Assessing the Performance of Satellite-Based Models for Crop Yield Estimation in the Canadian Prairies
Canadian Journal of Remote Sensing(2023)
摘要
Timely monitoring of crop production using a remote sensing-based approach offers promise toward enhancing food security. Statistical models developed using satellite data typically employ a single vegetation index from a single sensor for yield estimation. With the increasing availability of satellite datasets, there is now an opportunity to investigate the potential of available vegetation indices from different sensors in estimating yields. The key objective of this study was to develop a best-performing satellite-based yield model for the Canadian Prairies for wheat, barley, and canola, trained using municipality-level data from 2009 to 2019. We tested the statistical performance of models built using (a) indices from different sensors (Landsat and Sentinel-2), (b) indices sensitive to different yield properties, and (c) single versus multiple vegetation indices. Results showed Landsat-NDWI as the best performing single-index across all indices and sensors for each crop. Sentinel-2-EVI performed best for wheat and canola and Sentinel-2-SR for barley; but these models were built using only 4 years of data. We found that best-performing single-index models recorded similar predictive accuracy as multi-index models during model validation. The results from this work suggest that satellite-based yield estimation can be improved by selecting the right index related to different crop properties.
更多查看译文
关键词
crop yield estimation,canadian prairies,satellite-based
AI 理解论文
溯源树
样例
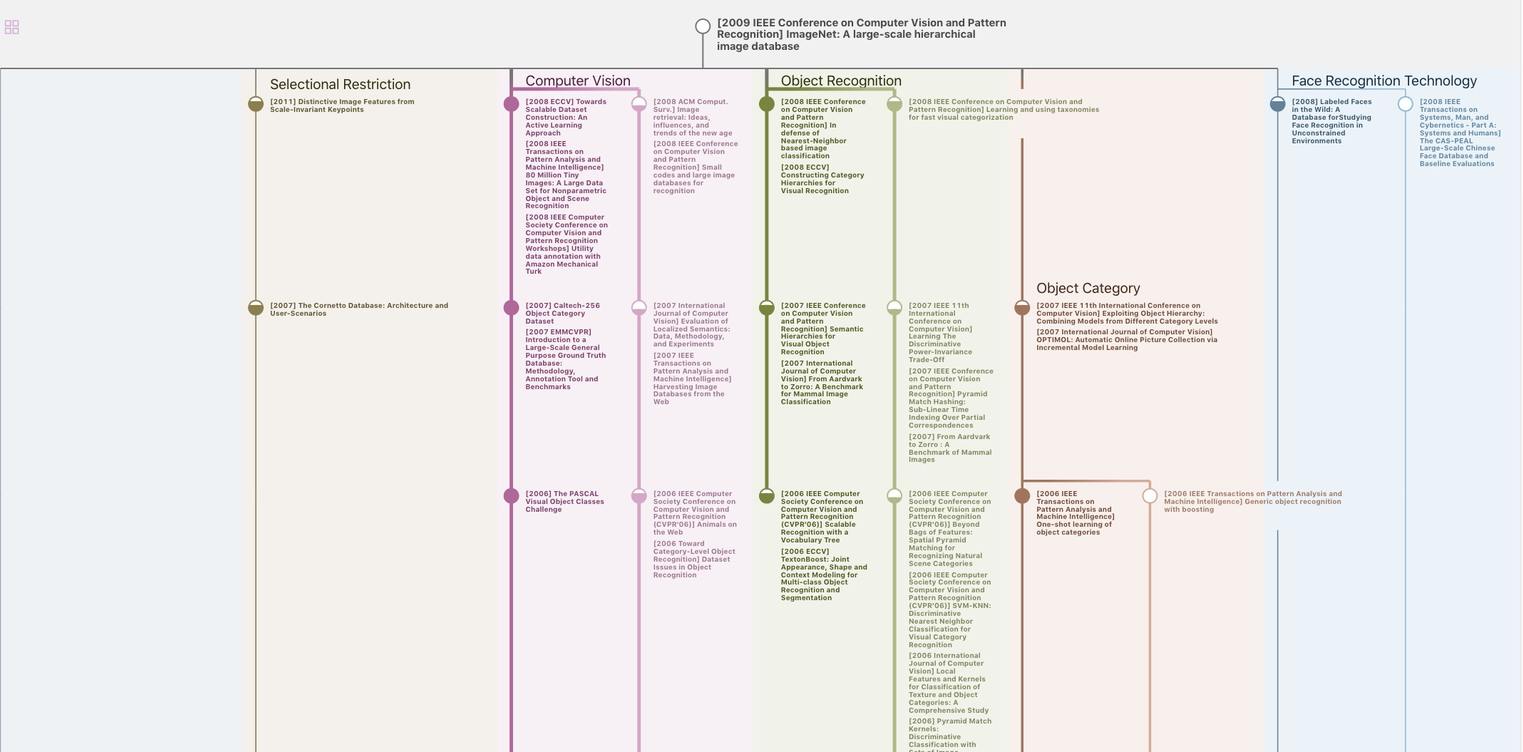
生成溯源树,研究论文发展脉络
Chat Paper
正在生成论文摘要