Accurate remaining useful life estimation of lithium-ion batteries in electric vehicles based on a measurable feature-based approach with explainable AI
The Journal of Supercomputing(2024)
摘要
Electric Vehicles (EVs) become increasingly prevalent, accurately estimating Lithium-ion Batteries (LIBs) Remaining Useful Life (RUL) is crucial for ensuring safety and avoiding operational risks beyond their service life threshold. However, directly measuring battery capacity during EV operation is challenging. In this paper, we propose a novel approach that leverages measurable features based on the discharge time and battery temperature to estimate RUL. Our framework relies on a novel feature extraction strategy that accurately characterizes the battery, leading to improved RUL predictions. Multiple machine learning algorithms are employed and evaluated. Our experimental results demonstrate that the proposed method accurately estimates capacity with minimal hyperparameter tuning. The R^2 scores across various battery numbers indicate strong predictive performance for models like XGBoost, RF, AdaBoost, and others, with improvement percentages ranging from 85
更多查看译文
关键词
Remaining useful life,Lithium-ion batteries,Discharge time,Battery degradation,Learning algorithms
AI 理解论文
溯源树
样例
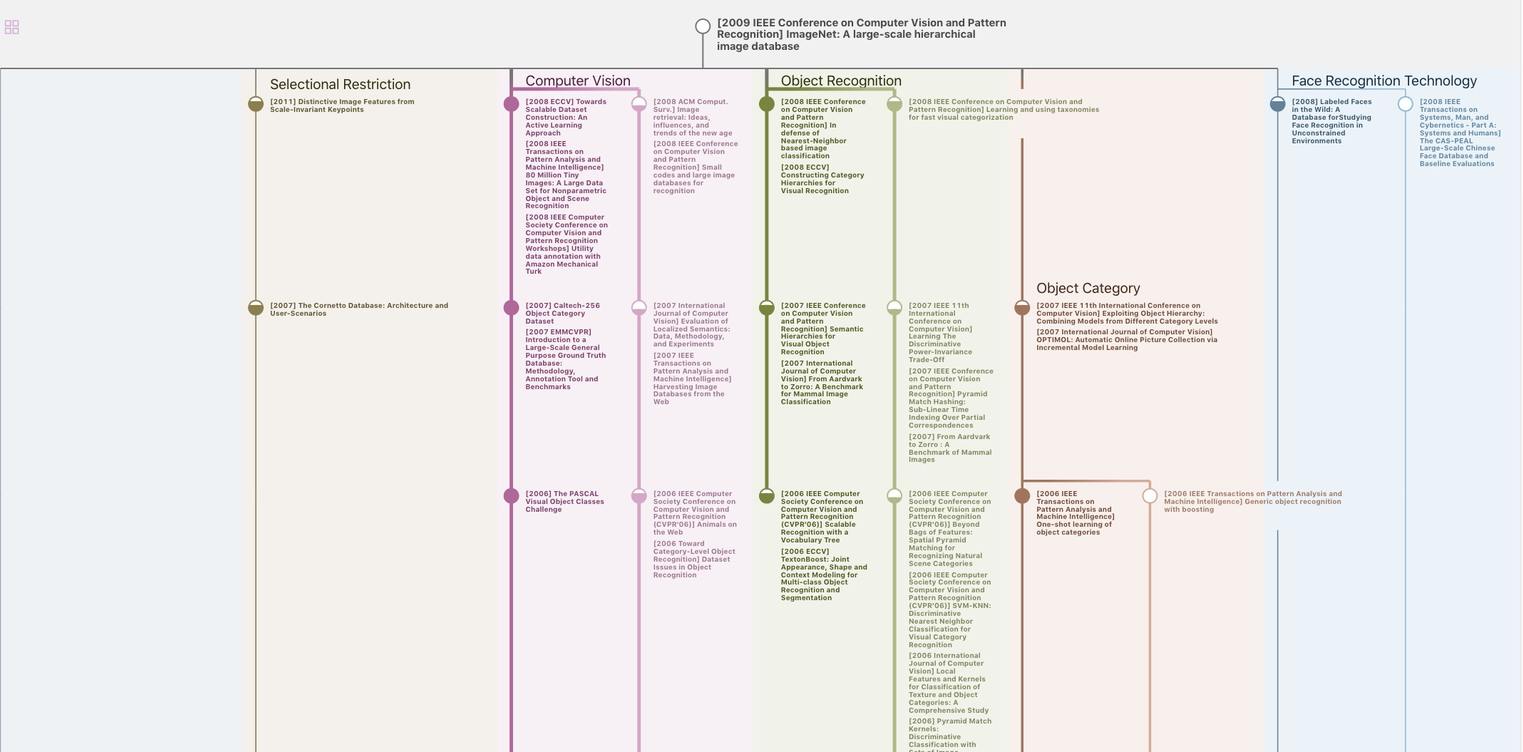
生成溯源树,研究论文发展脉络
Chat Paper
正在生成论文摘要