Multi-Channel Bin-Wise Speech Separation Combining Time-Frequency Masking and Beamforming
IEEE Access(2023)
摘要
This paper presents a novel Blind Source Separation method that can handle convolutive mixtures that may be underdetermined. Our method combines TF masking and beamforming and exploits the source signals sparsity in the Time-Frequency (TF) domain. Remarkable performance can be achieved by TF masking-based methods, even in the underdetermined case, although they tend to generate unwanted artifacts at the level of the separated signals. Besides, beamforming techniques can achieve satisfactory performance only in the overdetermined and determined cases without distorting the estimated signals. By combining these two approaches, we can leverage their respective strengths. Firstly, we exploit the source signals sparsity in the TF domain to estimate probabilistic “bin-wise” masks by modeling the frequency observation vectors with a complex Gaussian Mixture Model and using an EM algorithm. However, due to the sensitivity of the EM algorithm to initialization, we propose properly selecting the initial values of the model parameters using Hermitian angles between the frequency observation vectors and a reference vector. Then, we utilize the estimated TF masks to estimate the Relative Transfer Functions of each source. Finally, we propose a new technique to obtain an estimate of the spatial images of the separated sources, which can be regarded as an underdetermined extension of the Linearly Constrained Minimum Power beamformer. Good performance was observed in test results for our method, both in the determined and underdetermined cases, compared to various existing methods with similar working hypotheses.
更多查看译文
关键词
separation,speech,multi-channel,bin-wise,time-frequency
AI 理解论文
溯源树
样例
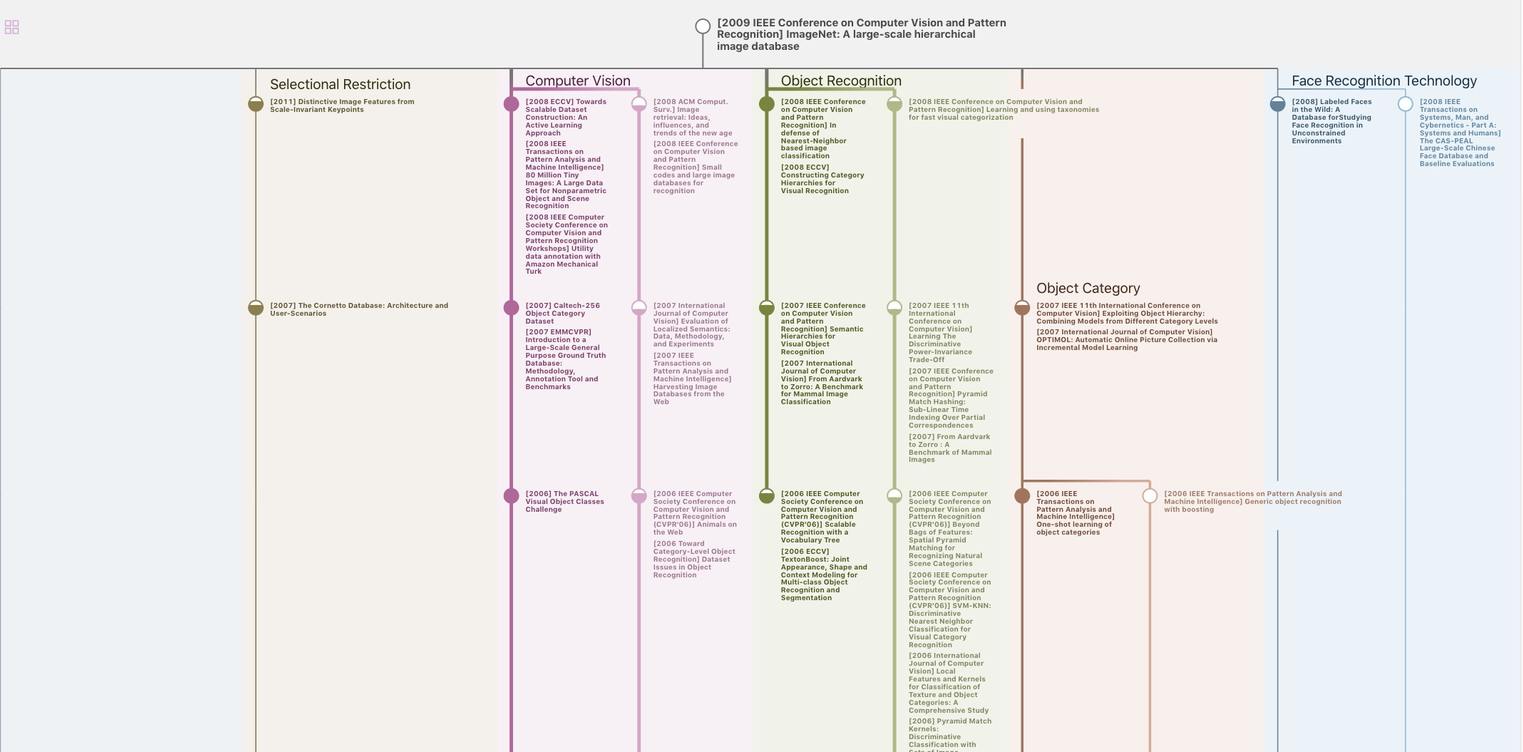
生成溯源树,研究论文发展脉络
Chat Paper
正在生成论文摘要