Koopmans' Theorem-Compliant Long-Range Corrected (KTLC) Density Functional Mediated by Black-Box Optimization and Data-Driven Prediction for Organic Molecules
JOURNAL OF CHEMICAL THEORY AND COMPUTATION(2023)
摘要
Density functional theory (DFT) is a significant computational tool that has substantially influenced chemistry, physics, and materials science. DFT necessitates parametrized approximation for determining an expected value. Hence, to predict the properties of a given molecule using DFT, appropriate parameters of the functional should be set for each molecule. Herein, we optimize the parameters of range-separated functionals (LC-BLYP and CAM-B3LYP) via Bayesian optimization (BO) to satisfy Koopmans' theorem. Our results demonstrate the effectiveness of the BO in optimizing functional parameters. Particularly, Koopmans' theorem-compliant LC-BLYP (KTLC-BLYP) shows results comparable to the experimental UV-absorption values. Furthermore, we prepared an optimized parameter dataset of KTLC-BLYP for over 3000 molecules through BO for satisfying Koopmans' theorem. We have developed a machine learning model on this dataset to predict the parameters of the LC-BLYP functional for a given molecule. The prediction model automatically predicts the appropriate parameters for a given molecule and calculates the corresponding values. The approach in this paper would be useful to develop new functionals and to update the previously developed functionals.
更多查看译文
关键词
density functional,molecules,ktlc,theorem-compliant,long-range,black-box,data-driven
AI 理解论文
溯源树
样例
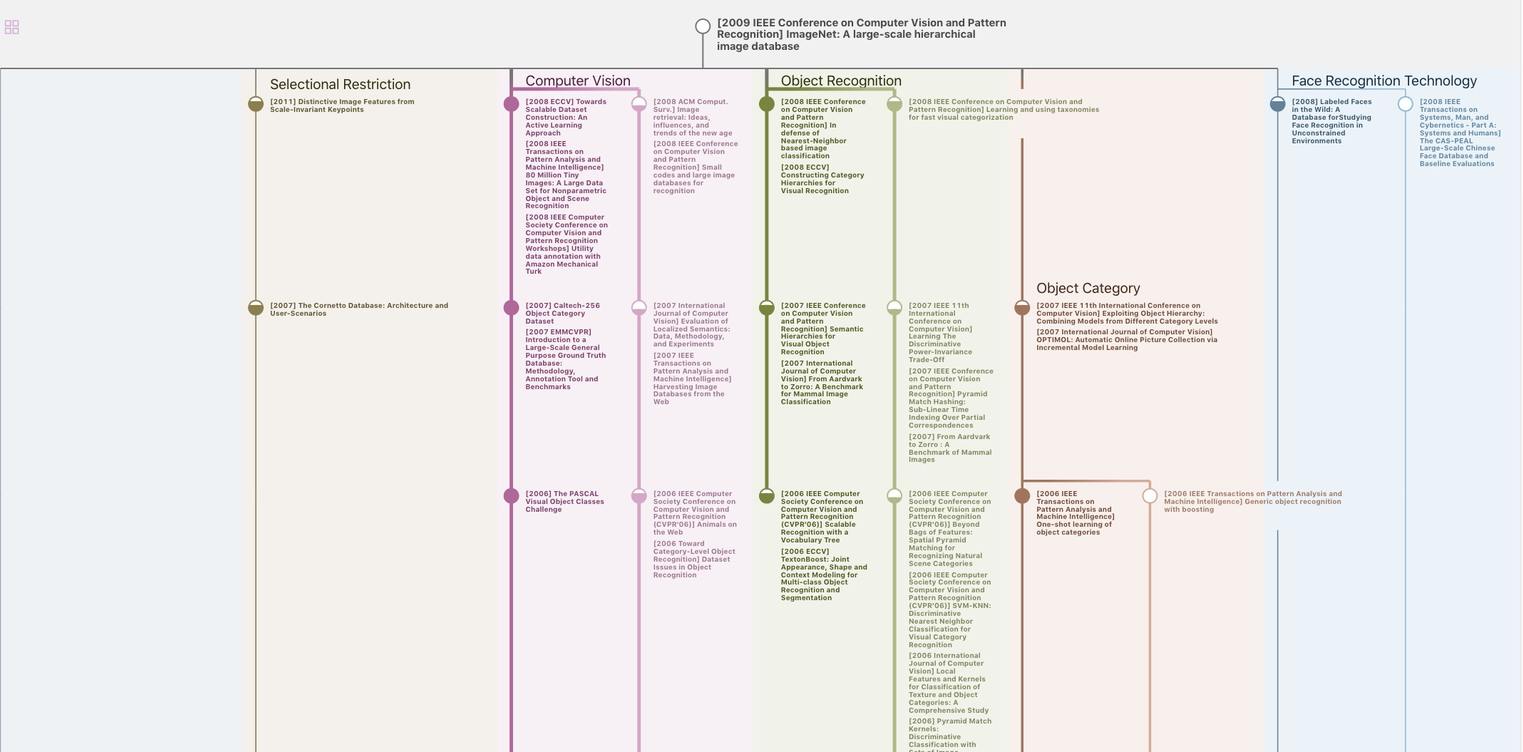
生成溯源树,研究论文发展脉络
Chat Paper
正在生成论文摘要