Poster Session: Bayesian inference and adaptation in neural responses.
Journal of vision(2023)
摘要
Many of the perceptual changes induced by adaptation can be captured in a Bayesian framework, in which adaptation renormalizes neural responses to weak or strong stimuli, consistent with correcting the likelihood to match the prior (Emery and Webster VSS 2020). We explored what this model implies about Bayesian estimation at the level of an individual neuron or channel. The prior corresponds to what the neuron expects to see, but the adaptation causes responses to match what it expects. If the decoder does not "know" the neuron's adaptation state (Series et al 2009), these adjustments effectively flatten priors for dimensions the neuron adapts to, preserving expectations only for information that it does not adapt to, such as how signals deviate from the predicted value. Bayesian-like estimates of these deviations can be computed within the neuron because the shape of the prior is instantiated in the neuron's contrast response function (CRF). For example, for a Gaussian prior the corresponding CRF is a cumulative Gaussian. Steeper CRFs bias responses more toward the mean. The biases approximate but do not equal the Bayesian posterior. If, like adaptation, the decoding does not account for this approximation, then the interpreted stimulus value is distorted relative to the Bayes estimate. This may underlie the distortions evident in some perceptual dimensions, such as nonlinearities in perceived contrast.
更多查看译文
关键词
bayesian inference,adaptation,responses,poster
AI 理解论文
溯源树
样例
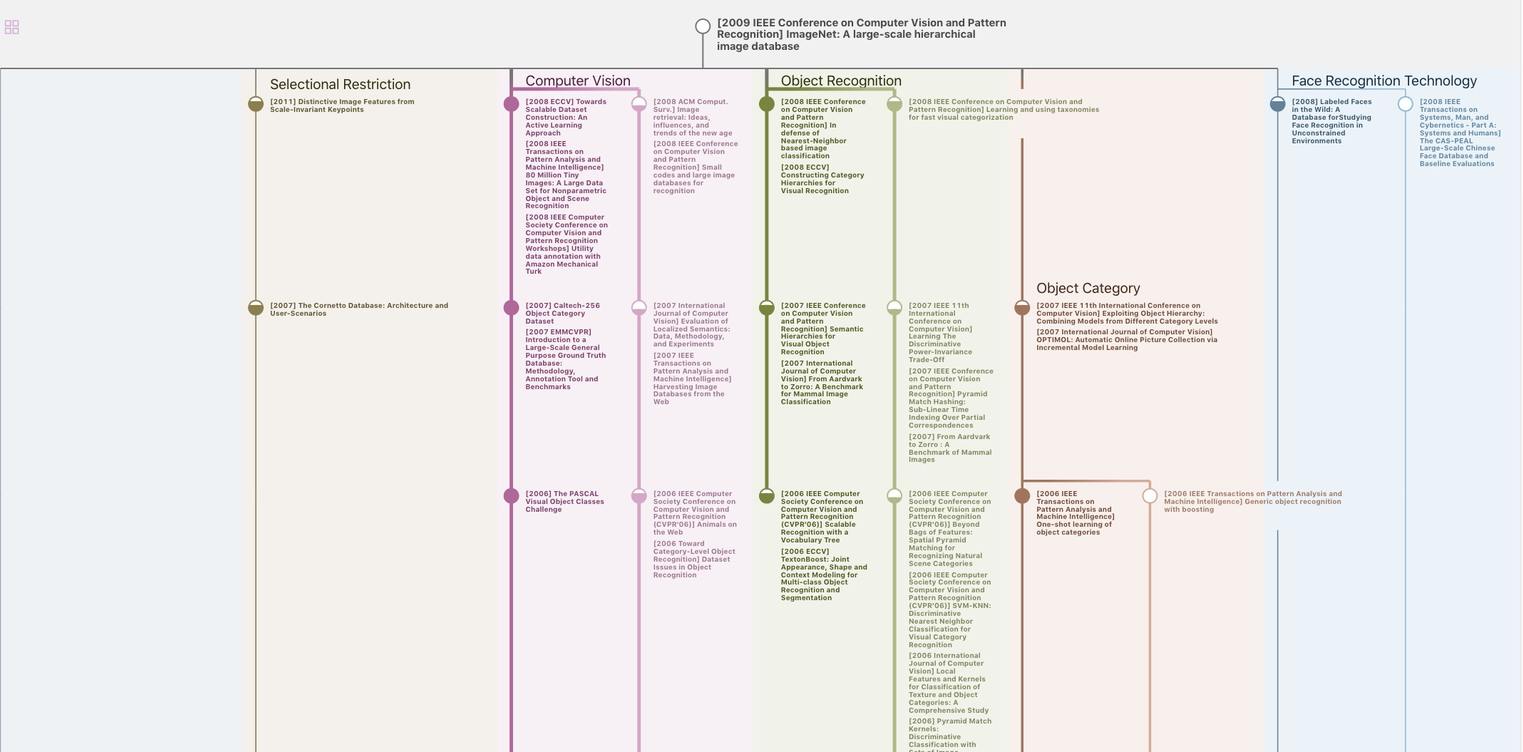
生成溯源树,研究论文发展脉络
Chat Paper
正在生成论文摘要