Optimizing Multi-Modal Electromagnetic Design Problems Using Quantum Particle Swarm Optimization With Differential Evolution
IEEE Access(2023)
摘要
Many versatile and promising swarm intelligence evolutionary algorithms are being developed to solve engineering optimization problems. Although evolutionary algorithms have been implemented in various optimization fields, there is still potential for enhancement in the domain of complex, electromagnetic, and multimodal objective problems. To effectively address the shortcomings and slow convergence speed observed in both smart quantum particle swarm optimization (QPSO) and differential evolution (DE), a hybrid strategy is proposed. In the proposed QPSODE, apart from the smart strategy of QPSO for improving the exploration as a whole, more additional features such as non-linear adaptive control parameter, the partition of the swarm to apply smart and gaussian mutation mechanism, crossover and selection of best particle using Boltzmann strategy to avoid premature convergence are introduced. Consequently, applying the new design algorithm to several benchmark-constrained, mostly non-convex, and superconducting magnetic energy storage (SMES) electromagnetic problems shows a marked performance improvement. The performances of the QPSODE is compared with those of many other widely recognized population-based swarm intelligence optimizers. Experimental results and statistical analysis using Friedman test show that the search accuracy and the convergence of the hybrid QPSODE strategy are advantageous over other optimization approaches.
更多查看译文
关键词
Smart particle,hybridization,QPSO,DE,Boltzmann selection strategy,energy storage device (SMES)
AI 理解论文
溯源树
样例
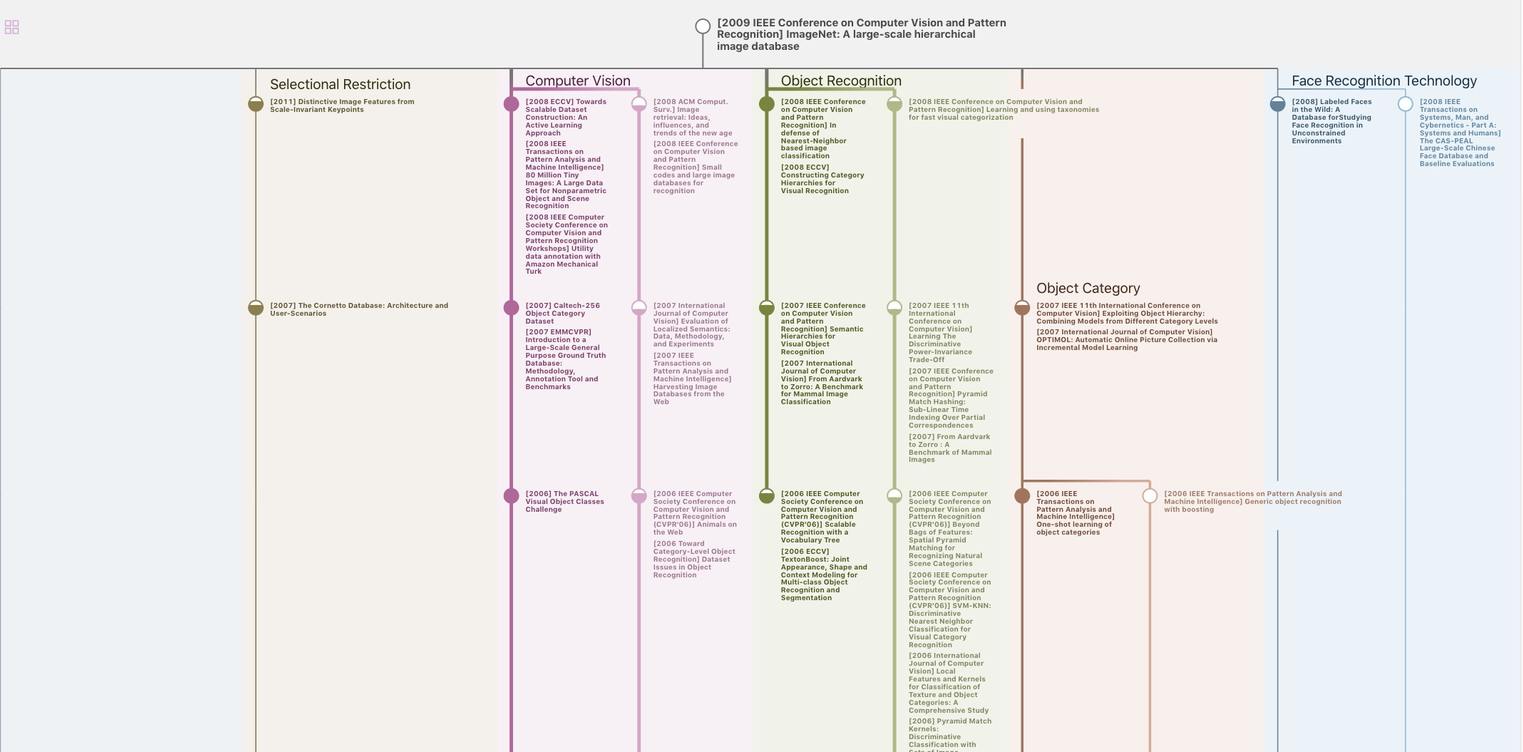
生成溯源树,研究论文发展脉络
Chat Paper
正在生成论文摘要