Environment-biased Feature Ranking for Novelty Detection Robustness
CoRR(2023)
摘要
We tackle the problem of robust novelty detection, where we aim to detect novelties in terms of semantic content while being invariant to changes in other, irrelevant factors. Specifically, we operate in a setup with multiple environments, where we determine the set of features that are associated more with the environments, rather than to the content relevant for the task. Thus, we propose a method that starts with a pretrained embedding and a multi-env setup and manages to rank the features based on their environment-focus. First, we compute a per-feature score based on the feature distribution variance between envs. Next, we show that by dropping the highly scored ones, we manage to remove spurious correlations and improve the overall performance by up to 6%, both in covariance and sub-population shift cases, both for a real and a synthetic benchmark, that we introduce for this task.
更多查看译文
关键词
novelty detection robustness,feature ranking,environment-biased
AI 理解论文
溯源树
样例
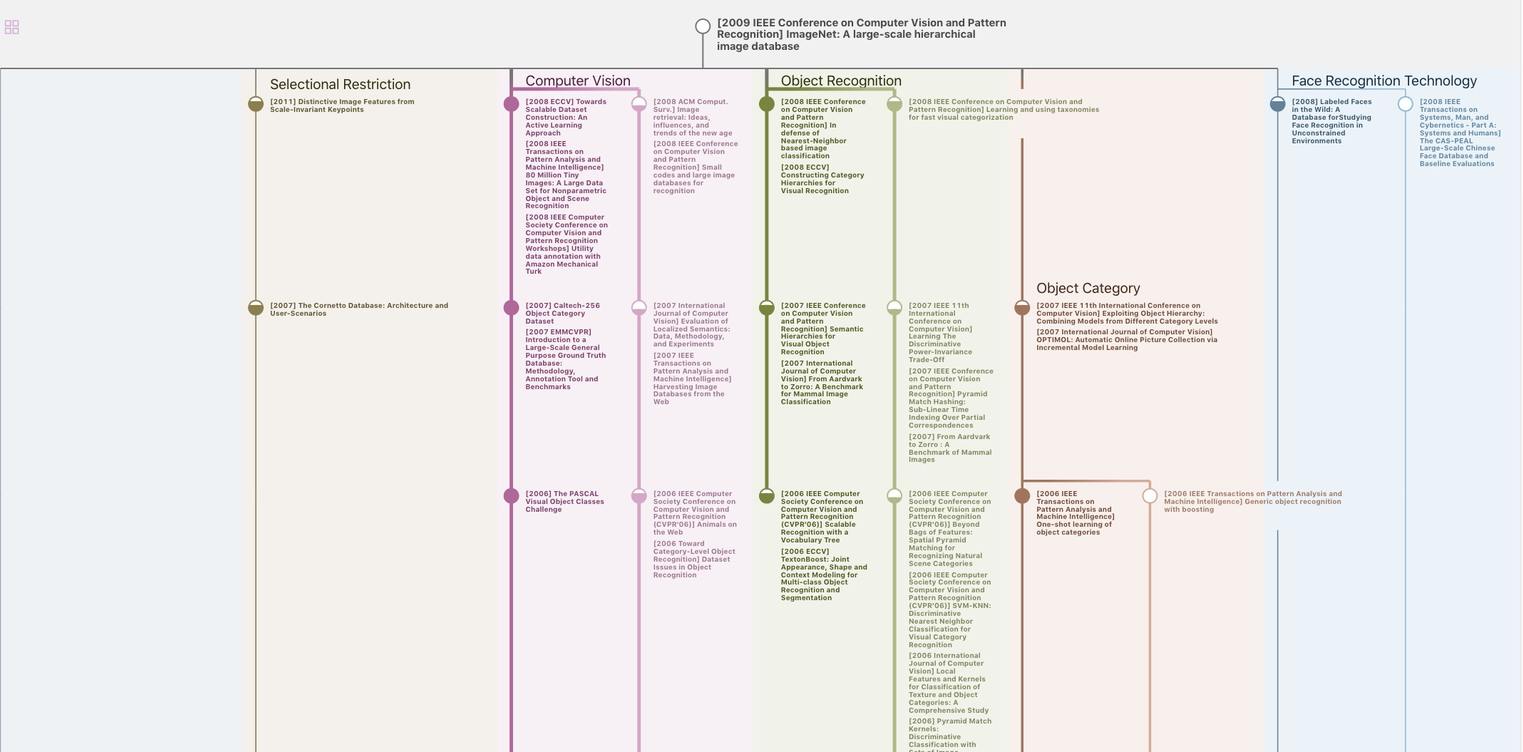
生成溯源树,研究论文发展脉络
Chat Paper
正在生成论文摘要