Deep Reinforcement Learning for NextG Radio Access Network Slicing With Spectrum Coexistence
IEEE Networking Letters(2023)
摘要
Reinforcement learning (RL) is applied for dynamic admission control and resource allocation in NextG radio access network slicing. When sharing the spectrum with an incumbent user (that dynamically occupies frequency-time blocks), communication and computational resources are allocated to slicing requests, each with priority (weight), throughput, latency, and computational requirements. RL maximizes the total weight of granted requests over time beyond myopic, greedy, random, and first come, first served solutions. As the state-action space grows, Deep Q-network effectively admits requests and allocates resources as a low-complexity solution that is robust to sensing errors in detecting the incumbent user activity.
更多查看译文
关键词
NextG,network slicing,reinforcement learning,admission control,resource allocation,spectrum sharing
AI 理解论文
溯源树
样例
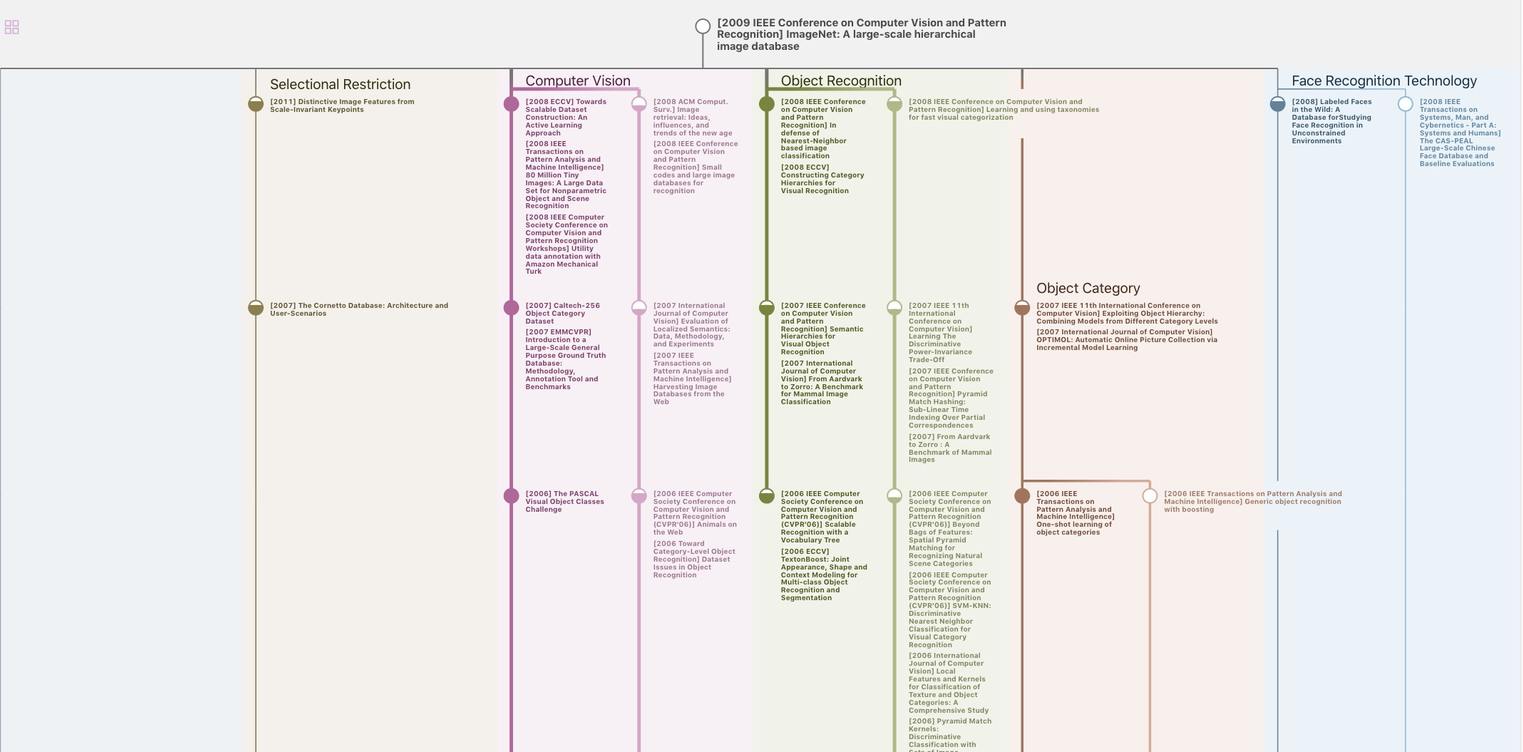
生成溯源树,研究论文发展脉络
Chat Paper
正在生成论文摘要