Factors Influencing the Referral of Patients with Prediabetes to a Diabetes Prevention Program in the Bronx, New York.
Preventing Chronic Disease(2023)
摘要
INTRODUCTION:Disparate engagement in the Diabetes Prevention Program (DPP) may occur as early as the point of referral for certain subgroups, including Black and Hispanic men. We aimed to determine patient demographic and provider characteristics associated with referrals to a health system DPP in the Bronx, New York. METHODS:Patient and health system characteristics for DPP-eligible patients seen in primary care between July 1, 2015, and December 31, 2017, were obtained through the electronic health record. Generalized mixed-effects modeling was used to test the association between referral rate and clinical and sociodemographic variables. RESULTS:Of 26,727 eligible patients, 66% were female, 46% were Hispanic, and 39% were non-Hispanic Black. Only 10% (n = 2,785) of eligible patients were referred to DPP. In the adjusted analyses, lower odds of referral were observed for men versus women (OR = 0.60; 95% CI, 0.52-0.66), for non-Hispanic White versus Hispanic patients (OR = 0.53; 95% CI, 0.40-0.71), and for uninsured patients versus Medicaid patients (OR = 0.66; 95% CI, 0.54-0.80). The odds were higher for patients in the highest versus lowest hemoglobin A1c (OR = 2.49; 95% CI, 2.27-2.72) category; for those in the highest versus lowest body mass index categories (OR = 1.61; 95% CI, 1.45-1.79); for middle-aged patients (aged 45-64 y) versus those aged 18-26 y (OR =1.63; 95% CI, 1.33-2.00); and for patients being seen by a family versus an internal medicine physician (OR = 1.65; 95% CI, 1.22-2.22). CONCLUSION:We identified under-referral for men and highlighted other patient and health system factors associated with referral rates. Interventions to address bias in referrals and increase referrals for men at high risk for diabetes, not typically represented in DPP, are recommended.
更多查看译文
关键词
Chronic Disease Management
AI 理解论文
溯源树
样例
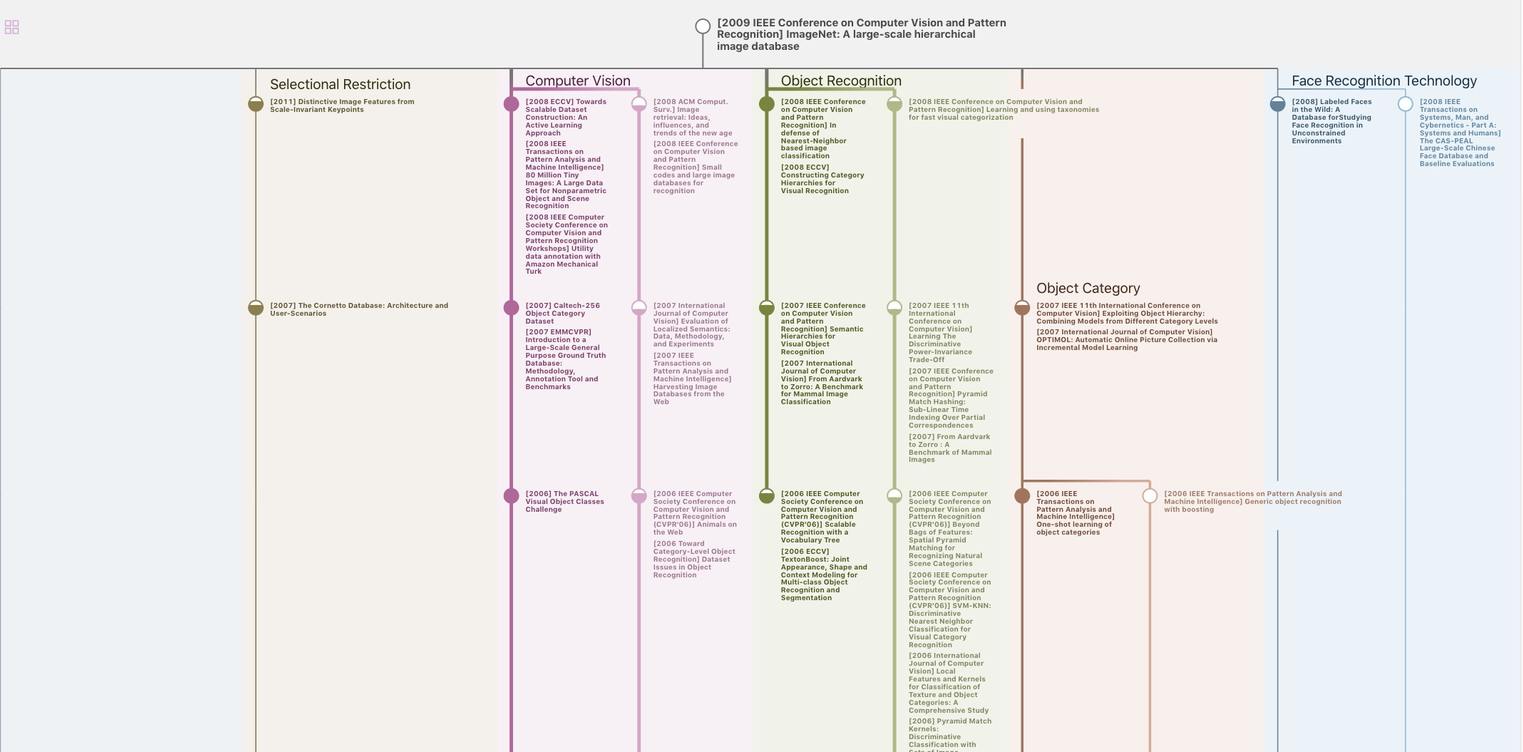
生成溯源树,研究论文发展脉络
Chat Paper
正在生成论文摘要