Inferring Capabilities from Task Performance with Bayesian Triangulation
CoRR(2023)
摘要
As machine learning models become more general, we need to characterise them in richer, more meaningful ways. We describe a method to infer the cognitive profile of a system from diverse experimental data. To do so, we introduce measurement layouts that model how task-instance features interact with system capabilities to affect performance. These features must be triangulated in complex ways to be able to infer capabilities from non-populational data -- a challenge for traditional psychometric and inferential tools. Using the Bayesian probabilistic programming library PyMC, we infer different cognitive profiles for agents in two scenarios: 68 actual contestants in the AnimalAI Olympics and 30 synthetic agents for O-PIAAGETS, an object permanence battery. We showcase the potential for capability-oriented evaluation.
更多查看译文
关键词
bayesian triangulation,task performance,capabilities
AI 理解论文
溯源树
样例
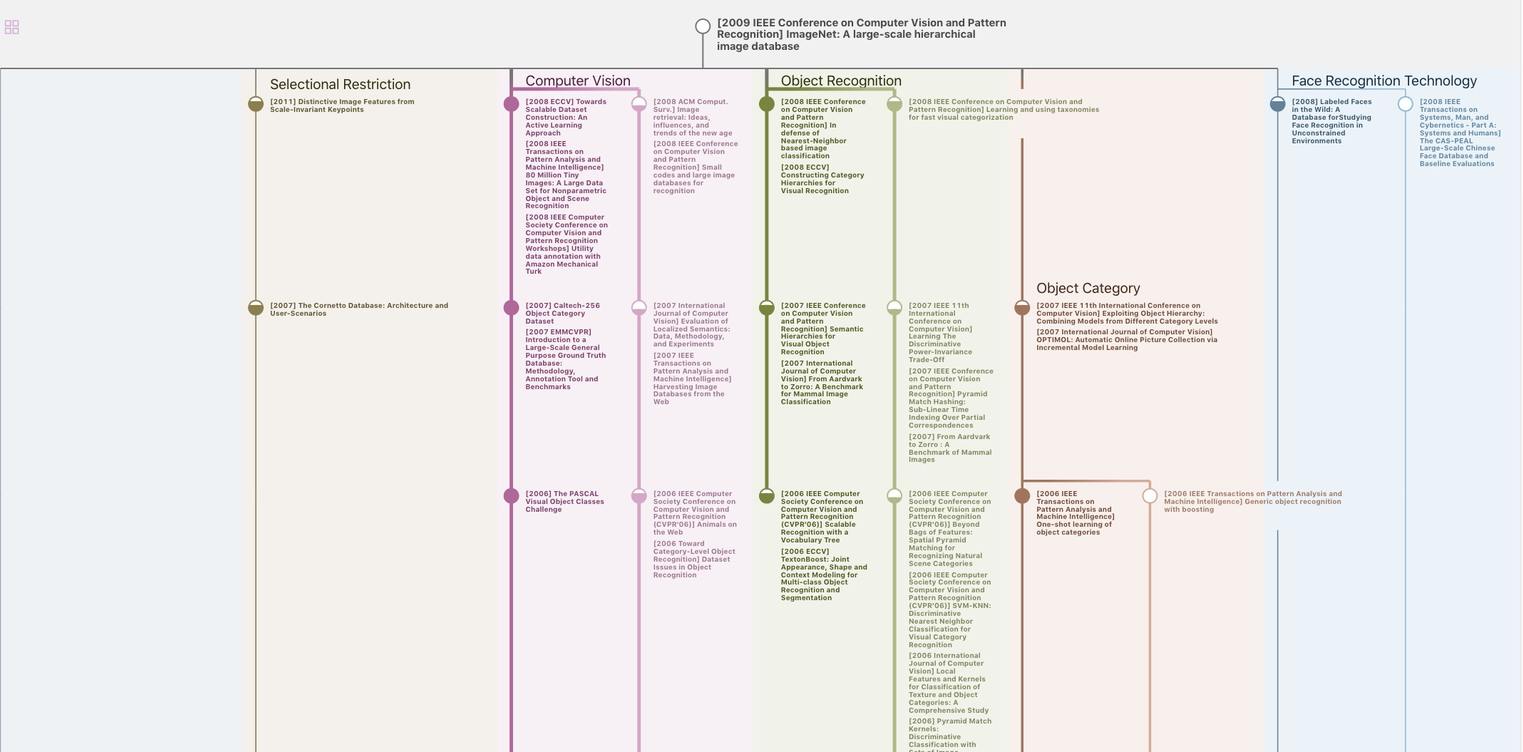
生成溯源树,研究论文发展脉络
Chat Paper
正在生成论文摘要