Computer models in healthcare shed light on the roots of missed care in system design: Nurse workload
JOURNAL OF ADVANCED NURSING(2024)
摘要
This commentary has been inspired by the recent editorial in JAN on missed nursing care and cultures of busyness (Jackson, 2023). We do not rebut any of the content in this excellent overview of the issue of missed care – but offer here another perspective on the concept of the ‘culture of busyness’. We agree that much of missed care is caused by workload issues for nurses (rather than merely ‘forgetting’). Our position is that this workload is not a ‘cultural’ product, but instead a measurable consequence of the design of care systems. Our research, using computer simulations of care delivery processes (created and validated using real-world data), suggests that the roots of nurse workload lie in the design and operational situations nurses find themselves in where the demands on nurses far exceed their capacity to complete the required tasks within their working shifts. Nurse ‘Busyness’ is, in this case, the product of workload levels that drive the missed care problem. Thus, we argue that the way forward in managing and minimizing missed care lies in care-system design and better workload management. In this commentary, we highlight the basic modelling approach used to understand the drivers of missed care and some of the results obtained to date using this quantification approach. In the Canadian context, and arguably other jurisdictions, there is currently no shift-long measure of nurse workload that would reveal the level of missed care that results from the typical patient care work assignment. As the adage goes: ‘if you can't measure it you can't manage it’, and right now our healthcare system is incapable of managing nurses' workloads. This leads to a form of ‘magical thinking’ about the workload problem – somehow nurses will magically be able to complete their care tasks even as staff are reduced due to austerity politics and new technologies add hours of data entry each week to the nurses task list – a lack of attention to nurse workload in job design. The lines of effect are illustrated in Figure 1 which is based on the ‘Better Work – Better Care’ (BWBC) framework (Neumann & Purdy, 2023). While the original framework addresses care quality issues and patient outcomes more broadly – we adjust this figure here to focus on the issue at hand, namely ‘Missed Care’. The BWBC model recognizes that the nature of a nurse's work is caused by work design that can include issues related to the physical layout of the built environment, the nature of the care tasks needed including patient characteristics, operational policies and assignments, patient and material logistics, technologies and equipment in use, and staffing and HR policies. All of these design elements contribute to the demands and experience of a nurse working in a given ‘Care Delivery System’ (Figure 1). The workload will, in turn, have consequences for the working nurse: which include ‘busyness’, fatigue, experience over time and, in the face of prolonged excess workload, problems like burnout and sickness-absence. Finally, whatever the effects of workload, in particular work overload, have on the nurse will impact the care delivered to the patient. In this particular focus missed care will result from this experience of work overload and fatigue and will determine rates of missed care and subsequent outcomes including costs to the healthcare system itself. While this is, of necessity, an abridged account, the BWBC framework points out that negative outcomes like missed care, are the result of design and operational choices made in the design and formation of the healthcare system. Addressing the missed care problem, therefore requires attention to the design of the system. While there are many calls for ‘evidence-based tools’ to support staffing (e.g. nurse–patient ratio) decisions in healthcare, there are few tools that provide objective quantitative data linking system configuration to nurse time demands at work. Our interdisciplinary team has developed an approach using computer-based process (discrete event) simulations to create task-by-task simulations of the care delivery process to examine outcomes such as missed care under a variety of operational scenarios (Qureshi et al., 2019, 2020, 2021a, 2021b; Qureshi, Purdy, et al., 2022; Qureshi, Bookey-Bassett, et al., 2022). In this commentary, we describe this approach very briefly and then share some of the specific results observed from several modelling studies. The model was built using a commercially available discrete event simulation (DES) software and the model-building process could be readily adapted to other settings. We provide a brief description of the model inputs and outputs here for the case of an acute care hospital unit. Inputs to the model include: (1) Floor plan and distances for all locations in the unit, (2) A set of patient care tasks, task frequency, and task duration distributions, per patient – from records or measured in time motion studies if needed), (3) task movement sequences (e.g. to gather supplies from storage before going to a patient), (4) care task priorities and care delivery logics, obtained via focus groups, to specify how nurses decide what to do next. The DES model can handle the complex time and frequency variability of tasks and the resulting shift-long working patterns can be established for hundreds of shifts in minutes. Scenarios of interest, for example, the impacts of patient acuity on workload indicators – with associated changes in certain task frequencies and duration ranges – can be explored. Outputs of the model include a time history of care task execution, time and distance spent walking, the number of tasks ‘in queue’ that the simulant nurse must keep in mind (an indicator of mental workload), time a task spent ‘waiting’ to be performed, direct care time and, central to this commentary, the number of missed care tasks which remain undone at the end of a 12-h shift and the amount of time it would take to complete these tasks. This approach provides a platform in which design choices can be ‘interrogated’ individually or in concert for their impacts on specific outcomes of concern – such as missed care. While the description here is necessarily brief, further details are available in the published works (c.f. Qureshi et al., 2019, 2020, 2021a, 2021b; Qureshi, Purdy, et al., 2022; Qureshi, Bookey-Bassett, et al., 2022). The method has been validated by comparing model runs to specific nurses' work assignments with good results and seems to