Radar based Fall Detection with Imbalance Data Handling and Data Augmentation
2023 IEEE Sensors Applications Symposium (SAS)(2023)
摘要
Radar-based fall detection is helping seniors to live independently. In this paper, a new method for fall detection from human activities is proposed using data augmentation and class imbalance handling. Data collected from a radar signal is processed and a time series is obtained by aggregating the individual time series in the fast-time of the radar returns. This time series is used as input to several classifiers to distinguish fall from non-fall activities. To this end, we augment the radar time series data using a Mu-Sigma method. To handle the imbalanced data, we apply the synthetic minority over-sampling technique and class weighting strategy. A comprehensive study is performed to build a supervised learning method with or without data augmentation and imbalanced data handling. The performance of the proposed method is compared with some of the other existing methods using different classifiers including k-nearest neighbors, decision trees, naive Bayes, support vector machine and multi-layer perceptron. The results demonstrate that the proposed fall detection method outperforms the other methods in terms of providing higher accuracy, precision, sensitivity and specificity values.
更多查看译文
关键词
Smart home care,pervasive healthcare,fall detection,ultra-wide band radar
AI 理解论文
溯源树
样例
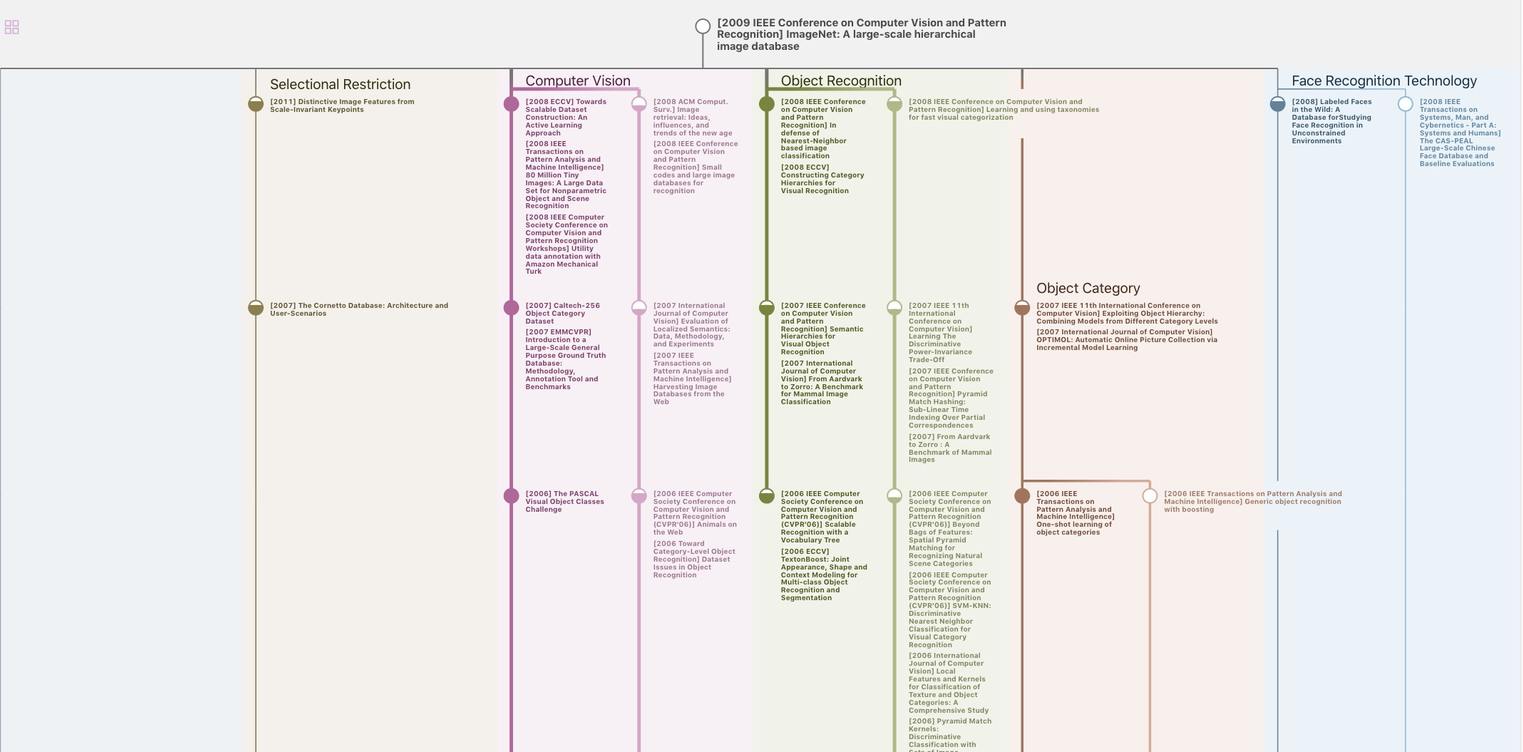
生成溯源树,研究论文发展脉络
Chat Paper
正在生成论文摘要