Foundation Models for Quantitative Biomarker Discovery in Cancer Imaging
medRxiv : the preprint server for health sciences(2023)
摘要
Foundation models represent a recent paradigm shift in deep learning, where a single large-scale model trained on vast amounts of data can serve as the foundation for various downstream tasks. Foundation models are generally trained using self-supervised learning and excel in reducing the demand for training samples in downstream applications. This is especially important in medicine, where large labeled datasets are often scarce. Here, we developed a foundation model for imaging biomarker discovery by training a convolutional encoder through self-supervised learning using a comprehensive dataset of 11,467 radiographic lesions. The foundation model was evaluated in distinct and clinically relevant applications of imaging-based biomarkers. We found that they facilitated better and more efficient learning of imaging biomarkers and yielded task-specific models that significantly outperformed their conventional supervised counterparts on downstream tasks. The performance gain was most prominent when training dataset sizes were very limited. Furthermore, foundation models were more stable to input and inter-reader variations and showed stronger associations with underlying biology. Our results demonstrate the tremendous potential of foundation models in discovering novel imaging biomarkers that may extend to other clinical use cases and can accelerate the widespread translation of imaging biomarkers into clinical settings.
### Competing Interest Statement
The authors have declared no competing interest.
### Funding Statement
The authors acknowledge financial support from NIH (H.J.W.L.A: NIH-USA U24CA194354, NIH-USA U01CA190234, NIH-USA U01CA209414, and NIH-USA R35CA22052), and the European Union - European Research Council (H.J.W.L.A: 866504).
### Author Declarations
I confirm all relevant ethical guidelines have been followed, and any necessary IRB and/or ethics committee approvals have been obtained.
Yes
The details of the IRB/oversight body that provided approval or exemption for the research described are given below:
HarvardRt, the singular non-public dataset used in this study was collected under an IRB-approved retrospective protocol with a waiver of consent (Dana-Farber/Harvard Cancer Center protocol 11-286).
I confirm that all necessary patient/participant consent has been obtained and the appropriate institutional forms have been archived, and that any patient/participant/sample identifiers included were not known to anyone (e.g., hospital staff, patients or participants themselves) outside the research group so cannot be used to identify individuals.
Yes
I understand that all clinical trials and any other prospective interventional studies must be registered with an ICMJE-approved registry, such as ClinicalTrials.gov. I confirm that any such study reported in the manuscript has been registered and the trial registration ID is provided (note: if posting a prospective study registered retrospectively, please provide a statement in the trial ID field explaining why the study was not registered in advance).
Yes
I have followed all appropriate research reporting guidelines, such as any relevant EQUATOR Network research reporting checklist(s) and other pertinent material, if applicable.
Yes
The majority of the datasets utilized in this study are openly accessible for both training and validation purposes and can be obtained from the following sources: i) DeepLesion [], used both for our pre-training and use-case 1 ii) LUNA16 [] used for developing our diagnostic image biomarker iii) LUNG1 [wiki.cancerimagingarchive.net/display/Public/NSCLC-Radiomics] and iv) RADIO [] used for the validation of our prognostic image biomarker model. The training dataset for our prognostic biomarker model, HarvardRT, is internal and unavailable to the public. HarvardRT was collected under an IRB-approved retrospective protocol with a waiver of consent (Dana-Farber/Harvard Cancer Center protocol 11-286). As the trained foundational model is public, all the results can be reproduced using the accessible test datasets.
更多查看译文
关键词
quantitative biomarker discovery,foundation,imaging,cancer
AI 理解论文
溯源树
样例
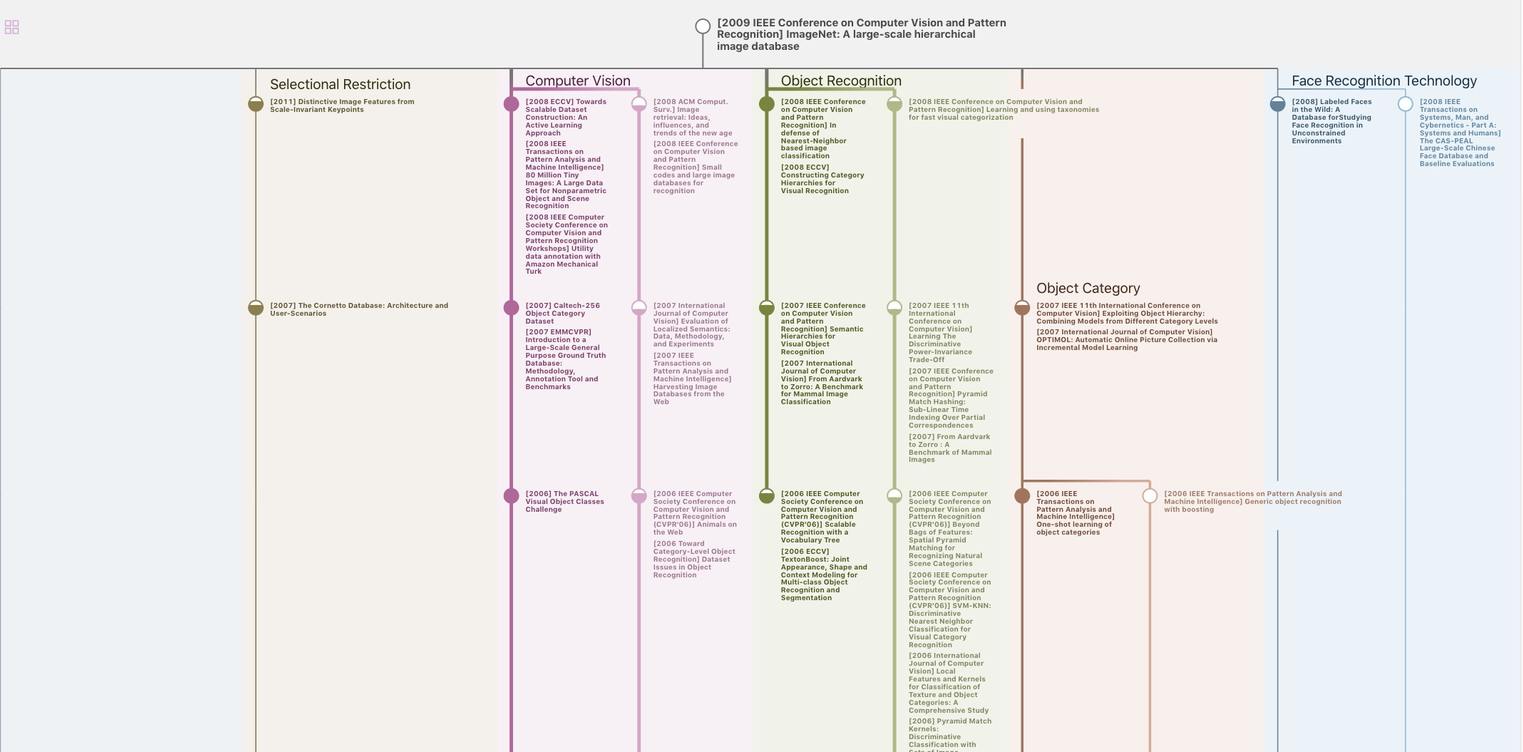
生成溯源树,研究论文发展脉络
Chat Paper
正在生成论文摘要