track their movement patterns well (Qureshi et al., 2021a; Qureshi, Bookey-Bassett, et al., 2022). We now present some examples of the results of this approach in terms of the impacts of changing key work-design parameters on missed care (tasks remaining to be done at the end of a 12-h shift) and missed care time (the time that would be required to complete those tasks based on average task duration data). The examples here are drawn from studies in an acute care hospital setting. This approach can be adapted to other settings as needed. We remind the reader here that the quantified ‘missed care’ from the simulation represents tasks left undone at the end of a simulated 12-h shift. In practice this could be reduced by either rushing tasks, or with overtime care – both are solutions that generate their own problems for cost and quality of care. Figure 2 illustrates time utilization in a med-surg unit based on the institution's own standard task-time data. A 12-h shift without breaks and 12-h shift with breaks led to 2.3 and 2.9 h of missed care delivery time, respectively. This is equivalent to 18.6 and 20.9 missed care tasks per shift on average (Qureshi et al., 2021a). Figure 3 further describes the per cent division of Missed care tasks, examining two care delivery logics. In both cases, the highest per cent of missed care tasks were ‘non-patient care’, ‘evaluation’ and ‘hygiene-related’ tasks. However, for care delivery logic 1, ‘teaching and emotional support’ and ‘other direct nursing care’ were higher than logic 2. This example (Figures 2 and 3) illustrates how both the working situation (decisions to take or skip breaks) and the working logic of nurses can be explored using simulation to understand the impacts of missed care (Qureshi et al., 2021a). Such information can help manage both the quantity and types of care missed under different operational conditions as suggested by Dr. Jackson's (2023) editorial. Using the same field-validated model as above with its specific geography and layout, we extended our analysis to examine the impact of geographic patient bed assignments. Figure 4 illustrates the impact that bed assignment can have on missed care. We found that both the ‘average distance of beds to the nursing station’, as well as the ‘distance between assigned patient beds’ (e.g. clustered vs scattered) affect missed care at the end of a 12-h shift. All other working conditions, such as nurse–patient ratio and acuity levels, were the same for these comparisons (Qureshi et al., 2021b). It would be feasible with this approach to examine the impact of different architectural configurations of key rooms (e.g. for medicine or material supplies, etc.) at the time these layouts are being designed. During the recent pandemic, we were able to examine the impacts of the presence of COVID-19-positive (C+) patients on nurse workloads and potential missed care volumes. This analysis was based on the same medical-surgical hospital unit as the previous examples, as this unit was designated a COVID care unit during the height of the pandemic. In this simulation the 5-patient assignment was adjusted to include 0 to 5 C+ patients; with each patient requiring increased care due to their acuity (increasing task times and frequencies) as well as the required infection prevention and control routines – particularly the donning and doffing of PPE required for each room entry. We quantified substantial increases in missed care driven primarily by the time required for IPAC as illustrated in Figure 5 (Qureshi, Bookey-Bassett, et al., 2022). In this commentary, we have presented several examples that illustrate how computer simulation of the care delivery system can provide quantitative information related to nurses' time utilization and key quality indicators such as missed care. We do not claim ‘generalisability’ of any specific analysis result here – but instead illustrate the linkages between design decisions and missed care response patterns – revealing the drivers of workload related missed care in healthcare systems. These kinds of simulation tools are adaptable and can provide quantitative information on design choices for a wide range of care delivery systems. Any model input can be varied as desired to obtain quantitative analyses of its impact on workload and care quality indicators such as missed care. These evaluation tools can help generate knowledge and data for those making critical decisions affecting nurse workload, but they are not in and of themselves idea or solution generating. They do allow users to explore idea solutions and understand possible consequences their design choices or options may have if implemented. The inclusion of quantitative staff workload indicators, alongside conventional quality and cost indicators, provides a strategic advantage in the long term success of healthcare systems improvement approaches (Neumann & Purdy, 2023) This can help overcome the ‘if you can't measure it, you can't manage it’ problem that exists in many aspects of staff workload management and healthcare system design. Our modelling analyses suggest that missed care is the product of a broad range of specific isolable decisions made in the design and operation of the healthcare system. Efforts to reduce missed care should, therefore, focus on the design of the care system and the task assignments given to working nurses. Simulation tools can help diagnose the problem, by providing quantitative data on operational performance, but healthcare leaders at all levels are responsible for appropriate decision making and action. Ultimately, we argue (Neumann & Purdy, 2023), quantitative evaluation of nurse workload and working environments is an essential element of designing and managing healthcare systems at all levels, and that better work for nurses means better care for patients. All authors have contributed to the formulation and writing of this article. The research presented here has been supported in part by the Natural Sciences and Engineering Research Council of Canada (NSERC RGPIN 2018-05956 and grant no. 341664) and by the Canadian Institute for Health Research (CIHR Operating grant: COVID-19 – 440186). This research was funded by the NSERC (Natural Sciences and Engineering Research Council of Canada) Discovery Grants program (RGPIN 2018 no. 341664); and by the Canadian Institutes for Health Research (CIHR), COVID-19 Rapid Grant program (440186). The authors have no conflict of interest to report. Data presented in this article are from models that contain instituional data which is not freely avilable. Please contact the authors with queries.
