NRD-Net: a noise-resistant distillation network for accurate diagnosis of prostate cancer with bi-parametric MRI images
Multimedia Tools and Applications(2024)
摘要
In recent years, deep learning-based methods have been extensively developed to diagnose Prostate Cancer (PCa) with bi-parametric Magnetic Resonance Imaging (bpMRI) images. Due to the vague characteristic of PCa bpMRI images, the spacial lesion annotations and grading annotations inevitably contain some noises, which has a serious impact on the performance of deep learning models for the diagnosis of PCa. Furthermore, the similar background features of PCa bpMRI image also disturb the prediction performance of deep learning models. In this paper, we propose a two-branch Noise-Resistant Distillation Network (NRD-Net) for the accurate diagnosis of PCa with bpMRI images. Firstly, the influence of irrelevant background on the classification can be reduced by segmenting the labeled constrained classification response maps. Then a novel confidence-based binarization segmentation scheme and a multi-branch online distillation classification scheme are proposed to reduce the spatial and grading noises simultaneously. Extensive experiments are conducted on a private dataset and the public PROSTATEx-2 dataset. For the private dataset, the proposed network obtains the best performance for GG prediction, achieving a mean quadratic weighted Kappa of 0.5115 and a mean positive predictive value (PPV) of 0.9506. For the public dataset, the proposed method achieves state-of-the-art results of 0.5058 Kappa and 0.9473 PPV.
更多查看译文
关键词
Prostate cancer diagnosis,bpMRI images,Online distillation,Dual-branch network
AI 理解论文
溯源树
样例
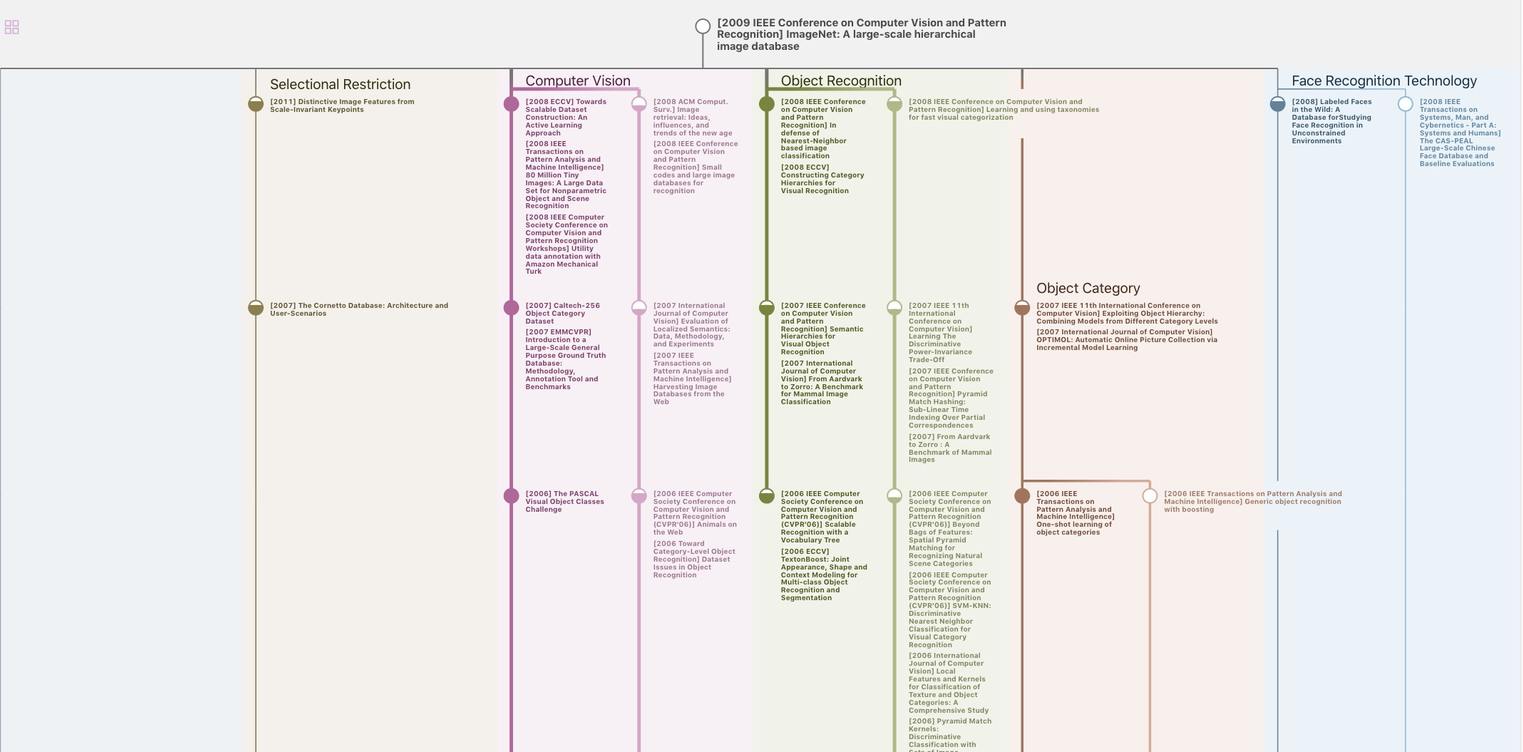
生成溯源树,研究论文发展脉络
Chat Paper
正在生成论文摘要