Interpretation of SNP combination effects on schizophrenia etiology based on stepwise deep learning with multi-precision data
Briefings in functional genomics(2023)
摘要
Schizophrenia genome-wide association studies (GWAS) have reported many genomic risk loci, but it is unclear how they affect schizophrenia susceptibility through interactions of multiple SNPs. We propose a stepwise deep learning technique with multi-precision data (SLEM) to explore the SNP combination effects on schizophrenia through intermediate molecular and cellular functions. The SLEM technique utilizes two levels of precision data for learning. It constructs initial backbone networks with more precise but small amount of multilevel assay data. Then, it learns strengths of intermediate interactions with the less precise but massive amount of GWAS data. The learned networks facilitate identifying effective SNP interactions from the intractably large space of all possible SNP combinations. We have shown that the extracted SNP combinations show higher accuracy than any single SNPs and preserve the accuracy in an independent dataset. The learned networks also provide interpretations of molecular and cellular interactions of SNP combinations toward schizophrenia etiology.
更多查看译文
关键词
SNP combination,GWAS,interpretable machine learning,schizophrenia
AI 理解论文
溯源树
样例
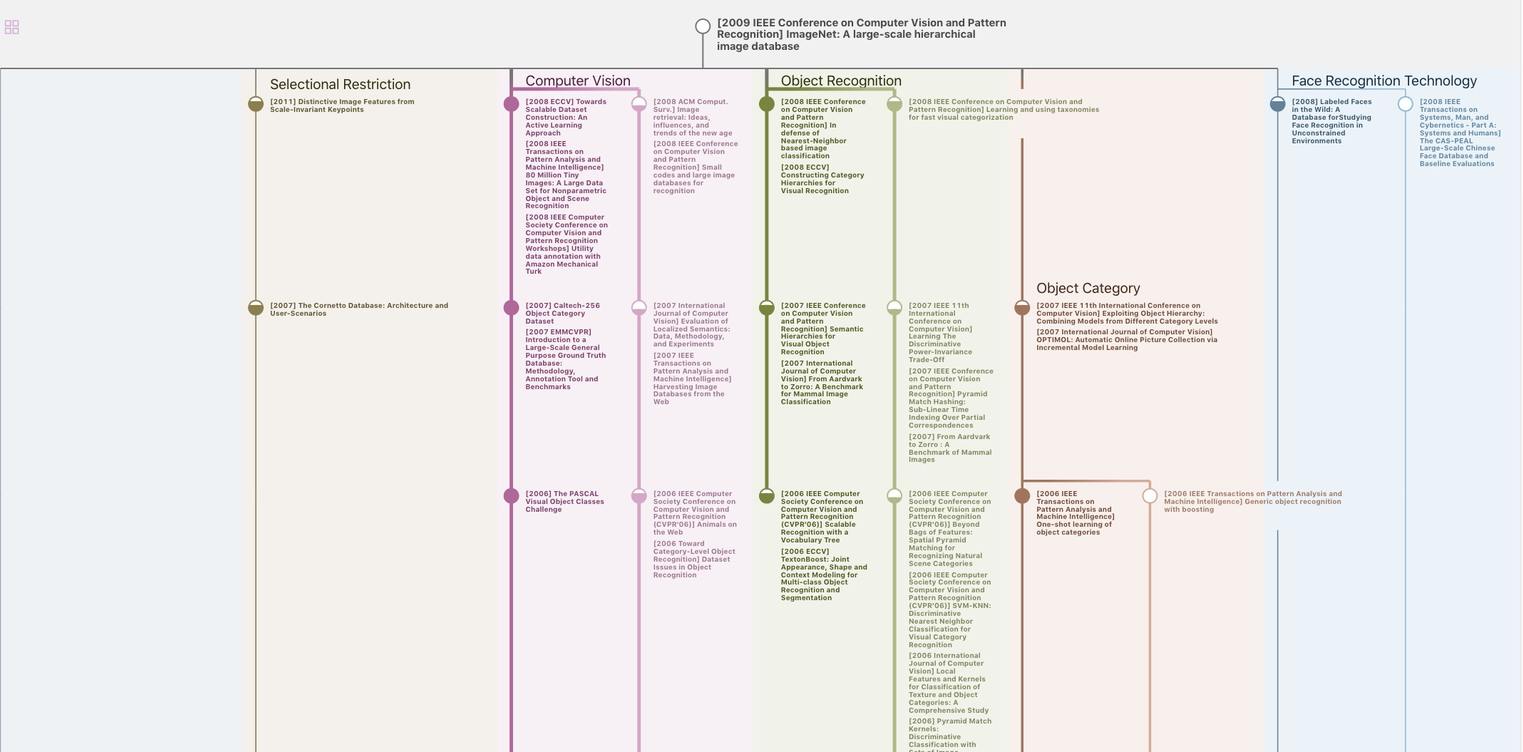
生成溯源树,研究论文发展脉络
Chat Paper
正在生成论文摘要