Deep Broad Learning for Emotion Classification in Textual Conversations
TSINGHUA SCIENCE AND TECHNOLOGY(2024)
摘要
Emotion classification in textual conversations focuses on classifying the emotion of each utterance from textual conversations. It is becoming one of the most important tasks for natural language processing in recent years. However, it is a challenging task for machines to conduct emotion classification in textual conversations because emotions rely heavily on textual context. To address the challenge, we propose a method to classify emotion in textual conversations, by integrating the advantages of deep learning and broad learning, namely DBL. It aims to provide a more effective solution to capture local contextual information (i.e., utterance-level) in an utterance, as well as global contextual information (i.e., speaker-level) in a conversation, based on Convolutional Neural Network (CNN), Bidirectional Long Short-Term Memory (Bi-LSTM), and broad learning. Extensive experiments have been conducted on three public textual conversation datasets, which show that the context in both utterance-level and speaker-level is consistently beneficial to the performance of emotion classification. In addition, the results show that our proposed method outperforms the baseline methods on most of the testing datasets in weighted-average F1.
更多查看译文
关键词
emotion classification,textual conversation,Convolutional Neural Network (CNN),Bidirectional Long Short-Term Memory (Bi-LSTM),broad learning
AI 理解论文
溯源树
样例
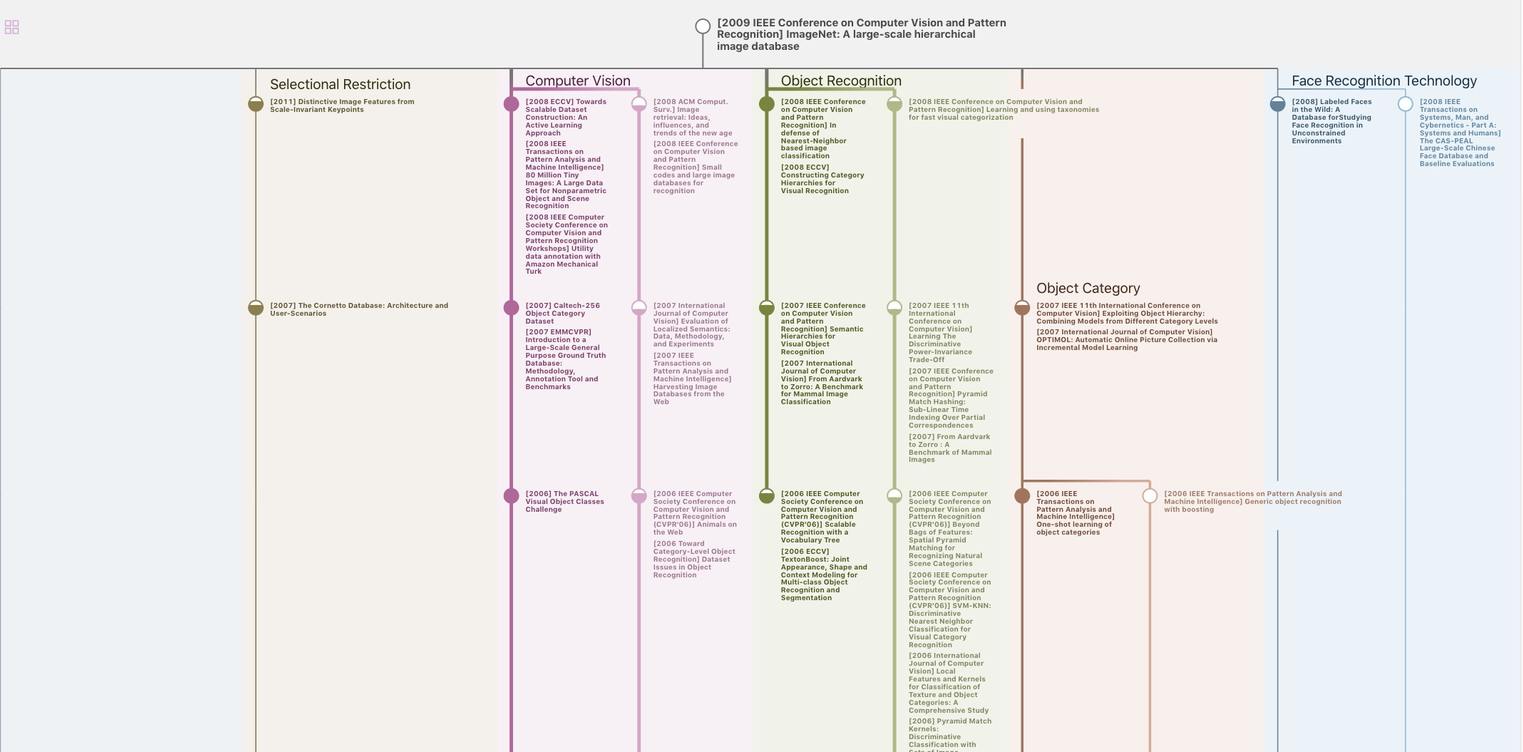
生成溯源树,研究论文发展脉络
Chat Paper
正在生成论文摘要