCompletion uses knowledge from ChatGPT model pretraining while LlamaIndex also extracts relevant information from documents. Observing that document-grounded response generation via LLMs cannot be adequately assessed by automatic evaluation metrics as they are significantly more verbose, we perform a human evaluation where annotators rate the output of the shared task winning system, the two Chat-GPT variants outputs, and human responses. While both ChatGPT variants are more likely to include information not present in the relevant segments, possibly including a presence of hallucinations, they are rated higher than both the shared task winning system and human responses.
更多查看译文
关键词
large language models,response
AI 理解论文
溯源树
样例
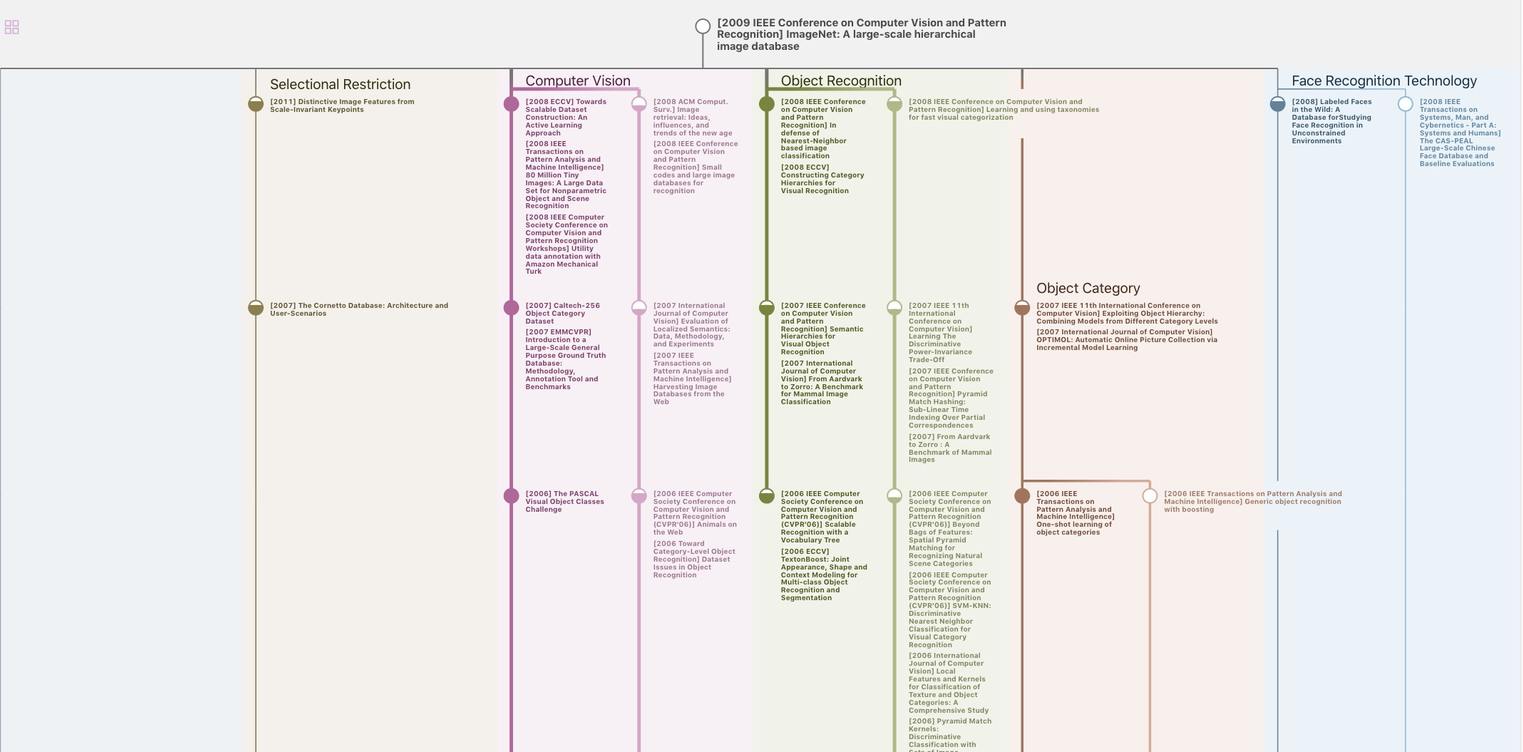
生成溯源树,研究论文发展脉络
Chat Paper
正在生成论文摘要