更多查看译文
关键词
better work better care,care quality,ergonomics,healthcare engineering,human factors,system simulation,workload measurement
AI 理解论文
溯源树
样例
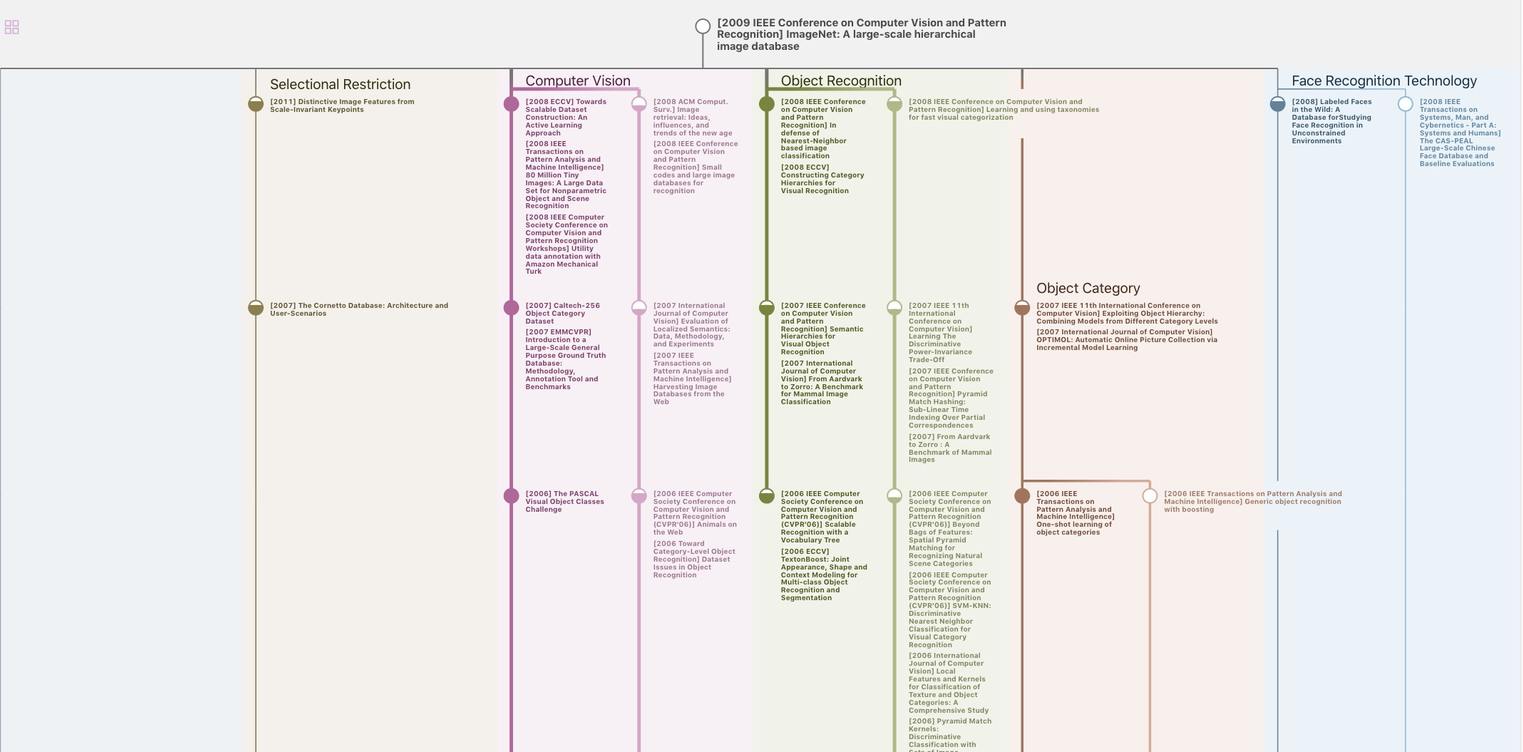
生成溯源树,研究论文发展脉络
Chat Paper
正在生成论文摘要