Future Perspectives of Deep Learning in Laparoscopic Tool Detection, Classification, and Segmentation: A Systematic Review
2023 IEEE 11th International Conference on Serious Games and Applications for Health (SeGAH)(2023)
摘要
Background—Classification, detection, and segmentation of minimally invasive instruments is an essential component for robotic-assisted surgeries and surgical skill assessments. Methods—Cochrane Library, PubMed, ScienceDirect, and IEEE Xplore databases were searched from January 2018 to May 2022. Selected studies evaluated deep learning (DL) models for image and video analysis of laparoscopic surgery. Comparisons were made of the studies' characteristics such as the dataset source, type of laparoscopic operation, number of images/videos, and types of neural networks (NN) used. Results—22 out of 152 studies identified met the selection criteria. The application with the greatest number of studies was instrument detection (59.1%) and the second was instrument segmentation (40.9%). The most tested procedure was cholecystectomy (72.73%). Conclusions—Although CNN-based algorithms outperform other methods in instrument detection and many have been proposed, there are still challenging conditions where numerous difficulties arise. U-Nets are the dominant force in the field for segmentation, but other models such as Mask R-CNN follow close behind with comparable results. Deep learning holds immense potential in laparoscopic surgery and many improvements are expected as soon as data quality improves.
更多查看译文
关键词
Artificial Intelligence, Deep Learning, Computer Vision, Laparoscopy tools
AI 理解论文
溯源树
样例
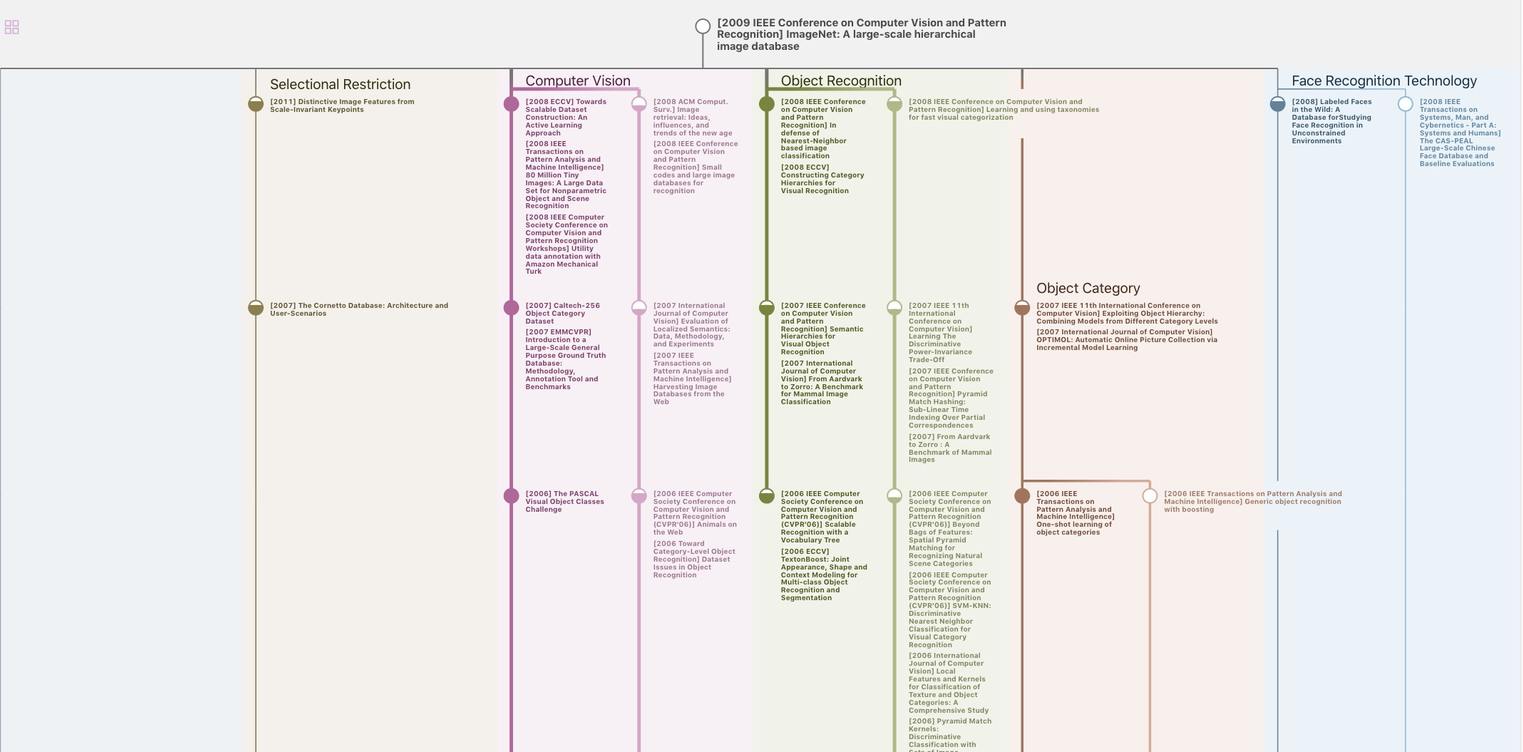
生成溯源树,研究论文发展脉络
Chat Paper
正在生成论文摘要